While La Tribune recently reported that French start-ups in the Agtech and Foodtech sector – meaning digital technologies applied to agriculture and agri-food – had raised no less than 560 million euros over the year 2020, putting France in first place in Europe and fifth place in the world in terms of investments, we now learn that the French government, through its Minister of Agriculture Julien Denormandie and its Secretary of State for Digital Transition Cédric O, is launching a vast plan of more than €200 million to support Agtech and Foodtech companies: French AgriTech.
For its promoters, digital technology in agriculture is presented as both a revolution and a necessity. Big Data, Connected Objects, Robotics, Decision Support Tools, Block Chain, Artificial Intelligence, 5G … – these technologies and buzzwords that are supposed to respond to all the challenges facing agriculture are increasingly present in the mouths of the players who gravitate in and around the agricultural ecosystem, even if it is not certain that these players have really understood their scope. I wanted to show these digital tools in a different way…
This article is very different from those I have written in the past. Here, no overly technical subject or long interviews with professionals in the sector. This work is the result of numerous readings of scientific articles in the humanities and social sciences on digital agriculture (the entire scientific bibliography is available at the bottom of the article), supplemented by personal reflections. As a discipline that is still too little known and followed by technologists, I let the humanities and social sciences have their say in this synthesis, often through targeted extracts from their research work. Although some researchers in these disciplines have been working on digital agriculture for several years, the subject has really exploded in the literature over the last 4-5 years (just look at the publication dates of the articles cited in the bibliography). The human and social sciences point to numerous ethical, social, societal, cultural and political issues that the historical agricultural players and the new digital entrants do not seem to have grasped. Or, said perhaps a little less naively, that these actors do not really want to consider insofar as these issues are not totally consistent with their objectives and personal interests.
Let’s be clear, this article is not intended to totally demonise and discredit the use of digital tools in agriculture. I am still very much involved in this field, but I aspire to a digital world in which the values of inclusiveness, knowledge sharing, collaboration and emancipation will be at the heart of the concerns of the agricultural sector. It is time to stop asking what these technologies are, but rather to look at what they do, what they promote, what they imply and in which system they fit. The subject of digital in agriculture is not simply technical. It is much broader than that and it is time to realise this…
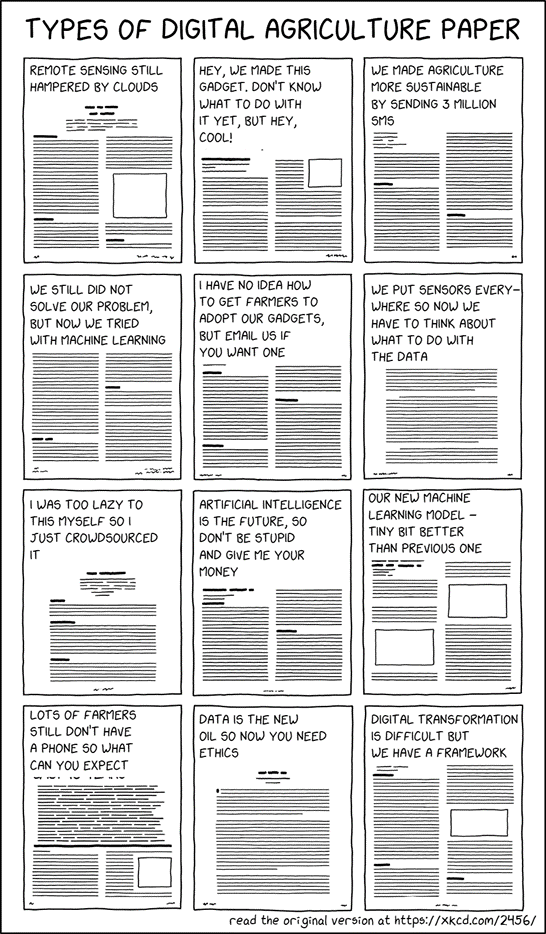
Discourse analysis and the digital rush
The dominant narratives of the Agtech ecosystem
The dominant narratives use powerful images to frame society’s perception of what is currently at stake. The most common image is undoubtedly that of scarcity. Combining a set of theories, numbers and keywords about the pressures on ecosystems induced by population growth, scarcity narratives call for urgent responses (Gras and Cáceres 2020). The proposed statement and framework are clear: the world’s population will increase significantly by 2050, and global food production will need to increase by the same amount while reducing its environmental impact and using roughly the same amount of land currently under cultivation, in order to keep the world fed and secure (Miles 2019). These narratives of scarcity, with varying degrees of nuanced forecasts of a catastrophic future, cement the discourses and literature of the agricultural digital ecosystem.
Given its prevalence and stability over time, this framing of the future of digital tools in agriculture can be seen as a kind of master narrative that outlines how food and ecosystem issues are viewed today. The texts published by the main international bodies – World Bank, FAO, OECD – effectively present digital technologies as the technical solution to future food insecurity (Lajoie-O’Malley et al. 2020). The discourses of Agtech and Foodtech actors are punctuated with references to these narratives of scarcity to justify and legitimise the development of their solutions. How many times have we heard the figures put forward in the introduction to conferences, seminars and workshops of the digital ecosystem in agriculture on the increase in food demand in the years to come? The emphasis on the positive impacts of digital agriculture in much of the existing scientific literature may indicate that this master narrative is also operating in this literature (Lajoie-O’Malley et al. 2020). A recent analysis of the sentiments and emotions associated with discourses on precision agriculture on Twitter seems to support the idea that these technologies will be useful in addressing the challenges facing the agricultural sector (Ofori and El-Gayar 2021).
Using this forward-looking vision, the future becomes the cause and justification for some form of action in the here and now. The dominant narratives describe scarcity not as absolute but as relative to the way resources are used. From this perspective, the current problems of agricultural models and those created by the use of digital technologies could thus be overcome by intelligent adjustments to existing technological solutions (Gras and Cáceres 2020). The promoters of digital technologies see them as a means for agriculture to increase the effectiveness, efficiency and productivity of its farming itineraries and operations. The slogans commonly used are quite evocative, particularly the most frequently used one “the right dose in the right place at the right time”, which is sometimes supplemented or updated with other superlatives. These strengths are also found in the recent definition of Precision Agriculture by ISPA (International Society of Precision Agriculture) researchers, who consider it to be a management strategy aimed at guiding modulated plant or animal management decisions to improve resource efficiency, productivity, quality, profitability, and sustainability of agricultural production.
Digital technologies in agriculture are often presented in the popular press, industry marketing and technical literature as a revolutionary answer to global food insecurity problems – a kind of disruptive, forward-thinking, and largely positive force that would change agriculture for the better. Some would go so far as to see these digital tools in agriculture as presaging a new green and/or industrial revolution (Miles 2019). Digital technologies are thus promoted as novelties, rather than as an extension of existing farm management strategies. Indeed, early adopters of new technology are regularly celebrated in the media for their expertise in new technologies. This narrative of novelty, which organises technological responses, is that of the innovative farmer, or in other words, a farmer who is always willing to adopt cutting-edge technologies and take informed risks (Gras and Cáceres 2020). The integration of digital technologies into the physical infrastructure of contemporary agriculture has been enacted and coded by subtle changes in farmers’ subjectivity. The imaginary of the ‘good farmer’ (more on this later), as promoted by dominant narratives, is being transformed from a figure who is supposed to know his land to a figure who knows his land digitally. The image of the good farmer, commonly deployed to encourage the adoption of these digital agricultural technologies, appears to be increasingly data savvy (Kuch, Kearnes, and Gulson 2020).
In all these imaginings, we can also find the idea that technologies will help solve the problem of climate unpredictability. By helping to forecast disasters or predict yields, these technologies should enable automated predictions (Lajoie-O’Malley et al. 2020). The image of nature as unpredictable, uncertain and a source of risk, while becoming predictable thanks to digital tools, tends to cast doubt on the state and reliability of conventional knowledge on agriculture. In agriculture, the production of new ‘precision’ data is accompanied by, or in some cases dependent on, the use of sensors to increase the certainty of knowledge and decisions (Kuch, Kearnes, and Gulson 2020). In this sense, data-driven agriculture is seen as a way to mitigate the risks to agricultural productivity caused by lack of predictability (Lajoie-O’Malley et al. 2020). The promotion of precision farming systems, however, strategically presents digital technologies as decision support tools, increasing the observation and decision-making capacity of farmers (Stock and Gardezi 2021). The development of new data infrastructures in these areas is heralded as providing the conditions for new forms of knowledge, practice, evidence and valuation.
The power of language and imaginaries
It is important to understand not only the technical dimensions of innovations, but also the kinds of imaginaries they express (Duncan et al. 2021). Our imaginaries do not come out of nowhere. Rather, they draw on deeply embedded stories that depict a world with rules and norms that we have set for ourselves. And these imaginings are rooted in a shared past. This shared past and imagined future take shape through the dominant narratives around technologies. These dominant narratives – those of scarcity discussed above, for example – recur in public themes and discourses that refer to well-known stories and even legends. Dominant narratives, whenever used, offer references to a shared tradition, and thus express a collective way of thinking and, subsequently, a way of life (Lajoie-O’Malley et al. 2020). Dominant narratives bring together social values, beliefs, and knowledge that help to normalise, reinforce, confront or challenge a given state of affairs (Gras and Cáceres 2020).
In giving substance to these imaginary worlds, language and the simple choice of words have very strong implications. In the media, at trade fairs and seminars, we hear talk of intelligent agriculture, agriculture 4.0, connected agriculture, precision agriculture, agriculture of the future, and digital agriculture. What futures and imaginaries do these lexical fields evoke in the heads of those who hear them? How do these words resonate in our heads? Are we to believe that a farmer who does not use these so-called innovative technologies is not intelligent, works like a pig, or is terribly behind the times? It is perhaps not even certain that, in the mouths of those who use these expressions, there is any desire to aspire to a completely technological future. Nevertheless, the impact is present and the imagination is constructed.
Discourse analysis allows us to highlight the power of words and language. And this is what the disciplines of the social sciences and humanities have been working on for several years. Discourse analysis can reveal how seemingly simple and innocent language can in fact have powerful effects on perceptions of right or wrong, or perceptions of inclusion or exclusion. Perceptions that, if left unanalysed, would be completely accepted by the population and invisibilised in common language, and thus never questioned. Discourse analysis challenges the fundamental framing of ideas that might otherwise lead to the maintenance of the status quo and the perpetuation of existing inequalities in existing agricultural models. Discourse analysis differs from current approaches in that it addresses the source of the adoption problem (value conflict, culture clashes, lack of trust, lack of participation), rather than the symptoms (lack of awareness, lack of information, lack of skills) (Fleming et al. 2019).
Discourses work to establish norms and rules and act in opposition to other competing discourses. Discourses are therefore dynamic and constantly changing. By becoming aware of how a discourse works, it becomes possible to conceive of how that discourse might be different, or to start rejecting it altogether. Everything we do as individuals is part of a discourse. Discourses are therefore influential social constructs that need to be examined, particularly in relation to behavioural change towards the extensive use of technological tools and solutions in agriculture.
Imaginaries to be deconstructed
A neo-Malthusian discourse centred on techno-solutionism
Thomas Malthus is a British economist who became known mainly for his theories – referred to as Malthusian or neo-Malthusian theories – on the links between a very strong increase in population on the one hand, and resources or production that would not increase as quickly on the other. According to Malthus, by not controlling population growth – notably through birth control and delaying the age of marriage – we would inevitably face demographic catastrophes. As discussed above, the dominant narratives of the digital ecosystem, both in the mouths of stakeholders in the field, but also in international texts (World Bank, FAO, OECD) present digital agriculture through a neo-Malthusian and techno-progressive prism, as the technical solution to future food insecurity caused by population pressure, growing food demand and environmental vulnerability (Lajoie-O’Malley et al. 2020) (Ravis and Notkin 2020). The analysis of the scientific literature also shows that the research themes of the Agtech ecosystem are increasingly technology-oriented. A recent mapping of the scientific literature in precision agriculture, for example, identified three main clusters of keywords, one of which was specific to ‘technology’, a second on ‘indices’ and ‘image analysis’, thus indirectly referring to technological tools for vegetation monitoring, and a third on ‘soil’ and ‘spatial variability’ (Pallottino et al. 2018). Even more recently, by analysing the keywords of a large number of scientific publications on the same topics, a group of authors identified five major research streams, namely climate-smart agriculture, variable rate technology, remote sensing, internet of things and machine learning (Bertoglio et al. 2021) – topics again largely dominated by technology.
Current key actors envision future food systems with a focus on maximising food production through technology (Klerkx and Rose 2020). This vision reflects a long-standing discourse on the role of technology in food systems innovation, which starts from the controversial assumption that increased food production leads to improved food security (Lajoie-O’Malley et al. 2020). Food security has always been high on the list of global challenges that technology could address, but it now seems poised to emerge, at least temporarily, as the dominant issue of agricultural techno-solutionism. Madeleine Fairbairn and Julie Guthman argue that the current primacy of interest in food security is not entirely coincidental. Food security would be a more appropriate framework in the sense that neo-Malthusian productivism would always have been a dubious foundation given the prevalence of overproduction in agriculture (Fairbairn and Guthman 2020).
It is interesting to realise that the statistics and figures traditionally put forward as part of dominant narratives are a key discursive tool used by dominant institutions, individuals and actors with their own ways of thinking about food security. Isobel Tomlinson argues that reference documents, particularly the 2006 FAO document (World Agriculture: Towards 2030/2050), would be misinterpreted (Tomlinson 2013). She insists that the FAO report does not explicitly or implicitly state that global food production must increase dramatically by 2050. The modelling work on which the report is based would not have a normative agenda, and the report’s assumptions and projections would in fact reflect the most likely or probable future, but not necessarily the most desirable or the one that should be (Tomlinson 2013).
A common theme in World Bank, FAO, and OECD texts is that of inevitable agricultural change, with the underlying assumption that digital technologies would bring about positive transformations in the agricultural sector. The message, both implicit and explicit in these texts, is that societies risk not ensuring the sustainability and security of the food system if they do not adopt digital agricultural technologies (Lajoie-O’Malley et al. 2020). Most texts also convey the idea that digital tools will inevitably transform small-scale farming. This transformation, described as inevitable and driven by technological innovation, is mostly presented as necessary (Lajoie-O’Malley et al. 2020). Still on these same documents from international institutes, Alana Lajoie-O’Malley and her team also note that when the benefits of digital tools in agriculture are mentioned, they always go beyond simple food production. In particular, there are many claims of ‘increased employment in rural areas’, ‘increased income for rural people’, ‘increased living standards’, or ‘increased disposable income’ (Lajoie-O’Malley et al. 2020). In this way, the so-called digital revolution in agriculture could be contrasted with the Green Revolution, the latter being portrayed as mainly technological.
The rush to make everything smart (referring to smart agriculture or smart cities) is taking place all over the world (Fraser 2021). Technological optimism leads to the belief that new technologies lead not only to technical progress, but also to social, political and even moral progress. Technological optimism can even be traced back to the Enlightenment vision of progress with the idea that progress would be closely linked to the accelerating pace of scientific and mechanical innovations (Lajoie-O’Malley et al. 2020). In their analysis of World Bank, FAO, and OECD texts, the authors point out that technological optimism is manifested in visions of farmers in remote areas of Africa using smartphone applications to manage their farms, implement ecosystem services, and meet the needs of local communities. Again, these reports would show that technologies are necessarily embedded in social contexts. In the texts, priority is given to the argument that farmers become part of existing global food systems through the use of these digital technologies, accessing digital tools and infrastructure and producing more food (Lajoie-O’Malley et al. 2020). These organisations have very positive visions of the digital agricultural future and therefore endorse major digital transformations in the agricultural system (Lajoie-O’Malley et al. 2020)
These techno-centric narratives should be accepted with caution (Tomlinson, 2013). Indeed, note that some actors will go out of their way to protect dominant narratives, going so far as to say that the harms associated with missed use of technological tools should not be ignored – missed opportunities to use these technologies to achieve sustainable development goals are likely to be responsible for at least as much damage as leaks and privacy breaches (Espinoza and Aronczyk 2021). The rise of mainstream thinking in the Agtech ecosystem is likely to extend this discourse to the high-tech end of the innovation spectrum, sidelining other responses to food security challenges that are not technology-based. These include social responses to food security challenges that have shown that lack of access to food is rarely caused by a lack of food production, but instead is linked to unequal distribution and entitlement to the food produced due to societal inequalities. Achieving the Millennium Development Goals (MDGs) on food security would require not only producing more food, but also tackling under- and over-consumption. Alistair Fraser is amused that some people see data as a fully-fledged agricultural cash crop, when data cannot be eaten and malnutrition currently affects about one-seventh of the world’s population (Fraser 2021). Technological optimism tends to reify technologies and obscure the complex social or socio-technical causes of complex problems. Neo-Malthusian approaches to food systems circumvent population-resource contradictions by increasing production, which essentially serves to reinforce the legitimacy of existing institutions by providing a technological substitute for social reform (Lajoie-O’Malley et al. 2020). It should also be added that a techno-centric narrative diverts attention from other ideas such as the degrowth paradigm, which sees existing economic structures as the main reason for food insecurity and environmental degradation (Klerkx and Rose 2020).
To be meaningful, the recurring calls for increased food production must be placed in the broader context of current production and distribution systems, which now waste a very large share of the food produced. The issues of hunger, nutrition and culture normally grouped under the umbrella of food security are not issues of simple scarcity; they are largely issues of socially, politically and culturally shaped distribution – both of food itself and of power more generally. Issues of production and waste are also issues of cultural norms, particularly those that link authenticity, masculinity, vitality and wealth to the slaughter and consumption of other species (Lajoie-O’Malley et al. 2020). Increasing food production through technology, particularly in the developing world, is not a solution to this problem in itself. While technology is important for boosting productivity and has indeed contributed to improved food security and prosperity, simply producing more food does not guarantee better food security for marginalised groups (Klerkx and Rose 2020). It is clear that no single approach will be fully effective in addressing food insecurity (Garnett et al. 2013) and that it would be unfortunate if there were not also incentives to increase food production. But the overall context of current production and distribution systems cannot and should not be forgotten.
A very or overly Agtech perspective may risk focusing our attention on too specific things rather than on the wider food system. Such a focus can cause us to miss the environmental and social trade-offs of non-climate related agricultural change and, in so doing, lead to inappropriate solutions or even missed opportunities for sustainable development within our food systems. Impressive narratives of benefits in terms of yield, soil improvement or water use efficiency can lead to the scaling up of technologies and the setting of ambitious adoption targets, but can also mask a multiplicity of context- or farming system-specific costs, trade-offs or risks (Whitfield, Challinor and Rees, 2018). To address the urgent need to build resilient, sustainable and just food systems capable of feeding a growing population, a systems approach invites us to reflect, for example, on the globally interconnected nature of local production, consumption and waste (e.g. through markets and trade); the dependence and impacts of food supply on changing ecological and climate systems and services; and the role and movement of knowledge, information and values through nodes of decision-making at various scales (Whitfield, Challinor and Rees 2018).
The contemporary discourse on precision farming tends to focus on emerging digital technologies rather than integrating them with long-standing precision management approaches. Dominant narratives focused on novelty and innovation ignore the fact that the most tangible gains on farms may well come from better implementation of simple ideas that we already know work (Klerkx and Rose 2020). The dominance of emerging innovations associated with digital technologies may divert money and attention away from currently feasible technologies (or the design of those technologies) that could make a difference now. Rose and Chilvers (2018) argue that there is an ecology or spectrum of innovations in agriculture, ranging from emerging high-tech (e.g. robotics) to more mundane low-tech products (e.g. a set of scales to weigh livestock) (Klerkx and Rose 2020)
Some authors even see this techno-centric vision of agriculture as a way of overcoming the legitimation crisis of the hegemonic global model of agricultural production and consumption (Gras and Cáceres 2020). As socio-political constructions, narratives are unstable and contested, and therefore involve a constant work of legitimation (Gras and Cáceres 2020). Narratives would essentially be involved in the functioning of the hegemony, obscuring the critiques that challenge the dominant organisation of agri-food systems, while showing that with the appropriate adjustments it can adequately address environmental issues. Emily Duncan and her co-authors add that much work in the field of precision agriculture is concerned with studying the spatial and temporal heterogeneity of production factors in agricultural plots, but in a way that legitimises and re-roots the industrialisation of agriculture (Duncan et al. 2021).
From an environmental perspective, one can also observe the tendency, following the language of the Agtech ecosystem, to adopt an overly narrow concept of sustainability, in which issues of water, biodiversity, social equity, livelihoods become peripheral with the risk that trade-offs in these additional dimensions are overlooked (Whitfield, Challinor and Rees 2018). These phenomena can also be found on the ground when technological innovations are deployed without regard to the broader societal, economic and ecological conditions in which farmers operate, prioritise and make decisions (Whitfield, Challinor, and Rees 2018) (Fraser 2021).
It is easy to be seduced by a techno-centric solution to a problem presented as simple (Rose et al. 2021). Strategic for the construction of environmental policy, scarcity narratives simplify and obscure the links between heterogeneous elements related to the environmental threats posed by industrial agriculture, the actors who have the power to solve them and the types of knowledge that support them (Gras and Cáceres 2020). The challenges facing the agricultural system are not simple – on the contrary, they are extremely complex and intertwined. H.L. Mencken puts it very explicitly: ‘For every complex problem, there is an answer that is clear, simple and wrong’. It is important to note that the neo-Malthusian framing used in mainstream narratives is not inevitable. It is the product of human choice. Neo-Malthusian framings of food issues have been challenged throughout the long history of Malthusianism and neo-Malthusianism (Lajoie-O’Malley et al. 2020).
The risk of self-fulfilling prophecies
Sociologists and economists approach the explanation of present events differently (Carolan 2020b). Sociologists see present events as an end result emerging from the past, whereas economists tend to reason backwards from the future: decisions are explained by the present value of expected future rewards. According to Michael Carolan, it would be difficult to argue with the fact that sociology places considerable analytical and conceptual emphasis on the past, in contrast to mainstream economics, which is said to be very forward-looking and future-oriented (Carolan 2020b).
This forward-looking, very future-oriented view is not surprising for the agricultural sector, however. Farming is inherently a forward-looking activity, conditioned by activities driven by visions of impending hunger and other basic needs, by anticipated seasons, and by its own mortality – for example when it comes to finding a successor to the farm (Carolan 2020b). But there are different ways of imagining these futures with, on the one side of the spectrum, hoarding, which consists of subtracting a part of direct goods to reserve it for future consumption and which implies foresight and abstention from consumption, and, on the other side of the spectrum, capitalist accumulation, which leads to hoarding indirect goods for productive use. The first imaginary, based on solidarity, reciprocity and simple reproduction, is very different from the second, much more capitalist, distant and calculable, which is based on a logic of calculation, profits and markets, with examples such as crop insurance or futures markets (Carolan 2020b).
These acts of anticipation are far from innocent. They presuppose capitalist futures and imaginaries. For example, it would be absurd to buy thousands of euros worth of agricultural or digital equipment or to make costly capital improvements (e.g. irrigation systems, buildings, fences) without any added value and/or profit behind them. Not to mention that the very ability to buy these expensive facilities would not be possible without credit, which would not exist without fictitious expectations of what the future will be. These acts of anticipation tend to emphasise the need to anticipate the lives to be valued and thus value those lives, thus pushing the very ends initially used to justify particular actions into the present (Carolan 2017). With this anticipatory view, there does seem to be a real risk of abandoning some forms of life in order to protect others. When what is valued includes increasing food production and demand, current consumption patterns and all that implements them seem to be valued (Carolan 2017).
Economic forecasts make speculative future economic value very palpable (Duncan et al. 2021). Some authors go so far as to explain that economics is a discipline that aims to generate and create worlds, rather than simply describe them (Carolan 2020b). Michael Carolan gives a telling example with the labour requirements of the agricultural sector. When farmers imagine a future countryside with no available labour, whether due to immigration laws or a booming economy, and make investments in anticipation of this future, these farmers help to make the countryside real in their image. This anticipatory vision helps to make real a rurality that lacks available labour but also a countryside that lacks migrant and immigrant labour (Carolan 2020b). These acts of anticipation can be seen through the prism of a sociology of expectations in which imaginations, expectations and visions are generative; technologies make promises, often around their ability to realise monetary value (Duncan et al. 2021).
We can then ask to what extent the dominant narratives could actually be considered as self-fulfilling prophecies and to what extent the concepts and keywords widely used by the actors of the Agtech ecosystem would create an imaginary that is most likely to happen. Laurens Klerkx and his co-authors raise in particular the question of dichotomies between several very technology-oriented lexical fields: smart farming versus dumb farming, digital farming versus analogue farming, or farming 4.0 versus farming 2.0? (Klerkx, Jakku, and Labarthe 2019). This is not to suggest that anticipatory action is inherently bad. We cannot avoid anticipating the present. Nevertheless, Michael Carolan invites us to increase our critical sensitivity to the self-fulfilling nature of what anticipation means (Carolan 2020b). Rather, any normative judgement should revolve around understanding what is protected, and conversely destroyed and abandoned, by whom and with what effects (Carolan 2017). Some anticipatory arguments may nevertheless seem strange in the sense that we are ultimately talking about something that has not yet happened and may never happen.
A normalisation of the current system
There is no doubt that a variety of digital agricultural technologies have emerged over the past 30 years. While it is true that some of these technologies have led to real efficiency gains – geo-positioning technologies in the digital ecosystem come to mind – many authors question the ideas of novelty and revolution generally attributed to these technologies. The rhetoric around the efficiency of digital technologies (reducing environmental impact or input efficiency) is not dishonest, but it is incomplete in the sense that it does not provide a long-term solution to the impending crises in the global food system (Ravis and Notkin 2020). Generally defined as smart, these technologies show how the principles of industrial agriculture are re-articulated into a more rational and efficient way of farming that can mitigate biophysical and ecological problems and adapt to climate change (Gras and Cáceres 2020) (Bronson and Knezevic 2016).
The rise of digital technologies in agriculture is seen by some as a continuation of a process that dates back to the Green Revolution, namely the reconfiguration of agrarian life in order to increase profits (Ravis and Notkin 2020) (Stock and Gardezi 2021). Precision agriculture in particular, according to these authors, would be better understood as an intensification and evolution of the dominant modes of relationship structured by the capitalist organisation of production. The figure of precision agriculture and its algorithmic bent, presented as revolutionary, would have the rhetorical effect of normalising the intensive and destructive industrial production of agricultural products that is largely responsible for the social and environmental problems it is supposed to solve in the first place (Miles 2019). According to the latter author, precision agriculture would represent to the present what farm management, mechanisation, hybridisation and artificial inputs represented to the past: a movement to further transform objects (and now activities) into discrete commodities, to extend the reach of capital and to accumulate whole new geographies of possibility to the logic of the market. According to Timothy Ravis and Benjamin Notkin, the generation of profits in agriculture would depend in part on maintaining inputs for production and artificially cheap food. And digital technologies, by perpetuating an unsustainable diet of cheap food, would support the continued expansion of an equally unsustainable global urban system (Ravis and Notkin 2020).
Most digital technologies can be seen as extensions of general categories of tools, rather than entirely new types (Duncan et al. 2021). The promotion of novelty itself helps to fetishise the technical components of agricultural digital technologies, promising that connected technologies will bring value not only to traditional agri-food stakeholders such as farmers, but also, beyond the agri-food sector, to sectors such as finance and technology. More importantly, Duncan and her team note that the basic mechanism by which these technologies are expected to deliver efficiency and resilience gains has remained the same. These supposedly new technologies are embedded in a management philosophy that is not: the optimised management of specific agricultural areas through the use of field data (Duncan et al. 2021). Input optimisation allows the continued use of environmentally damaging chemicals and practices that would otherwise be abandoned if their effects were not actively mitigated. The externalities produced by the use of these inputs are, according to these authors, the unvalued and undervalued costs of industrial capitalist agriculture. While optimisation, facilitated by digital technologies, limits the short-term damage of unsustainable practices, it also makes these practices more politically and financially permissible. Thus, by passing off unsustainable practices as sustainable, the need to adopt more sustainable and socially just practices is delayed.
By focusing on input management, these technologies would promote a limited interpretation of sustainability, i.e. one that is still dependent on non-agricultural inputs, and not open to a more radical shift towards permanent sustainable practices (Ravis and Notkin 2020). The standardisation of the environment by moving towards monoculture was historically necessary for large-scale farming machines, and was as much a precondition as a product of industrial agriculture. Many of the recommendation maps constructed by Agtech service providers only make sense if one adheres to a rigid conventional farming strategy (Bronson 2019). Despite the abundant academic literature from the early 21st century that discusses the drivers and development of precision agriculture, industry and popular press discourse continues to situate digital agricultural technologies in the field of radically different options, distancing them from this longer history of site- and time-specific management approaches in agronomy (Duncan et al. 2021).
Emily Duncan and her team point out what is missing from the potential problems and barriers that digital technologies are supposed to address (Duncan et al. 2021). Dominant discourses mainly sidestep how industrialised food production methods (e.g. intensive use of fertilisers and fossil fuels, monoculture, huge numbers of livestock) create these challenges in the first place (Ravis and Notkin 2020). The discourse of precision agriculture, and more generally of digital, tends not to be explicit about managing policy change or ensuring equitable food distribution, nor is it concerned with the kinds of food that need to be produced to ensure sustainable and nutritious diets. The current debate continues to promote the narrative of sustainable intensification to meet the growing demand for food in a changing climate (Duncan et al. 2021).
Timothy Ravis and Benjamin Notkin go even further and add that digital technologies also underpin and perpetuate a rampant urbanisation – the authors refer to this as ‘extensive’ and ‘concentrated’ urbanisation (Ravis and Notkin 2020). Concentrated urbanisation is the moment of agglomeration where the material flows of global capitalism accumulate in cities, megacities and mega-regions. On the other hand, extended urbanisation is the moment when remote territories are enclosed and transformed into operational landscapes that transport energy, materials and food to the accumulation zones. Both moments cause and are caused by each other: the urban unfolds into the countryside just as the countryside retreats into the city. Global capitalist urbanisation is a process of displacement and consumption of the material world, involving both fragmentation and homogenisation. According to these authors, agricultural digital technologies pursue the centralisation of knowledge and economic power by facilitating the transformation of vast territories into operational landscapes that provide material, energy and labour for a rapidly expanding urban system (Ravis and Notkin 2020). Agricultural digital moves agricultural knowledge production, and subsequently agricultural decision-making, off the farm, and indeed, out of agrarian areas entirely (Ravis and Notkin 2020). The humans of computing capital – the programmers and analysts who develop the digital tools and algorithms – are clustered in cities. And so it is in the city that the analysis and processing of data is carried out. Nevertheless, the players in the Agtech ecosystem are urban not because of the location of their offices, but because of their role in the urbanisation process. The urbanisation – the off-site consolidation – of agricultural knowledge through the data capture of the digital ecosystem in agriculture, and the urban trend of IT capital, then urbanises agricultural practices and allows urban businesses to influence agricultural production from a distance. This transformation of the hinterland takes place through instructions, incentives, contracts and conformation. Through these direct and indirect processes, digital technologies would have the potential to reconfigure culture, land and labour in the interests of accumulation in urban non-farm sectors (Ravis and Notkin 2020).
It is interesting to realise that many of the companies in the Agtech sector do not necessarily have connections to the agricultural world. The imaginings and promises of this ecosystem make it particularly attractive to professionals outside of agriculture, both those who want to transfer one of their technologies to a new sector, but also those who feel the tide turning and don’t want to miss out on an opportunity to make a profit. This is what is driving some of the biggest multinational tech companies such as Amazon, Microsoft, Google, Oracle and Cisco – and the funds invested in these companies and the consultants they hire – towards digital agricultural technologies (Ravis and Notkin 2020). It should also be noted that these technologies have not escaped the attention of the main players in the agricultural and agri-food sectors, many of whom are looking for the next unicorn – in this case a company valued at more than one billion dollars on the stock market (Klerkx and Rose 2020). The development of many of the digital technologies that have been touted as new so far suggests that there is a potential playground for a number of start-ups, even if the large companies still seem to dominate the sector. While these trends indicate the potential to disrupt historical patterns of control in agricultural technology, it is important to recall that the early phases of hybrid seed research and agricultural biotechnology were also characterised by a high number of start-ups, which were eventually acquired by the big players as mergers and acquisitions continued at a steady pace from the 1970s to the early 2000s (Clapp and Ruder 2020). Today, many start-ups revolving around robotics and artificial intelligence are rapidly being acquired by agribusiness and distribution giants such as John Deere, Case IH, or Bayer (Rotz, Gravely, et al. 2019).
Corporations have used their economic power to control the direction of technology’s usefulness to agriculture and to shape the narrative to focus only on its positive aspects (Clapp and Ruder 2020). Emily Duncan introduces the concept of the ‘waiting consumer’, in this case the farmer, who should be willing to pay for the arrival of digital technologies, and who would constitute the future smart agricultural economy (Duncan et al. 2021). These companies are also directing research for technology improvement only towards its most profitable components, rather than towards other potentially more sustainable agricultural models (Clapp and Ruder 2020). Indeed, it is very likely that projects around technologies with the greatest potential for profit maximisation will be funded – the huge hype around artificial intelligence tools comes to mind. Unchecked, it can be expected that research on artificial intelligence will be directed towards applications where funding and commercial interests lie (Vinuesa et al. 2020).
Digital technologies are not neutral
We cannot and should not say much about technological forms when they are abstracted from their networks, practices, affects and discourses. Technological devices are never simply independent objects; they are always relational in their essence. The functioning or non-functioning of a technology is then the result of how it is embedded in wider hybrid socio-technical networks (Driessen and Heutinck 2014). In one of his works, Michael Carolan takes the example of programming, explaining that there is nothing intrinsically wrong with coding. When code is integrated into (non-proprietary) assemblies, it can generate liberating effects, while in other assemblies (e.g. proprietary) it can evoke a sense of being in a straitjacket (Carolan 2017). In another of his works, Michael Carolan uses the philosopher Foucault’s concept of ‘dispositif’, reminding us that we need to decentralise things (e.g. a robotic milking parlour) and consider objects as a completely heterogeneous set of discourses, institutions, architectural forms, regulatory decisions, laws, administrative measures, scientific statements, and philosophical, moral and philanthropic propositions (Carolan 2020b).
It is therefore important to understand that digital technologies do not exist but become. These technologies do not stand alone and do not come out of nowhere, they are both a driver and a consequence of politics. The opaque algorithms and predictive tools used in conventional agriculture have been given a distinct veil of neutrality (Carolan 2017). The governance of digital technologies is part of urgent needs solutions, mainly aimed at improving market access. Carla Gras and Daniel Caceres remind us that the links between technological and market-driven changes are embedded in power. The initiatives of the Agtech ecosystem should then be understood as a political project linked to the companies that dominate the production and distribution of food and fuel (Gras and Cáceres 2020). Eléonore Schnebelin and co-authors add that the trajectories of digitalisation are impacted by the institutions associated with the industrialisation of agriculture, notably through the structures of advice, training and work. These trajectories would be interdependent with the associated transformation mechanisms: expansion, outsourcing, labour and intensification (É. Schnebelin, Labarthe, and Touzard 2021).
In particular, Jostein Vik and his team studied the case of milking robots in Norway to find out whether the observed structural change in the use of milking robots could be attributed to changes in Norwegian agricultural policy. According to the authors, it would be incorrect to attribute the societal change to farmers’ wishes and motivations alone. Agricultural development would tend to be highly political, and Norwegian dairy farming would be no exception. The national policy framework would not have driven the observed structural developments; rather, the policy would have adapted to them. However, it seems that the structural developments resulting from the introduction of robotic milking in Norwegian agriculture are a series of unintended consequences of farm strategies, policy adaptations, technological characteristics and milking robot capabilities (Vik et al. 2019). Michael Carolan has worked on labour issues in agriculture in the US. The large fruit and vegetable production industries are particularly dependent on immigrant labour and, as such, have historically been among the most powerful voices in the pro-immigration lobby. In his interview panel, several respondents would have admitted that the political pressure historically exerted by this sector, in favour of immigration, may well disappear once robotics become a fixture of their operations. A few respondents even felt that robotics would cause some companies, particularly early innovators, to change their stance and start opposing porous borders and worker visas (Carolan 2020b).
Digital technologies could also be seen as a medium through which we perceive and manipulate our world. This approach is interesting in that it avoids taking fundamental positions that either create the illusion that humans are losing autonomy and that autonomous technology has taken over all aspects of their lives, or that humans have the capacity to retain full control over the dominant power structures in agriculture (Gardezi and Stock 2021)
The imaginary of the good farmer
Agtech companies exercise discursive power to create new social categories of farmers that influence farmers and their social identities. The dominant narratives put the innovative and modern farmer on a pedestal. This farmer, always ready to adopt advanced technologies and take informed risks (Gras and Cáceres 2020) trusts scientific knowledge and technology to improve his farming practices (Gardezi and Stock 2021). The new male images of agriculture would also be designed to correspond to a more scientific form of agriculture (Kuch, Kearnes, and Gulson 2020). The ‘good’ farmer would follow the data and not his gut (Shepherd et al. 2018) (Carolan 2017). According to the dominant narratives, it might seem that the success of these farmers lies in having changed over time. Indeed, farmers who are early adopters of a new technology are regularly celebrated in the media for their skills with new technologies. Indeed, according to Kelly Bronson and Irena Knezevic, it would be obvious that the typical user of digital technologies as imagined by leading agri-business companies is a farmer who is a smooth adopter of the latest technologies (Bronson and Knezevic 2016).
I have always been surprised by the way farmers were portrayed in relation to digital innovations. Several studies widely reported in the media have presented (and still present) farmers as innovators and technology enthusiasts simply because they use smartphones or connect to the internet, as if, by the very fact of being farmers, it is very surprising that these people take advantage of the rather conventional technologies used by a very large part of the population. In these imaginations, farmers are thus described as a marginalised part of the population, who live in their own world, and whose behaviour cannot be analysed like that of the normal population. This very strange type of discourse also makes it possible to justify the development of the digital sector in agriculture, since it would obviously be eagerly awaited by the main stakeholders, and therefore necessarily adopted as soon as tools and services are developed.
As recently as 2016, Goldman Sachs presented an analysis with the rather provocative title: “Ensuring that humanity cheats Malthus again over the next 100 years” (Goldman Sachs, 2016). Seen through this neo-Malthusian logic, so-called early adopters of digital technologies in agriculture are positioned to save the world while so-called late adopters are seen as a threat to global food security (Carolan 2020a). Narratives of scarcity thus portray non-users of digital agricultural tools as responsible for the declining yields that would affect a growing human population (Gardezi and Stock 2021). Farming methods – and indirectly farmers – who forgo these technologies are then implicitly described as folkloric and backward (Gardezi and Stock 2021).
The adoption of technologies shapes a farmer’s social identity and also contributes to the formation of widespread trust in these technologies. Social identity theory is based on the idea that people evaluate themselves in terms of social categories. They thus tend to distinguish themselves from other groups, or other social categories. Identification with a nominal social category, such as ‘digital technology users’, makes farmers more willing to act as a member of that group and on behalf of that group. Such collective identities imply that cooperation will be oriented towards group members and that farmers’ trust in these technologies will be formed according to salient nominal categories created by agricultural technology companies, such as ‘early adopters’ or ‘late adopters’. Farmers who use the technologies come to see digital technologies as belonging to their moral community, a community in which there are codified sets of values and norms of behaviour. Digital technologies can then be seen by these farmers as an ethical and social practice, not through a careful assessment of their risks and benefits, but through the cultural transmission of idealised values of what it means to be a good farmer. (Gardezi and Stock 2021)
Carla Gras and Daniel Caceres explain that these narratives serve to mask the mechanisms by which powerful agribusiness actors exert control over farmers’ technological innovation pathways. These mechanisms are said to have a disciplinary force, making farmers’ acceptance and adoption of specific technologies a personal decision. And acceptance would go far beyond the materiality of the technologies. It would convey the search for productivity and profit improvement as an objective, thus recreating the ideological role of techno-scientific norms (Gras and Cáceres 2020).
The myths of precision, data and algorithms
Algorithmic rationality
The push for more algorithmic management of the economy, culture, politics or everyday life draws on discursive imaginaries that are now well established. Computers and technology are objective, humans are fallible, and data is the harvestable raw material of truth. Reasoning is an act of judgement, and therefore involves wisdom, deliberation and subjectivity. It can be a source of disagreement and uncertainty. Rules and rationalisation, by contrast, are clearly defined, quantitative, and designed to be universal and conclusive. Where reason involves an embodied history, rationality aspires to abstract, functional, rule-bound parameters (Miles 2019). Christopher Miles introduces here the concept of ‘algorithmic rationality’, i.e. the reorganisation of industry and reasoning on the basis of quantifiable rules and standards. The author adds that the discursive narratives around this algorithmic rationality also often invoke the rhetorical trappings of egalitarianism and democracy. On the one hand, because data and computers are treated as impartial, and on the other, because data crowdsourcing – or tapping into the public for data collection – is often put forward as a more egalitarian route to truth than relying solely on experts in the field (Miles 2019).
In a process common to data capitalism, digital technologies perform what some authors call ‘epistemic harvesting’ in which physical events and actions are translated into computationally understandable information (Ravis and Notkin 2020). Land and crops produced are being digitised and farmers’ observations, as qualitative descriptions of what they see and feel, are now being transformed or augmented into data composed of 0s and 1s. To describe this transformation, some researchers conjure up images of digitised humans or data-generating machines. Not only would farmers be converted back into data-generating objects, but by generating commercially viable environmental data through proprietary technologies, they could also be interpreted as commodities (Gardezi and Stock 2021). (Miles 2019) goes on to explain that in the field of precision agriculture, acting, thinking and doing are themselves subject to commodification via datafication (this transformation of agriculture into ‘data’), in the sense that these phenomena and indiscrete realities can be translated into quantifiable and marketable functions. With digitalisation offering the possibility of capturing, accessing, managing and exchanging what was previously inaccessible, what then prevents someone from considering a farmer as a mere number growing next to another number?
With this digitalisation, the agricultural sector can be understood on ever larger scales, facilitating financial flows at all levels, and across all actors. Like all political-economic actors, finance capitalists demand readability – and the computed data provided by digital technologies in agriculture can provide it. (Ravis and Notkin 2020). Christopher Miles gives the image of a truth-telling combine by reliably generating relevant quantitative data to facilitate commodity-capital relations. According to Miles, it would be absurd to say that by expressing the starch or moisture content of a kernel of corn, the machine would tell the truth about that corn. The harvester tells the truth insofar as it is not supposed to be engaged in deliberative, contextual and positional judgements. It tells the truth insofar as it accurately generates information relevant to the conversion of maize into a commodity-logic of function, of relational values: that is, into a thing legible to capital (Miles 2019).
Digital farming thus means, or at least increases the likelihood of requiring farmers to normalise the environment (Bronson, 2019) (Fraser 2021). None of this should sound fanciful, according to these authors; the logics at work would be quite obvious. There is already pressure on producers around the world to comply with food safety standards, forcing farmers to adopt (at least the basics) digital architectures in order to stay in the supply chain. This standardisation of the environment also facilitates the quantification of ecosystem services and opens the possibility of payments for services rendered. Although (Caquet, Gascuel, and Tixier-Boichard 2020) emphasise that a better characterisation of the environment and the identification of simple measurable quantities that account for its functioning contribute to the sustainability of agro-ecosystems, it is questionable whether the normalisation and standardisation of the environment can be justified entirely by the implementation of conditional payments.
This standardisation could have other perverse effects. First, reliance on fixed standards of action could reduce actors’ creativity and experimentation, limiting their ability to discover and exploit – even by mistake – new paths (Lioutas et al. 2019). As standardisation and normalisation go hand in hand with homogenisation, there could be concerns about a rejection of diversity and variability in agricultural production systems. Thierry Caquet and his co-authors insist on the fact that for agroecology to benefit from digital technologies, it is essential that it uses biology and valorises the heterogeneities of environments encountered at different scales. Whereas agriculture sought to mask heterogeneities in order to standardise farming practices, agroecology aims to take advantage of local conditions in order to make better use of them, to distribute risks and complementarities between animals or crops (Caquet, Gascuel, and Tixier-Boichard 2020).
This translation into bits does not only convert information into material representation. The ability to move this collected information to city-based companies, far from the countryside, makes new spaces readable at a distance. In this model, knowledge is then no longer learned by farmer-actors in their locality, but is computed by machines and computing structures in cities, which then produce recommendations and instructions for farmers on how to run their farms and what to do (Ravis and Notkin 2020). Farmers are then no longer seen as the only pool of intelligence, as cognition and decisions are increasingly distributed between farmers and these digital technologies (Gardezi and Stock 2021). Fraser (2019) describes this contemporary displacement of information away from agrarian actors as data capture, similar to the land seizures that may have taken place in the past.
Still in a market logic, information, knowledge and data (e.g. on areas, crops, yields) could be seen as a way to make land investable. Investors could then calculate risks more efficiently and develop strategies to implement investment plans. This is asset-building, which requires investors to make assessments based on data about yield levels, soil quality or water availability. Alistair Fraser refers to the land grabs of the colonial period, which relied mainly on cartographic data to document elevation, river flow, soil variability or the potential existence of mineral deposits, and suggests that the land grabs of the 21st century will be based on digital knowledge (Fraser 2019). According to this author, data grabbing has become a new battleground for the future of agriculture, with data grabbing potentially amplifying global land grabbing. (Fraser 2019).
Digital agricultural data is therefore increasingly ready to provide traceability or transparency in food processing. In the age of green or local marketing, agricultural data is inextricably linked to a phenomenon that some sociologists have called the governance of standards (Miles 2019). And to note that the development of environmental regulations and private standards (such as the implementation of HVE3 certification in wine, or CRC4 in cereals) favours digitisation tools consistent with traceability (É. Schnebelin, Labarthe, and Touzard 2021). Conventional actors place more emphasis on creating information through traceability technologies in order to better comply with value chain standards and to increase consumer confidence and knowledge about products. Nevertheless, some actors outside the conventional logic are concerned about the risk of standardisation, fearing, for example, that the industrialisation of organic products will result in standards linked to or imposed by digital technologies aimed at promoting traceability (É. Schnebelin, Labarthe, and Touzard 2021).
On this logic of traceability, if everything we do and every step we take in life is tracked, recorded, and fed into evaluation systems, we lose the freedom to act independently of the behavioural and performance expectations embodied in these systems. However, it is questionable whether the food industry is really willing to push for full traceability of products on the market – processed or not. Would they be prepared to show the real price at which production is bought from farmers, thus revealing the margin made by the entire chain from producer to consumer? Would they be prepared to highlight the carbon footprint of production, thereby risking showing the sometimes incoherent and absolutely gigantic journeys of certain products around the world before they reach the consumer’s plate?
Christopher Miles thus considers that the discourse around digital technologies implements a form of algorithmic normativity, and dresses up a certain number of values positioned under the appearance of simple and quantifiable technical facts (Miles 2019)
Algorithmic epistemology
In discussing the emerging changes in the connotations of ‘algorithm’ over the last decade, particularly within discourses on Big Data, artificial intelligence and more generally on digital technologies, Christopher Miles (2019) introduces the concept of ‘algorithmic epistemology’ or, in other words, the fetishisation of information that attributes a supernatural divination to digital technology.
The greatest risks to society come not from the accuracy of digital tools and technologies, but from the absolute and almost unquestioned belief in their accuracy. This belief can lead to decisions based on unreliable evidence, potentially dangerous when implemented on a large scale, which is currently allowed by digital solutions. Misconceptions of what terms like ‘accuracy’ and ‘precision’ actually mean and unquestioned working assumptions about these same terminologies can also prevent critical examination of new technologies (Visser, Sippel and Thiemann 2021). In the same vein of the concept of algorithmic epistemology, some authors refer to the mythological dimension of digital technologies, i.e. the widespread belief that large data sets offer a superior form of intelligence and knowledge that allows us to glimpse phenomena that were previously invisible (Visser, Sippel and Thiemann, 2021). All this of course with the aura of truth, objectivity and accuracy. Oane Visser and his team suggest that there is a danger of a ‘precision trap’. They define this trap as an exaggerated belief in the precision of digital technologies that, over time, leads to an erosion of checks and balances on farms. The danger of ‘precision traps’ is said to increase with the opacity of algorithms, the shift from real-time measurement and advice to forecasting, and the increasing distance of farmers from field operations (Visser, Sippel and Thiemann, 2021).
The over-belief in these digital technologies and the data generated can also lead to a focus on predictions and correlations rather than causation. Or in other words, to push for what happens on farms or plots, without really looking at why what happened actually happened. Rather than testing a theory by analysing the relevant data, the new data analyses seek information from the data. However, no matter how large the data sets, data analysts say that data cannot speak for itself. Processing data is always an act of interpretation (Visser, Sippel and Thiemann, 2021). In addition, the popular view of big data emphasises the velocity (one of the 3 V’s of big data) with which data is collected. The generation of data in (near) real time obviously has obvious advantages, as it facilitates a rapid response. However, the predominant focus on the present can hinder the reflection and use of longer-term historical data sets, which could really be used to understand the facts and phenomena observed.
Michael Carolan echoes the thoughts of the philosopher Geertz, who worried that once we begin to let theory openly guide our investigations and name events, our empirical explorations will become matters of predestination. Under these conditions, we run the risk of only looking for data that confirms what we are trying to show and miss the details that can help us understand what is actually happening, which is necessary if we are to avoid confusing the abstract with the concrete (Carolan 2020c). Other authors would have gone so far as to admit that the ultimate goal of digital technologies would be to discover ideas – no matter how complex – without asking questions; this could be defined as a kind of digital serendipity (Carolan 2020c). Yet it would be more beneficial to encourage work that does not elaborate or confirm what we already know, but instead observes, interprets and yields to emerging knowledge (Ingram and Maye 2020). This distinction and adjustment would, for example, allow us to move beyond the current stage of precision agriculture, providing accurate data based on biologically derived information (Caquet, Gascuel, and Tixier-Boichard 2020). A shift towards causality rather than correlation would surely pave the way for precision agronomy, rather than just precision agriculture (Duncan et al., 2021).
Thierry Caquet and co-authors make a distinction between ‘measuring to know’ and ‘measuring to control’. For example, according to them, while it is easy to identify and report on the biological activity of a soil in a measurement campaign for a dedicated experiment, the same cannot be said for imagining how this knowledge can be mobilised in an action to modulate practices, assess the risk of certain impasses, or manipulate corrective factors to increase or decrease the observed dynamics (Caquet, Gascuel, and Tixier-Boichard 2020). Ensuring the articulation between interpreted observation and action depends on the metrics used, as the outputs of one stage should be the inputs for the next. It is therefore necessary to investigate how new technologies can offer promising prospects for helping to characterise the environment and its heterogeneities, to monitor the trajectories of major biological processes impacting the establishment of yield components, the closing of cycles, or the regulation of water resources in terms of quantity and quality. Data acquisition and correlations are not sufficient here. Understanding the complex phenomena in place, causality and interactions, are important to move towards agro-ecological practices.
The marketing around precision farming systems strategically presents digital technologies as decision support tools. This secondary status to human decision making is comparable to the way in which the representation of precision medicine technologies oscillates between a representation of the technologies as supporting and reinforcing existing healthcare practices and an alternative representation that presents precision medicine as a new form of healthcare in its own right (Kuch, Kearnes, and Gulson 2020). Some authors note that, despite the different definitions attributed to precision agriculture, whether as a set of tools or as a management philosophy and strategy, the dominant discourse tends to define precision agriculture by the tools used by farmers, rather than by the decisions farmers make with these tools. Even when precision farming is described as a specific management strategy, the focus always seems to be on the tools that enable it (Duncan et al. 2021). These authors are surprised that media and industry discourse is more likely to define precision farming in terms of tools than management strategies, let alone in terms of social relationships involving farmers, their advisors, retailers and input suppliers. By characterising precision farming in this way, focusing on the technological objects that will be used in the near future, Emily Duncan and co-authors conclude that the proponents of these technologies would have found a simpler way to promote precision farming (Duncan et al. 2021).
A line seems to be drawn between the disruptive power of these digital technologies in agriculture and their support of farm and farmer-specific knowledge. Given the narratives put forward, one might expect precision farming to be a revolution in the way farms operate. Yet it does not appear that these technologies will go so far as to fundamentally disrupt farmers’ decisions about their operations, mainly because such a claim would prove quite unpopular (Miles 2019). In the discourse of precision agriculture, there is an underlying uncertainty around who or what will be responsible for decision making and an ambiguity about how to characterise it. It remains important to point out that the emphasis to date has been on supporting, not replacing or displacing, the role of (Duncan et al. 2021). Emily Duncan completes her point by adding that the debate often put forward about digital technologies replacing farmers’ expertise would be misdirected in that it would miss the broader political economy of agronomic knowledge in which farmers’ expertise is already located. This rather simplistic way of reasoning overlooks the fact that farmers’ decision-making power is already dispersed. The authors argue that it was not until the advent of digital tools that farmers were no longer totally in control of their agricultural decisions regarding inputs. The latter idea stems from a rural imaginary of small farms with male heads of household making the decisions. According to the authors, farmers have long been constrained in their input decision making, due to various treadmills and lock-ins around pesticides and the squeeze on input costs and prices (Duncan et al. 2021).
How accurate is accurate?
The historical values of ‘precision’ have been presented as responsible, unemotional, objective and scientific. Yet the paradox is that the achievement of precision has been a remarkable cultural achievement establishing widely accepted conventions and norms (Kuch, Kearnes, and Gulson 2020). The socio-technical imaginary of precision – encoded with notions of accuracy, prediction, and quantification – functions to secure capital, knowledge, and people. Declan Kuch and his co-authors argue that many centralised infrastructures are reaching their limits in terms of extracted value. Precision then allows for a re-imagining of how previously unknown surplus value can be extracted in an incremental way (Kuch, Kearnes, and Gulson 2020).
Precision is, somewhat ironically, a marker of movement into a vaguely articulated future rather than a signifier of current location. Proponents of precision in multiple policy domains claim that its value lies in its future value, as new associations are made visible through various automated analyses. This shift to the future is key to the speculative political economy of data, in which assumed future use and exchange value is presented as the justification for collecting data in the present. Data is presented as highly mobile, and its sharing is a prerequisite and feature of contemporary business models and political logics in order to structure the research, development and implementation of precision technologies (Kuch, Kearnes and Gulson 2020). Precision would therefore not simply rely on the integration of digital technologies into the physical practices of agriculture. The promise of precision would be based on the expectation that new forms of knowledge – and business opportunities – will emerge from the compilation of various forms of data.
To deliver on this promise of innovation, precision must produce evidence of its own effectiveness. Developments in precision are presented as a new incremental change and digital technologies are presented both as a normative good in themselves and as an issue of national political importance. The addition of precision and the use of sensors often masks unacknowledged political commitments, such as the valorisation of highly industrialised and mechanised agricultural production processes based on the externalisation of environmental costs. For this reason, politicians are likely to overestimate the value of precision while helping to create the social, economic and political structures that allow this promise of innovation to be realised (Kuch, Kearnes, and Gulson 2020).
It is interesting to note, however, that advocates of digital technologies rarely question the supposed accuracy of these technologies. Inadequate or inaccurate functioning of the technologies, if acknowledged, is presented as a transitory problem as the technologies will become constantly more powerful and accurate. This view is also found in the social science literature, where inaccuracies are referred to as, for example, teething problems (Visser, Sippel and Thiemann, 2021).
Imaginations of precision, quality, and exceptional veracity of digital technologies have the somewhat disturbing rhetoric of attributing observed errors in data or recommendations to farmers. Errors are often assumed to come from the farmer, who is seen as insufficiently competent with the technology or as someone who would not rely sufficiently on the recommendations and advice of these technologies (Stock and Gardezi 2021). Clemens Driessen and Leonie Heutink give the example of robotics in animal husbandry (Driessen and Heutinck 2014). According to them, the general focus would have been mainly on udder health, related to the hygiene of the milking process, as the cows’ udders were not always adequately cleaned before milking. It was then conventionally accepted that increased somatic cell counts and udder infections had been reduced by improving teat cleaning by robotic milking. Where problems persist, they are now blamed on general hygiene, cow health and herd management, not the robot itself. While part of the explanation for disappointing results in digital agricultural technologies may indeed lie with the farmer, Oane Visser and his co-authors point out that the limitations of the technology itself cannot be ignored or minimised to achieve optimal results (Visser, Sippel and Thiemann, 2021). Considering the operator as the main problem reflects a decontextualised view of technologies as abstract solutions whose implementation and use problems are relegated to the human factor. (Visser, Sippel, and Thiemann 2021) point out that a digital technology cannot be understood without examining its broad socio-technical assemblage and use.
Surprisingly, the Agtech ecosystem also seems to overlook the essential efforts of farmers to make digital technologies more accurate, through calibration, validation and interpretation. (Visser, Sippel, and Thiemann 2021). The characteristics of the sensors, and their interaction with the materiality of the crops and the environment, will affect the data generated, which requires the users of these technologies to be as proactive as possible in order to allow these technologies to function properly. Some authors also talk about the importance of developing a ‘sense of error’ for users of technologies, so that they work with the technology and build a relationship between what the technologies collect and return, and what they actually observe (Visser, Sippel and Thiemann, 2021).
The large volume and granularity of data is often mistakenly equated with high accuracy and reliability. The volume of data says little about the usefulness of the data for the algorithmic task at hand. And the sensors that collect the data, apart from direct measurement errors, usually also have limitations in terms of the completeness of the collected data (Visser, Sippel, and Thiemann 2021). The dynamic evolution of the agricultural context, with countless changes in crop stages and meteorological changes during the year, poses additional difficulties for generating accurate algorithmic advice. An algorithm trained to detect weeds in sugar beets from crop images at the beginning of the season has already become less accurate a week later in the growing season (Visser, Sippel and Thiemann 2021). The large amounts of data generated by the technologies would often be precisely inaccurate because biases in the data would be easily overlooked due to the importance given to data size. The statistically highly significant results of the masses of data collected give the researcher a false sense of accuracy. Oane Visser and his co-authors give the image of ever larger haystacks created by ever larger data, haystacks in which anyone can constantly conduct expeditions that can end in spurious correlations. He added that the large size of a dataset can easily be confused with the statistical notion of a population, but in reality represents only a biased sampling of that population (Visser, Sippel and Thiemann, 2021).
The problems of inaccuracy discussed above are likely to become particularly relevant, and potentially risky, when the (mal-)functioning of digital technologies is not sufficiently corroborated by other knowledge. This corroboration can be based on farmers’ observations and experiential knowledge in the field, or on verification via alternative scientific knowledge, such as modelling and verification by dependent experts, (Visser, Sippel and Thiemann, 2021).
This is not to say that digital agricultural technologies necessarily need to be extremely accurate to be useful. It is often the lack of awareness of potential inaccuracies, and the failure to put in place checks and balances against these uncertainties, rather than the inaccuracies themselves, that cause problems. Under the right conditions, somewhat inaccurate technologies can generate good enough data for farmers to work with, while in other cases these inaccuracies can pose much greater risks. These risks tend to spread unevenly, so that – in addition to an ‘accuracy trap’ – Oane Visser and his co-authors also identify an ‘accuracy gap’, with accuracy and inaccuracies unevenly distributed across farm types; more on this later. An important point remains to regularly challenge users of these technologies on the uncertainty inherent in these tools (C. Eastwood et al. 2019)
In general, farmers do not need accurate, high-frequency data for every decision and also have limited capacity to manage data complexity (Lioutas et al., 2019) (Ingram and Maye 2020). Having a (more focused or expanded) set of choices does not necessarily clarify the actual choice (Rojo-Gimeno et al. 2019). The question arises to what extent additional precision adds value. The study proposed by (Rojo-Gimeno et al. 2019) highlights the fact that there is no single answer; the standard value of information remains very specific to each farm (Klerkx, Jakku, and Labarthe 2019). Cristina Rojo-Gimeno and her team give the example of precision breeding tools that would simply detect problems a few hours in advance compared to not using tools. This short gain in detection time may not offer any real opportunities to refine management decisions. Nevertheless, a slightly earlier detection of a problem in the herd can sometimes give the farmer just enough time to react to the problem, for example by reacting to the first signs of tail biting in a pen and thus preventing the problem from escalating. (Rojo-Gimeno et al. 2019). The authors add that the availability of more accurate information does not necessarily translate into a wider choice of available interventions, but it does allow for more targeted interventions on individual animals, batches or pens (Rojo-Gimeno et al. 2019). In crop production, one may be able to measure variability at a resolution of one square metre and analyse it at that resolution. It remains to be seen whether the farmer has the necessary equipment to control this process and manage his crop rotation at the same resolution. Understanding these practical implications around the size of the management unit is essential to provide credible solutions (Shepherd et al. 2018).
Let us conclude this section by arguing that data is not neutral. Data does not just exist, it must be generated. (Bronson and Knezevic 2016). Several authors insist that data is never raw, i.e. generated and collected without editing or any processing, but rather baked, in the sense that a degree of processing always exists, due in particular to the conditions of data collection, storage and transmission, modelling and interpretation (Lioutas et al. 2019). The trend towards digital and algorithmic mapping also goes hand in hand with connotations of sophistication, precision and accuracy. However, as has been widely argued in the critical literature in the fields of geography and cartography, maps are not neutral or accurate representations of reality, but social constructions. Data interpolation techniques, such as kriging, create smooth and detailed gradients in maps from point observations in plots (Visser, Sippel, and Thiemann 2021). Michael Carolan adds that we focus too much on models (especially agronomic ones) without taking into account that, although we have data coming out of our ears, these data themselves have blind spots (Carolan 2020c). Uncertainty and potential biases in the data could greatly affect our ability to accurately interpret the data and draw actionable conclusions, and could thereby cast doubt on the accuracy of current digital tools. (Lioutas et al. 2019). Some authors go a step further by raising serious concerns about the power of various actors to misuse data during processing to gain competitive advantages (Lioutas et al. 2019).
Digital technology is transforming the business of agriculture
Towards a change in the farming profession?
The development and diffusion of precision agriculture and digital technologies rely on an appropriation – and technological augmentation – of the figure of the farmer as an embodied environmental sensor. The good farmer, whose figure we have already introduced earlier in this post, is imagined as a subject assisted by algorithms. (Kuch, Kearnes, and Gulson 2020)
The introduction of digital technologies into farms cannot be seen as simply adding tools, all other things being equal. New roles are configured, new experiences are generated and relationships change in nature (Callum R. Eastwood and Renwick 2020). And perhaps even the nature of the animals – and humans – involved is changed in the process (Driessen and Heutinck 2014). The latter authors have worked on the case of robotics in animal husbandry, and more specifically on milking robots. For these authors, an investment in a robot is only worthwhile if it can operate at its maximum capacity. In their case study, the introduction of a milking robot would have required a profound change in the organisation of the milking process. It was not just a robot, but a larger systemic change in the organisation and layout of the dairy farm. This would be the reason why the name robotic milking would have been replaced by the more comprehensive term automated milking system (Driessen and Heutinck 2014).
Digitalisation can change the culture of farming from hands-on, experience-based management to a data-driven approach (Ingram and Maye 2020) and can discipline farmers’ work routines in certain ways, following Christopher Miles’ concept of algorithmic rationality discussed above (see section of the same name). The promise is that, whereas previous knowledge sources were based on general knowledge often derived from research experiments, digital technologies would be able to offer farmers farm-specific and local information. As such, these digital technologies would reflect a shift from generalised agricultural resource management to highly optimised, individualised, real-time, hyper-connected and data-driven management. Farmers are being called upon to adapt to a data-rich management style, which represents a shift away from traditional agricultural management systems (C R Eastwood, Chapman, and Paine 2009). These technologies transform some farmers’ knowledge capacity, or everyday social practices, including ways of knowing, doing, and being (Carolan 2020a). Farming practices are intertwined and transformed through or because of interactions with digital infrastructures.
In this model, the farmer moves from being a manager of agricultural inputs and outputs to being an operator and interpreter of agricultural data exchanges between the land and remote servers (Kuch, Kearnes, and Gulson 2020). A reconfiguration of agrarian work is taking place that turns farmers into office managers, sitting behind a computer and reading screens and dashboards, rather than farmers (Driessen and Heutinck 2014) (Gardezi and Stock 2021). Their main responsibility might even be to manage a team of specialists, which would now include data scientists (Bronson 2019). The fear is then for some that farmers will become data labourers (Klerkx, Jakku, and Labarthe 2019), furthermore proletarianised into unpaid digital workers for their data generation work through their engagement with agricultural digital technologies (Rotz et al, 2019). (Lioutas et al. 2019) add that although farm-related data relate to questions about the responses of crops and/or livestock to various physical conditions, growth factors and climatic variables, the farmer’s management practices strongly affect the information content of this data. In this sense, farmers are also co-producers of data. Therefore, they simultaneously construct big data physically, through their decisions and actions, and socially, through the different meanings they attribute to these data during their use.
The increasing distance between the farmer and what happens in the field, due to the increase in farm size, and the fact that farmers spend more time in their offices managing (part of) the farm processes from their computers and phones, reduces the possibilities for daily human observations, with consequently less room for control of data-based operations. Increasing the scale of farms and the distance between the farmer and the field carries risks, as errors and uncertainties are less likely to be detected by a farmer who rarely passes a certain field or sensor (Visser, Sippel, and Thiemann 2021). The Taylorist approach that characterises today’s agriculture breaks down the entire agricultural process into a series of discrete tasks or productive steps, such as chemical treatments, fallowing, sowing, pest control and harvesting. And the outsourcing of some of these tasks also contributes to separating farmers from farming itself. As a result, rather than being directly involved in production issues, farmers are increasingly taking on a management role to deal with agricultural contractors, develop financial strategies and/or seek new business opportunities. The simplification, standardisation and mechanisation involved in industrial agriculture make this separation more feasible. (Gras and Cáceres 2020)
However, it would be too simplistic to see digital tools only as a subtraction of the farming profession. Several studies on decision support systems reveal that these tools largely support the decision maker, rather than replace him or her, and that farmers use decision support systems not in a deterministic way to provide specific answers, but as learning tools (Ingram and Maye 2020). And Emily Duncan adds that the fact that some farmers have digital equipment does not necessarily mean that they are making decisions to implement a precision farming system (Duncan 2018). Clemens Driessen and Leonie Heutink discuss the co-evolution of the farmer’s profession with digital tools. Their understanding of this co-evolution testifies that the characteristics of cows, farmers and robots depend on how they relate to each other and to the wider fabric of the world. In particular, these authors are interested in the changes in the farmers and cows themselves, and how these affect the design and layout of the robot. (Driessen and Heutinck 2014)
For some farmers, the adoption of these technologies is seen as incompatible with their core beliefs about farming (Rose et al. 2018) (Bronson 2019). The fact that some new entrants to agriculture are using sensors and other digital systems would further reinforce this perception (Lioutas and Charatsari 2020). And there is a risk that technology will distract attention from other things that have driven farmers into agriculture – biodiversity, trust, strong communities (Carolan 2017). Research has shown the importance of physical labour, traditional farming practices and farmers’ experiences to their engagement with and understanding of their land and environment (Carolan, 2008).
The advisor and his/her relationship with the farmer
The development of digital technologies has been primarily focused on the needs of farmers, with limited consideration of the roles of other actors who influence technological innovation in agriculture (Duncan et al. 2021). And several authors insist that electronically simulating decisions without considering the agri-social systems of which land managers and farm advisory services are a part will not be enough (Fielke, Taylor, and Jakku 2020) (Callum Eastwood et al. 2019). Farm advisors, as intermediaries in agricultural knowledge and advice networks, are an essential component of any agricultural innovation system. In general, the involvement of the whole ecosystem surrounding the farmer – what (Higgins and Bryant 2020) call ‘frame congruence’ – is seen as critical to the successful implementation of technology in organisations and industries, as it creates a similar set of expectations about the role of technology in business processes or the nature of technology use. Instead, according to these authors, framework incongruence (as opposed to framework congruence) can hinder technology implementation by causing difficulties and conflicts around technology development, implementation and use.
The knowledge brokering and facilitation roles of advisors are also relevant in the context of digitising farming systems (Eastwood et al., 2019a). Nevertheless, it should be clear that agricultural technologies represent a substantial change from routine practice for those in agricultural extension and advisory roles. These digital technologies therefore create new challenges for these advisory structures to provide effective support to farmers. These challenges cluster around what some authors call the ‘symbolic practices of digiware’, or managing the proliferation and multiplicity of digitised forms and effects that characterise digital innovation. (Higgins and Bryant 2020). These service providers will need to adapt to operate at the intersection of cyber-physical systems, as increasing data-driven power is held within the digital world (Fielke, Taylor, and Jakku 2020). The increased use of digital technologies may lead to new ways of engaging with customers which, in turn, require new ways of finding and disseminating information, developing new skills, and building new networks (e.g. with digital tool providers). And this work will be all the more complex because advisors, historically experts in terms of improving agricultural productivity, now have even broader remits, whether on environmental sustainability, animal welfare, or climate change (C. Eastwood et al. 2019)
Rather, the humanities and social sciences tend to see the advisor as a ‘sense maker’ in the innovation system of digital technologies, rather than a promoter or barrier to technology adoption (C. Eastwood et al. 2019). Under digital technologies, the role of the adviser must shift from a front-office activity of gathering information in the field, to a back-office activity where advisers add their knowledge and skills to growing and varied volumes of information (C. Eastwood et al. 2019). Advisors have the ability to create hybrid knowledge where their knowledge of farming systems is combined with the outputs of digital tools. Advisors can then also act as an intermediary between the farmer and digital technologies. This role of advisors as expert users of more advanced digital farming tools is supported by several studies where advisors were operating farming systems modelling tools for their clients (Callum Eastwood et al. 2019).
The uncertainty associated with the implementation of a digital farm innovation can affect its eventual adoption. If such adoption is considered relevant and beneficial, advisors have an important role to play in managing this uncertainty by helping farmers become familiar with the nature of digital data and its interpretation (C. Eastwood et al. 2019). These authors add that, within their case study, the use of management software by advisors has been shown to increase the need for quality data among some advisors, leading them to encourage their clients to adapt their practices to increase their efforts around data capture (Callum Eastwood et al. 2019).
However, it is also conceivable that with the increasing use of agricultural technologies, the nature of the advisory relationship could evolve towards fewer physical visits to farms, and more remote monitoring of key performance indicator data via online software, at the risk of increasing the sense of isolation that many farmers already experience (Fraser 2021). Farmers still value face-to-face interactions with their advisors and agronomists, and prefer them to the cold, exclusive use of digital technologies, because of the agronomist’s knowledge of their farm, and their experience with the changes the farm may have experienced in the past (C. Eastwood et al. 2019).
Destabilising changes within the agricultural industry impact on advisers’ ability to provide advice, their available resources, their legitimacy in relation to their role, and even the relevance of their knowledge, potentially creating an identity crisis for the adviser profession as an agent of change, and even increasing feelings of insecurity in the profession. The ability of counsellors to make sense of change depends on establishing a sense of routine and predictability. The fact that digital technologies deviate significantly from usual practices and generate uncertainties in the minds of counsellors destabilises or destabilises the actors’ daily roles and routines, thus creating an impact on who they are and what they do. These risks and dangers create what (Bryant and Higgins 2021) call ‘ontological insecurity’ by destabilising existing social norms and practices (Bryant and Higgins 2021). Melanie Bryant and Vaughan Higgins demonstrate that advisers rely on two main cultural scripts to resecure their uncertainty and reflect established routines and a sense of stability about digital technologies in agriculture.
The first script is a commonly used line of argument in which stakeholders repeatedly refer to farmers’ attitudes and age as the main barrier or facilitator to the adoption of digital tools. This first type of discourse can give stakeholders a sense that some aspects of technological change are controllable, and reassure them as to how and who they think will respond to change in a particular way – for example, by seeking out the farmers they identify as likely to promote change by positioning them as innovators, while downplaying the role of others they assume are unlikely to adopt, slow to adopt, or not adopt at all. In the second script used, the focus is on easy technology – prioritising technologies that are known to be more easily adopted by farmers and have clear short-term benefits. This type of scenario allows stakeholders to get on with the job of what they already know will work, and to maintain a routine around research and technology development that avoids perceived uncertainties. One of the main corollaries is that these stakeholders are unlikely to prioritise digital technologies in their agricultural service delivery – other types of technology are easier for them to implement – and therefore less likely to encourage producers to adopt them.
The authors suggest that both of the cultural scripts discussed would be counterproductive in managing the uncertainty of innovation experienced by farmers (Eastwood et al., 2019). The focus on farmers as barriers or facilitators to adoption, and the focus on easy technology, are both inward-looking and industry-centric, with seemingly limited opportunities for collaboration. Cultural scripts limit the range of options that individuals may perceive to be available to them in specific situations and act as a barrier to collaboration and further implementation of digital agricultural technology, placing the onus on producers for adoption or non-adoption, and prioritising technologies perceived to be easier to adopt (Bryant and Higgins 2021). Although the scripts do not reflect the complexities of institutional and technological change, Melanie Bryant and Vaughan Higgins argue that these discourses provide actors around farmers with tools to re-establish their sense of self and role and, thus, their legitimacy as change agents (Bryant and Higgins 2021).
In general, (Higgins and Bryant 2020) note that each of the technology frameworks used by their interview panel reflects particular assumptions, expectations and knowledge about digital technologies and its users, as well as local understandings of the factors that may influence specific uses of the technology. The identification of these frameworks, according to these authors, provides further evidence of the challenges faced by actors around farmers in managing the diversity, complexity and uncertainty associated with digital technologies. Taking the example of work in New Zealand, (Higgins and Bryant 2020) detail how the digitisation actions of advisory structures in response to digital farming are often ad hoc, starting with adapting capabilities, practices and services to suit the needs of their clients and partners. This contrasts with a more strategic approach, which would involve fundamentally changing organisational values, allowing for greater flexibility in roles and processes and changing business models to cope with the uncertainty of digital technologies. The authors also give the example of the Australian rice industry, highlighting the diversity of ways of thinking about digital farming. In their work, the authors show, for example, that commercial agronomists and advisors are interpreted on the one hand as being central to the implementation of digital agricultural technologies at the regional level, while on the other hand they are seen as having a limited and in some cases counterproductive role in promoting technological change (what the authors call ‘frame incongruence’). Stakeholders also appeared to be very divided on the extent to which the sector should focus on technologies – such as varieties – that are rapidly adopted by producers rather than investing more in promoting the adoption of digital agricultural technologies (Higgins and Bryant 2020). The authors believe that the community would benefit from reframing the question of who or what is (or should be) responsible for the adoption of digital technologies by farmers, and in particular not simply considering the farmer as solely responsible for the adoption or non-adoption of the tools, but rather to consider a broader framework where structural and climatic factors of the farm are also considered.
A need for training and skills development
Digital technologies, if they continue to be deployed, will require new knowledge and skills from the entire agricultural ecosystem (C. Eastwood et al. 2019). While there is already evidence of adoption of some technologies, for example geo-positioning technologies, Julie Ingram and Damian Maye note that these are essentially embodied knowledge technologies that do not really require additional skills to be valued. These technologies build on the knowledge that farmers already have about how to use their machines. This is in contrast to more information-intensive technologies that will generate data or agronomic recommendations, which will require more specific skills to understand the results obtained (Ingram and Maye 2020). (Lioutas et al. 2019) also use the example of agricultural machinery, sharing that the way a farmer uses his tractor only affects it in the long term, so that the quality of the tractor remains relatively stable when the analysis is limited in time. These tractors would be in contrast to the broader digital technologies, which are constantly evolving, in the sense that each new action on the farm would generate new data, thus changing the nature of the digital technology. Farmers are then called upon to continuously adapt to the ever-changing nature of digital technologies and to develop new knowledge and skills (internal tools) in order to effectively transform the data from digital technologies into usable decisions. Indeed, authors have pointed to a growing trend among farmers to outsource precision farming services (Nguyen, Brailly, and Purseigle 2020), perhaps as a way to compensate for a lack of in-house expertise on the subject.
(C. Eastwood et al. 2019) argue that digital farming innovation has the potential to disrupt advisory practice and services by requiring a hybrid knowledge capacity that challenges advisers’ data analysis capacity. There is a real need to link predictions and metrics at the farm level, to manage the proliferation of digital attributes of farming systems, and to understand how digital data can be integrated into existing farm management decision making. The advisory ecosystem surrounding agriculture is thus also called upon to continuously improve its level of understanding and participation in digital agricultural innovation (Ebrahimi, Sandra Schillo, and Bronson 2021). This increase in the competence of these advisory actors would be all the more important as some farmers would admit to having changed their agricultural advisor because their previous advisor was not familiar with precision agriculture data processing (Duncan 2018). Other authors have highlighted the lack of availability of support and advice for the implementation of advanced digital technologies (Higgins and Bryant 2020). However, it is important to note that the need for training is not simply technical. Advice and support around digital tools requires a paradigm shift in the sense that data and recommendations are immaterial, unlike the agricultural inputs and equipment historically emphasised by these actors.
Innovative learning models may be needed to enable advisers to experiment (e.g. by testing different technologies or data interpretation processes), to engage in collaborative learning with stakeholders such as farmers and technology providers, and to seek specific and advanced training – for example, in data analysis (C. Eastwood et al. 2019). These authors emphasise the need to move away from traditional training frameworks – often in the form of technology transfer – and instead favour more reflective training approaches, associated with peer-to-peer learning. This type of development could be particularly relevant in dynamic contexts such as digital agriculture (C. Eastwood et al. 2019).
Several authors point to the risks associated with the upskilling of these coaching and advisory structures. The responses of advisers, and whether they change to play a greater role in agricultural digital technology innovation systems, will depend on the desire of the advisers themselves to change, as well as their organisational contexts, and the internal/external support they receive (C. Eastwood et al. 2019). The change process for a counsellor requires consideration of a number of factors (C. Eastwood et al. 2019) including:
- the counsellor’s professional identity which may be at risk if new practices do not match the counsellor’s perceived identity,
- the counsellor’s organisational context in that the counsellor may not support the use of digital tools in their counselling services,
- changes in professional identity,
- changes in established practice such as the frequency of advisor visits to a client’s farm, the increased use of technology and data, and the changed nature of interaction with others such as farmers and other advisors.
Advisory organisations may find it difficult to implement new knowledge into their service offerings. Advisers can also be expected to provide a service based on current scientific knowledge, which may become increasingly difficult in a fragmented and competitive advisory sector.
A final point, which has been little studied in the social sciences and humanities literature, is the need for other actors to become more competent in the use of digital technologies, namely agricultural machinery dealers and digital tool retailers and distributors. Digital technology, by actively introducing itself into these spheres – one of my last blog topics was about standards and agricultural interoperability (https://www.aspexit.com/standards-et-echanges-de-donnees-dans-le-numerique-agricole/) – requires these players to become more competent as well. The fragmentation must not be too strong between the tool designers, the resellers and distributors of these technologies, and the structures that organise the consulting around these technologies. In her study, Emily Duncan had begun to identify gaps in understanding between those who sell the tools to collect the data and those who interpret it (Duncan 2018)
A fragile agricultural model and the risk of a two-speed agriculture
Digital technologies adapted to agricultural systems that are still not sufficiently diversified
The majority of current digital tools and services are focused on a relatively small number of crops, mainly industrial and intended for export markets, whose by-products are commonly traded. These crops are often grown as monocultures, where inter- and intra-species and intra-variety diversity is very low or non-existent, and are generally grown in fairly simple crop rotations. The potential risks of moving towards specialisation (and intensification) of farms are now well identified, including the reduction of biodiversity, the loss of traditional crop varieties, and the degradation of soil and water quality (Lioutas, Charatsari, and De Rosa 2021).
The concentration of digital technologies on these agricultural productions can be understood quite easily. These simplified agricultural ecosystems allow the retrieval of data that can be more easily standardised and contextualised. Algorithms can then be calibrated more efficiently and learn more quickly – without the need to consider complex interactions between soil and plants. While the digital players are happy to collect large data streams, in reality it is quite limited data that is being collected. Limited in the diversity of the ecosystems considered (the plots and agricultural systems are fairly similar), in the agronomic relevance of the data collected (in the end, it is not so much agronomic data as regulatory data that is collected), and in the temporality of the events considered (for example, for certain head crops that do not recur very often in crop rotations, it takes many years of data to come up with something interesting).
Digital technology still considers agriculture too much as a simplified ecosystem. More complex and systemic farming practices – intermediate crops and cover crops, complex crop rotations, or permaculture – are likely to pose extremely complicated challenges to these digital tools. In a monoculture system, it makes much more economic sense to keep a whole field uniform than to drive a huge combine around several small plots. The problem is not so much that an individual farmer is not green enough (Miles 2019). The problem is with a conventional system whose underlying logic makes a market-based choice more viable than an ecological one inevitable (Miles 2019). The enormous variety of plant growth stages and weather variations currently pose considerable challenges to technologies. Climate change will greatly increase this unpredictability, with more extreme weather conditions, new plant diseases and pests. To avoid tackling this complexity, there is a fear that tools and services will become more concentrated and innovative around a very low variability of crops and farming styles. This phenomenon would be self-reinforcing as algorithms, by continuously learning about these simplified ecosystems, would be able to render increasingly accurate analyses of these same ecosystems. As a result, farmers growing the few selected crops mainly targeted by all the tools and services in the sector could benefit the most from the progress of this rampant digitalisation. To benefit from the many technologies that exist, farmers would then have to simplify their production to fit within the strict framework of what the new technologies can observe and optimise. In other words, digital tools do not yet seem to be very oriented towards farmers working outside the dominant industrial model (Bronson, 2019).
Multi-speed agriculture, fractured on all sides
We could therefore fear the risk of a multi-speed agriculture with various fractures, but all more or less intertwined. Let’s look again at the possible fractures generated, supported or even facilitated by the use of digital technologies in agriculture.
A “precision divide” where, on the one hand, we would find industrial crops in a simplified ecosystem that are largely monitored and supported by digital tools, receiving sufficiently good and precise advice and, on the other hand, more diversified crops for which the tools and advice would be of lesser quality and poorly adapted to their operations, and which would derive little benefit from the digitisation underway. For the latter crops, the advice could even go so far as to make erroneous or even totally counter-productive decisions if the algorithms – having learned on conventional crops – are suddenly transferred to these crops. Indirectly, the digital system would have a kind of right of control or veto over existing crops, a kind of right to make certain monocultures flourish and to let other plant species wither away (Stock and Gardezi 2021). Many of the organic farmers in the (E. Schnebelin 2021) panel also point out that the current technological tools are not adapted to their production systems, and may even be counterproductive. This accuracy divide is actually very much related to an ‘algorithmic divide’ defined by (Yu 2020) as the uneven benefits of algorithms and artificial intelligence. This accuracy divide is likely to deepen and become multi-layered also within the group of farmers producing the staple crops targeted by digital tools as it is likely that paid premium options will provide some users with more accurate and fine-tuned advice, while others will have to make do with basic algorithmic advice. Thus, even those farmers who would have been successful in accessing and using the new technologies could still face significant disadvantages depending on their crops and their ability to pay for the digital services. With no pun intended, these farmers might feel that they are in a system of imprecision agriculture (Visser, Sippel, and Thiemann 2021).
A ‘digital’ divide, which mainly refers to unequal access to and use of the internet (or digital technologies more generally), for example where farmers have poor internet connectivity. Problems with access to pre-existing infrastructure may be associated with a lack of comfort with emerging technologies, issues of trust in technology, or a perceived lack of use of technology for agriculture (Rotz, Gravely, et al. 2019). There remains some scepticism about the improvement of rural and regional digital infrastructure in the near future, which some authors have associated with the widening of the rural-urban divide. Indeed, one might ask whether the arrival of 5G will exacerbate these differences, as some rural areas do not yet have 3G or 4G. The rhetoric of the digital divide highlights this division between civilised users of tools and uncivilised non-users, amplified by the fact that technological changes are very rapid and not really well regulated.
A “Big Data” divide, i.e. the gap between, on the one hand, companies that decide on the data they collect and have the technology and expertise to interpret it, and on the other hand, those that do not have access to the data or have no role in deciding on the data collected or in its interpretation. The need to collect, combine and interpret data changes the distribution of power in the whole network of stakeholders around farms (van der Burg, Bogaardt, and Wolfert 2019). Stakeholders who have the capacity to develop a high level of big data competence are the most privileged (Lioutas et al. 2019). While farmers may perceive and apply big data in different ways, agricultural businesses with a higher level of value chain integration and better information may benefit more, while businesses with less access to information (due to less dense advisory networks) and less integration in the value chain, may be more exposed to risk (Fleming et al. 2019)
An ‘accuracy’ divide: namely a denunciation of all factors that cannot (yet) be measured with great precision. A preoccupation with agronomic parameters (or not) that can be measured with precision could, for example, distract farmers from deepening a systemic understanding of key crop determinants, such as soil, and environmental effects that are more difficult to measure.
A ‘North-South’ divide: (Vinuesa et al. 2020) point out that applications of artificial intelligence are currently oriented towards issues related to the Sustainable Development Goals, which mainly concern the nations where most artificial intelligence researchers live and work. These authors also consider that if AI technologies are designed and developed for technologically advanced environments, they may exacerbate the problems of less affluent nations (e.g. in food production). In a similar vein in agriculture, (Sparrow and Howard 2020) imply that digital technologies could lead to the empowerment of some groups (e.g. farmers in rich Northern countries) over others. The above findings on artificial intelligence raise serious concerns that developments in these technologies could increase inequalities between and within countries, which would run counter to the general idea of the Sustainable Development Goals. Structures undertaking such work must ensure that solutions are not simply transferred from technology-intensive nations. Instead, they need to be developed on the basis of a thorough understanding of the region or culture concerned in order to increase the chances of adoption and success. (Ravis and Notkin 2020) add that digital technologies in agriculture manifest themselves differently in the South and the North, and that until now, most of the literature on the subject would only focus on the manifestation of tools in the North, which mainly involves precision farming equipment.
The biggest gap in the adoption of precision farming tools is on medium and small farms in the developing world that do not use motorised mechanisation (Ravis and Notkin 2020). These farms are not using such technologies to improve their farming systems because research has developed very few technologies that would be profitable for non-mechanised medium and small farms, and because entrepreneurs have not commercialised the few technologies developed for these uses. The business strategy still being pursued by large companies is to try to sell simplified and cheaper versions of industrialised country technologies in developing countries. The history of technology suggests, however, that this strategy is rarely successful. (Ravis and Notkin 2020) even worry that digital technologies, by lowering the price of crops, will further exacerbate the inequalities of farmers who would not have access to these digital technologies and therefore could not potentially lower their costs or increase their yields.
Conventional wisdom holds that developing country agriculture needs motorised mechanisation, but current digital technologies are most effective when they can be used in the context of large rectangular fields. The creation of large rectangular fields in a landscape previously dominated by smallholder agriculture – particularly in a southern farming context – is highly disruptive socially, economically and environmentally. Some authors advocate that the development of specific technologies, including small customised agricultural robots could be a way to skip the step of motorised mechanisation (Lowenberg-DeBoer et al. 2019). However, there could be concerns about the risk of technology leapfrogging on such farms.
To take the critique a step further, (Ravis and Notkin 2020) call for an analysis of the neo-colonial functions of digital technologies in agriculture. The authors argue for the colonial and neo-colonial origins of some digital technologies used in agriculture and the role of development organisations in promoting them in Southern agriculture. In their view, there is still too little – if any – research on the neo-colonial functioning of digital agriculture as an industry.
A ‘Big Farm-Small Farm’ divide: i.e. the fact that digital technologies, considered expensive and supporting an intensive agricultural production model, would be more useful to big farms – which could for instance benefit from larger economies of scale – than to small family farms (van der Burg, Bogaardt, and Wolfert 2019). This divide could be seen as a kind of barrier to entry into agriculture and/or food production (Sparrow and Howard 2020). According to (Bronson 2019), digital technologies favour wealthier farms that grow in ways that are conducive to data collection – having potentially more accurate information on a more regular basis – and that make sufficient profits to afford the technology; this privilege begins with the installation of these technologies on farms. Implementing these technologies requires capital investment in sensors to acquire data, connectivity infrastructure to connect the data, and advanced machinery to use the data. The cost of such equipment would limit much of their application to wealthier or more financially secure farms (Bronson 2019). It is clear that the economic size of a farm influences the ability to invest in particular types of digital technology. This capacity can be achieved by increasing the size of farms, by collective investment or by expansion through outsourcing (E. Schnebelin 2021). Nguyen et al (2020) have shown that outsourcing is a way of gaining access to the use of such devices without investing in them.
Economies of scale are very important for robots which, in the short term at least, may offer only marginal improvements over the farming methods they replace (Sparrow and Howard 2020). As robots currently perform poorly in unstructured environments, a standard way to improve their performance is to impose more structure on the environments in which they are used. This favours companies that have access to sufficient capital to modify the landscapes and plantings of their farms or to modify or rebuild milking, mowing or feed handling facilities to take advantage of automation. It is possible that the invention of smaller, more sophisticated mobile robots could change this thinking, for example if they can be manufactured and sold at a low enough price to be accessible to small farms. For now, however, the major farm equipment manufacturers are mostly focused on automating their largest and most expensive products. If large agricultural producers are able to drive down the prices of small producers because they are better able to realise the cost savings and/or productivity gains offered by robots, this could lead to the failure of small firms and ultimately to consolidation and concentration of ownership in agriculture (Sparrow and Howard 2020). Given that globally only 6% of farmers cultivate areas larger than 5 ha, the exclusion – or at least non-inclusion – of smallholders in the race to digitise raises questions about digital inclusivity and concerns about their ability to compete in markets and, consequently, the security of their livelihoods (Lioutas, Charatsari, and De Rosa 2021).
A multi-dimensional social divide (“Urban-rural”, “Skilled labour – unskilled labour”, “Local labour – immigrant labour”): The expected growth of jobs in agricultural sciences, engineering and other high-level positions presents a concern for rural agricultural employees in particular. While it has been noted that digitalisation, such as broadband roll-out, may bring service sector jobs to the rural economy, it is unclear how jobs in primary agricultural production will be impacted. Skill-based technological change may contribute to wage inequality, as the demand for highly skilled, technology-savvy workers – with a growing demand for engineering graduates – leads to stagnation in the wages of less skilled workers (Rotz, Gravely, et al. 2019). Some authors consider that the impact of technology, and in particular automation, on agriculture over the last century has been to radically reduce the number of people employed and that there would be little reason to think that digital tools, including robotics, are an exception to this trend. (Ofori and El-Gayar 2021) insist that digital technologies will lead to net job creation, especially for the younger generation, due to the increased emphasis on technology education in schools. However, (Sparrow and Howard 2020) point out that the issue is much more complex than a simple increase or decrease in jobs in the sense that even if new jobs emerge for the construction and maintenance of agricultural robots, it is unlikely that these jobs will exist in the same places as the jobs eliminated by the robots. And several authors raise the possibility of a radically bifurcated labour market increasing social asymmetries (Rotz, Gravely, et al. 2019) (Rolandi et al. 2021).
Digital technologies may indeed be creating a new form of knowledge, both sophisticated and inaccessible to farmers, which is increasingly difficult to make sense of. As the veracity and relevance of farmers’ knowledge and expertise is questioned and new knowledge is organised through technology, there seems to be a widespread concern that farmers do not know what to do with the data generated by the Agtech ecosystem.
As digital technologies advance, it seems unlikely that agtech companies will produce technologies to improve the working conditions and training of migrant farmers, especially if farmers can be convinced to capitalise more by adopting technologies that displace labour (Rotz, Gravely, et al. 2019). In other words, current economic conditions lock employers (which in this case are farmers) into a decision between retaining manual workers or replacing them with technology. Under these conditions, a company would not be obliged to develop technologies that improve the working conditions of migrants or that support the integration of labour – fairly and efficiently – into increasingly automated agricultural systems. Thus, while farmers have expressed a desire to engage in workforce development, mentoring, cooperative labour pooling and collectivised/cooperative automation, these practices largely run counter to the economic goals of the agri-tech industry (Rotz, Gravely, et al. 2019). On the one hand, reducing the structural need for highly exploitable labour through automation may seem socially desirable. On the other, it raises questions about the kinds of obligations farmers – and nations – have to the people whose labour has subsidised agriculture for decades (Rotz, Gravely, et al. 2019)
On agricultural robotics more specifically, (Sparrow and Howard 2020) reflect on the fact that the skills required to build and maintain robots are likely to be very different from those typically possessed by people in rural areas and that, as a result, many of the jobs created by advances in robotics are likely to be located elsewhere in the longer term. The use of robots in agriculture could then lead to significant demographic changes. By eliminating the need for a significant amount of agricultural labour, there may be fewer economic opportunities for those living in rural areas in the future (Sparrow and Howard 2020). The authors go even further to imagine policy implications in that, with a potential reduction in the agricultural labour force and the number of people living in rural areas, it may become more difficult to mobilise electoral support for policies that address the needs of the agricultural sector. A key question will then be what will replace paid employment in the agricultural sector for those whose jobs have been taken by robots, and in particular how to ensure that these people have meaningful work (Sparrow and Howard 2020). Following on from this, (Rotz, Gravely, et al. 2019) ask how much of a moral obligation we have to return some of this income to migrant workers and their communities or, at the very least, to provide them with appropriate training if digital technology means that we are trading ex-migrant worker jobs for better paid technology jobs.
Let us add the concept of the ‘algorithmic’ divide (Yu 2020), born of the very existence of algorithms and technological tools. The key attributes of this divide are not specific to agriculture but are interesting to keep in mind. Some of these attributes can be paralleled with those described above. These include
- Awareness, or knowing that one is included or excluded from certain types of technologies or the outcomes of those technologies.
- Access: (in relation to the digital divide, North-South, and Large Farm-Small Farm) in the sense that not everyone necessarily has access to algorithmically enhanced technological products and services
- Affordability’: while lack of economic and technological resources may lead to inaccessibility, it may also determine the type of product and service to which a person can have access and the frequency with which that person can use the selected product or service.
- Availability’ (related to the precision divide). It is not uncommon for the specific type of product or service that an individual needs not to exist. Even if it does exist, it may involve algorithms designed by people who do not fully understand the specific needs, interests, conditions and priorities of the user, particularly in developing countries.
- Adaptability” which requires both knowledge and understanding (in addition to awareness) of technologies. In the age of artificial intelligence, the author points out that algorithmic literacy is just as important as algorithmic awareness.
All these divides have many dimensions and cover many different areas. Neither an emphasis on absolute equality nor a focus on binary divides will help society develop the laws, policies and institutional mechanisms needed to meet such a crucial challenge in the age of artificial intelligence (Yu 2020). The author, through his readings, observes that despite the most exaggerated hopes of some cyber-optimists, digital technologies will not suddenly eradicate the fundamental and intractable problems of disease, debt and disadvantage faced by developing countries. Instead, the author invites us to think relatively, asking whether certain digital technologies would improve these divides relative to a fixed baseline (Yu 2020).
To some extent, these expressions of divide imply a bipolar societal division. Nevertheless, as rhetorically appealing as divide expressions are, it is worth remembering that the emphasis on a binary divide often blurs the distinction between the different levels of inclusion of these technologies (Fleming et al. 2018). Indeed, to make matters even more complicated, an individual may be on one side of a divide based on certain categories, but on the other side based on other categories (Yu 2020). Rather than focusing on the object that is supposed to perform the automation or the skilled or unskilled humans involved, it would seem more useful to take a decentred position. This would highlight a multitude of artefacts, practices and affectivities that are not taken into account when arguments are limited to a binary position – milking robot/not milking robot, good/bad, skilled/unskilled work. It is forgotten, for example, that these transitions are also biased by qualifications. For example, many people with the skills to repair digital equipment are excluded from the labour market because they are not qualified to repair these technologies under intellectual property law and technology use agreements, as most repairs can only be done by certified technicians and dealers.
As with any period of technological transition, there will be winners and losers. While the opportunities for flexible, technology-savvy workers are particularly promising, it is essential to ask who exactly is able to access these opportunities, and who is not (Bronson 2019).
Across the supply chain, several authors argue that farmers bear the highest costs and risks of adopting digital technologies, as a bad investment can easily cost them their livelihoods. It then follows that they should receive the largest share of the benefits of the data and recommendations generated (Rotz, Duncan, et al. 2019). The latter authors speak of a new ‘sharecropping of agricultural data’. For them, many of the existing digital technologies are linked to specific data management platforms, which would imply that, while farmers still own their fields, they continue to rent their data. In this sense, farmers and farm workers would continue to bear the physical risks and impacts of agriculture on their livelihoods, while the capital gains of digitalisation would largely be extracted by data management companies. According to other authors, the costs of switching to management strategies such as precision farming would be sunk costs (Fraser 2019). Farmers would therefore absorb the start-up costs at the risk of not seeing the expected benefits. Although precision farming tools can bring gains, Alistair Fraser considers that these can be negated in the long run if improved yields result in increased production, but also in lower prices at which that production will be sold.
Conveyor belts and locks
Some knowledge emphasised over others
All the farmers interviewed by Michael Carolan thought they were more technologically literate than previous generations; sentiments which, if true, are a testament to the knowledge farmers have acquired (Carolan 2020a). However, on further investigation, this author found that farmers actually knew their plots differently, and tended to have lost or forgotten local knowledge. The Agtech ecosystem challenges the veracity of farmers’ knowledge by generating precise agricultural recommendations, promoted as quantifiable, distributable and searchable. Knowledge generated at the farm level by digital technologies is marketed as superior to farmers’ tacit knowledge of their own farming systems, and therefore more reliable (Gardezi and Stock 2021). The algorithmic rationality discussed in a previous section then induces a kind of process that discursively decides on certain legitimate forms of knowledge and excludes others. Farmers would thus become the architects of their own alienation (Gardezi and Stock 2021).
The repeated use of these technologies could lead to a social dependency of farmers who would get something in return – new knowledge – for their data generation (Fraser 2019) (Ingram and Maye 2020). Through the production of trusted knowledge and equipment, digital agricultural systems increasingly mediate a social order in which farmers become dependent on the information and commercial inputs provided by these companies (Stock and Gardezi 2021). And by helping to move farmers away from their plots, or in other words by freeing the farmer from the daily monitoring of his or her fields, the technologies also remove the possibility of acquiring knowledge through observation; knowledge that contributes to experiential learning (Ingram and Maye 2020). A farmer who starts to make decisions based on predictive models thus becomes highly dependent on the reliability of the underlying models, as it may not (yet) be possible to corroborate automated advice with human observations in the field (Visser, Sippel, and Thiemann 2021). And this dependence on new technologies can result in another form of loss of autonomy, if part of a digital tool fails and/or no tool specialist is located near the farm.
In Michael Carolan’s mind, learning to see like an algorithm also relies on those who create the algorithms implementing futures in the image of their assumptions, thus further limiting what (future) farmers see and therefore know. These digital technologies then also indirectly make a decision about the level of knowledge that farmers must have in their quest to practice so-called smart farming (Carolan 2020a)
Authors in anthropology and related fields have long emphasised the power of environmental learning; the experimental, innovative and adaptive farmer being generally considered the norm (Stone and Flachs 2018). Nevertheless, experiential learning becomes even more challenging as digital technologies rapidly evolve and become more complex. Too rapid a pace of development prevents communities from actually generating the necessary and local knowledge of how to use data, digital tools, or agronomic models. Mechanics that are too complex prevent farmers from questioning the recommendations and data generated. In their work, (Stone and Flachs 2018) discuss the sharp increase in the use of spraying in India as a response by farmers to their lack of knowledge about the performance characteristics of their seeds and the effectiveness of their crop protection products. These farmers thus end up continuously (and often desperately) searching for new seeds and crop protection products with which they have no experience and for which there is little accumulated local knowledge
Clemens Driessen and Léonie Heutinck attempt to take a step back from the issue of farmers’ loss of skills (Driessen and Heutinck 2014). To the question “Does a milking robot deskill the farmer?”, the authors explain that it is not possible to answer this question without taking into account the new understanding of the skills of robotic farmers. To the question “Does the robot alienate the farmer?”, the authors invite to open up to other variables: “alienated from what – cows? According to the authors, robotic cows would become to some extent individuals, and would have to be left to their own devices in social processes within the herd, while they would change their character in the process of adaptation to the robot. In response to the question “Is there an alienation from the rhythms of community life?”, the authors answer that the villages in which the farmers live were changing anyway, making the robot a device for regaining the possibility of maintaining community ties. From this perspective of more dynamic practice, deciding to install a milking robot means deciding what kind of farmer to be, what kind of cow behaviour to promote and what kind of human-animal relationship to bring about. (Driessen and Heutinck 2014)
Towards technological lock-in
New precision technologies are also deeply rooted in the prevalence of digital technology systems in society at large. The prevalence and familiarity of digital technologies in society for non-agricultural activities, such as obtaining news and weather forecasts or social media, contribute to the lock-in of farmers’ adoption of digital agriculture. As farmers adopt these new digital-related agricultural technologies, their embeddedness in the industrial agricultural system to which most of these technologies are linked only deepens (Clapp and Ruder 2020) (Stone and Flachs 2018). Michael Carolan returns to this treadmill logic. ‘Early’ adopters of digital technologies make profits for a short period of time due to their lower unit production costs. However, as more farmers adopt the technology, production increases, prices fall and it is no longer possible to make a profit even with lower production costs. Nevertheless, average farmers are forced by falling commodity prices to adopt the technology and reduce their production costs if they are to survive. The ‘laggard’ farmers who do not adopt new technologies are lost in the price squeeze and leave room for their more prosperous neighbours to expand (Carolan 2020b).
(Kuch, Kearnes, and Gulson 2020) explain that Precision farming systems are a form of social control in which farmers, to remain viable, must become increasingly dependent on the commercial inputs provided by these companies. (E. Schnebelin 2021) add that economic actors working towards the industrialisation of agriculture can promote the use of digital technologies. In particular, downstream companies such as cooperatives, traders and seed producers can encourage or even require the use of these technologies through contracts in order to standardise and better control their supply. In this sense, cultivation and seed trade contracts, combined with a specific exchange and advice model, can encourage or even impose the use of these digital technologies (E. Schnebelin 2021). The embedding of commercially oriented technical standards in digital technologies risks locking farmers into asymmetrical relationships in which they may be deprived of the ability to act in certain ways (Higgins et al. 2017).
And as farmers become increasingly dependent on and skilled in using digital technologies to guide their farming decisions, lock-in becomes self-reinforcing as farmers lose the ability to assess trade-offs and make decisions in the absence of digital assistance, as well as the ability to fix their own digital equipment and machinery (Clapp and Ruder 2020). On the latter point, on the one hand, digital systems may indeed be too complex to be used without external support – so farmers do not have the skills to use or repair their technologies. But on the other hand, licensing systems can be put in place to limit the right to repair or modify existing technologies (Lowenberg-DeBoer et al. 2019). Because of their need to access high-tech equipment, farmers, by entering into contractual arrangements with agricultural service providers, may find their freedom to determine how they use these services significantly restricted (Sparrow and Howard 2020)
This temporal nature of the treadmill means that lock-in can be self-reinforcing over time and may eventually crowd out other potential technology systems that could offer more benefits in the long run. (E. Schnebelin 2021) have indeed observed that the use of digital tools for agricultural production facilitates the industrialisation trajectory of farms, which in turn facilitates the use of these technologies. This lock-in may explain why proponents of the digital ecosystem in agriculture have maintained a discourse that focuses on the immediate benefits of adoption in the context of mainstream industrial agriculture (Clapp and Ruder 2020). In cases of lock-in, the cost of not adopting a new technology that fits into a dominant technology system can often be higher than the benefits of actually using that technology, even if there are better ways to solve the problem. In such situations, potential adopters typically make decisions about the costs of adopting (or not adopting) new technologies in the short run, even in cases where the benefits of switching to a different system may be higher in the long run. This dynamic tends to give the lead in debates about the adoption of new technologies to voices that reinforce the dominant technological system while weakening the influence of those promoting alternative systems (Clapp and Ruder 2020).
Data, Data me
Regulations and codes of conduct for agricultural data use
In response to concerns about data privacy and cyber security, the European Union (EU) enacted the 1996 Database Directive to strengthen the protection of databases in all EU Member States. This Directive strengthened the protection of subsequent databases created from the synthesis of various personal and non-personal data. Specifically, this directive prevented the unauthorised use of information contained in databases. As data became more susceptible to privacy breaches, the EU implemented the General Data Protection Regulation (GDPR) in 2018 to complement the Data Protection Directive. The GDPR protects how data aggregators process the personal data of EU residents. Prior to the GDPR, data privacy protection was governed by the different national regulations of each EU Member State. Like the Directive, data protection regulations were not uniformly applied across the EU. While the EU Database Directive covered both personal and non-personal data, the GDPR aimed to regulate personal data only. Therefore, the EU followed the RGPD with the introduction of the Regulation on the Free Movement of Non-Personal Data, with free movement referring to the unrestricted movement of non-personal data within the EU. This regulation was designed to complement the GDPR and stimulate the free flow of all categories of data within the EU market (van der Burg, Wiseman, and Krkeljas 2020)
In different parts of the world, agricultural privacy and security principles and data codes of conduct have been developed. Among others, the following have been launched:
- In 2014, in the United States, the American Farm Bureau’s Agricultural Privacy and Security Principles,
- In 2019, the New Zealand Agricultural Data Code of Practice,
- In 2019, the EU code of conduct on agricultural data sharing,
- In 2020, the Australian Agricultural Data Code (National Farmers Federation 2020).
The main objective of these regulations and codes of practice for sharing agricultural data is to build trust between farmers and companies through a contractual agreement. Although entirely voluntary, these principles and codes encourage companies to provide the farmer with information about what they are doing with the farmer’s data, in order to reassure the farmer and help him or her make an informed choice about sharing (or not) his or her data with companies that develop and provide technology services (van der Burg, Wiseman, and Krkeljas 2020).
In general, data codes aim to guide practices on consent, disclosure and transparency of data sharing, with the strong idea that greater transparency of the conditions of access and use of data will allow farmers to have confidence in the way their data is managed (van der Burg, Wiseman, and Krkeljas 2020). The European Commission itself has reinforced its position on the development of these regulations by stressing that with the codes, EU citizens and service providers will engage in clear and unambiguous practices when transferring data between service providers. As agricultural data is considered non-personal data for the purposes of the Free Movement Regulation, the development of codes has become very interesting for the European agri-food sector. As a result, key EU stakeholders within the agri-food sector were behind the rapid drafting of the EU code for agricultural data sharing (van der Burg, Wiseman, and Krkeljas 2020).
While concerns about the misuse of personal data have been met with a fairly rapid and extensive response from the law, in the form of a myriad of new privacy laws introduced around the world, the very question of whether all or some agricultural data falls within the scope of personal data remains open to debate. While many farmers would argue that all data arising from their farming activities is personal to them (i.e. the size of their farm, the varieties grown, productivity and thus income derived), privacy law does not necessarily take this view. (Wiseman et al. 2019)
The recently adopted regulation on a framework for the free flow of non-personal data in the EU is potentially more important than the impact of the GDPR on the flow of certain agricultural data. This regulation complements the GDPR in that it aims to remove barriers to the free flow of non-personal data within the EU. In turn, the free flow of data will enable the rapid development of the data economy and emerging technologies such as the Internet of Things, artificial intelligence and autonomous systems. There is a general concern that once agricultural data is published and shared, it will fall into the wrong hands. Although not all agricultural data is considered personal data (e.g. machinery data), the fact that much of the data collected on farms is linked to GPS location means that some of the data collected on farms, particularly that which can be linked to reveal arrival and potential profitability, is arguably personal data deserving of protection under privacy law. Article 4 of the Regulation refers specifically to the processing of agricultural data from digital technologies and prohibits their exclusive storage in a geographical area. Localization is prohibited as it can stifle innovation and limit the flow of useful information within global data economies (van der Burg, Wiseman, and Krkeljas 2020). It is important to note that the EU Good Practice Regulation explicitly refers to precision agriculture data and, in doing so, classifies it as non-personal data (van der Burg, Wiseman, and Krkeljas 2020)
While the complexities of agricultural data have been recognised, it should be noted that there is not just one type of data, but that data can often contain a combination of personal and non-personal information (Wiseman et al. 2018). The European Commission has acknowledged that guidance will need to be given on how to deal with datasets that contain both personal and non-personal data in order for businesses to better understand the interaction between the GDPR and the new regulation. However, it is the distinction between operational data that is personal and non-personal that requires further analysis. Interestingly, the proposed Regulation encourages self-regulation through the development of codes of conduct that will allow users to move from one service provider to another without hindrance (similar to the right to mobility under the GDPR) and focuses on vendor lock-in practices in the private sector. (Wiseman et al. 2019)
To date, one of the key initiatives to improve clarity around data ownership and sharing has been the emergence of ag-data codes of practice. The ultimate objectives of ag-data codes of practice are inextricably linked to consent, disclosure, transparency and ultimately trust building. One of the most positive features of the EU Code is that it has raised awareness of a wide range of issues raised by agricultural data sharing by attempting to open up the conversation between companies producing farm technologies and farmers about the terms of their data sharing relationship (Wiseman et al. 2019). However, while these schemes aim to ensure a high level of transparency by encouraging best practice in data management by technology providers as well as educating farmers to seek clarity on the control, access and sharing of their agricultural data by agribusinesses, their success and impact remains to be seen. (Wiseman et al. 2019)
In the future, it will be important to ensure that agricultural data is used for what it was requested for, much like the RGPD is doing now (van der Burg, Wiseman, and Krkeljas 2020). There will also be a lot to look forward to from the European Commission’s “Data Governance Act” on data exchange voted by the end of 2022. The Commission even talks about precision agriculture in the benefits associated with data exchange in agriculture (https://ec.europa.eu/digital-single-market/en/european-data-governance). This law will be the RGPD’s penchant for non-personal data, which is a large part of the agricultural world’s data.
Contract and consent
Informed consent is particularly important in social contexts where there is a power differential between people, for example between experts (those with digital knowledge and skills, such as Agtech companies) and people who are not digital experts (such as farmers). In these relationships, informed consent serves as a mechanism to deal with the vulnerability of the lay person in this power relationship, as it has the role of making coercion, manipulation and deception much more difficult to achieve and much easier to detect. In this way, it helps to establish, maintain and restore relationships of trust. Although there are many models of informed consent, informed consent basically involves a communicative relationship between two parties with unequal knowledge and expertise. One party in this relationship (who has the knowledge and is therefore the information provider) is given the responsibility to give accurate information to the other party about an action, service, procedure, intervention or measure, including the benefits, harms and possible risks involved. On the basis of this information, the other party (the consenting party) decides what action to take or whether to accept the service, procedure, intervention or measure.
Informed consent, like a contract in contractual-individualist approaches to trust, can take informal forms in practice, but also more formal forms that are like signed contracts. This informed consent, or contract, would not have a primary role in the establishment or foundation of trust relationships. It would only be used in situations where people who already trust each other are considering doing something that could potentially damage the trust between them. Any informed consent or contract presupposes a social world in which trust already exists. Consent is only sought for actions that in some way stand out and could potentially undermine that trust. The contract therefore only plays the role of trust maintainer in social contexts in which trust relationships are already there and are supported by norms, obligations, rights and principles that organise social interactions (van der Burg, Wiseman, and Krkeljas 2020). In the context of already established trust relationships, informed consent has a specific function: consent is only sought for actions that somehow stand out and could damage trust, as they do not respect certain principles, codes or obligations that usually apply in society. Informed consent then functions as a (temporary) waiver of obligations, ideals, principles and rights that would normally apply. Individuals who give consent choose to temporarily set aside those social prohibitions and values on which social trust usually depends. An example of such a renunciation of an accepted social prohibition is a situation in which a person asks for consent before taking something from another person’s bag: this action ceases to qualify as stealing because consent was sought first (van der Burg, Wiseman, and Krkeljas 2020).
According to the authors, those who attribute a fundamental role to consent generally do not promote trust but create accountability relationships. In these accountability relationships, professionals use informed consent to justify their actions. Professionals with knowledge and expertise give full information to clients to enable them to give consent, but they do not particularly care about their relationship with clients and therefore do not check whether they have understood this information. Informed consent procedures would then function mainly as a protection for the professional, who is already the most powerful party in the relationship. It leads to situations where the lay person, who is already the most vulnerable party in the relationship, has to digest voluminous and complex information before giving consent. Consent mainly protects the professional, who thus assumes responsibility for the risks associated with the service provided to the lay person. (van der Burg, Wiseman, and Krkeljas 2020)
If we want contracts to foster trust – as described above in relation to informed consent – the authors advocate three important elements. First, the information provided must be understandable to the most vulnerable party in the relationship (such as the farmer) before they give consent. Second, the more powerful parties must take responsibility for providing information. Finally, the information must be tailored to the information needs of the user, even when the data is reused over a long period of time. According to the authors, corporate data contracts should make a number of information transparent and understandable:
- the most important conditions
- the purpose of the data collection, sharing and processing
- the rights and obligations of the parties with regard to the data, the rules and processes for sharing the data, the security of the data and the legal framework in which the data is kept and in which back-up copies are stored
- the relevant software or application and the storage of information and use of agricultural data
- mechanisms for verifying the authorship of the data – transparent mechanisms for adding new and/or future uses.
While it seems good to ask for informed consent and give farmers the opportunity to make a choice, it is also important to be clear about the limits of this consent. While it is the responsibility of companies to take responsibility for informing farmers about what they are doing with their data, care should be taken in choosing how much information can reasonably be provided and understood by farmers about the re-use of data. If farmers are contacted regularly and asked to read piles of information to judge whether each new use of their data is acceptable or not, farmers can be expected to be overburdened. Digitising the information provision process (which is sometimes suggested as a solution to this problem) will not really solve these problems, as it is impossible to see whether the information provided meets the information needs of farmers in different situations. One way to limit this information is to offer farmers a menu of choices. For example, by giving them a choice between different possible users of their data (e.g. companies, governments, researchers), or between different types of purposes for which their data could be used (e.g. for research by publicly funded researchers, to inform policy, to inform banks and insurance companies, to develop innovative applications by companies, etc.). Alternatively, if it is difficult for farmers to make such choices, farmers could choose to store their data in a larger repository, managed by a group of farmer representatives, specialised in data governance issues, and which could establish general principles (e.g. fiduciary or public interest principles) governing decision-making about data re-use. In this way, individual farmers would delegate their decision to these representatives, who would set policy for the data repository and develop guidelines for how the data can be used and to whom data users can apply for access. However, discussion of these alternatives has reportedly not yet begun (van der Burg, Wiseman, and Krkeljas 2020).
The EU has provided a ‘checklist’ of questions to help farmers check whether their data is being used correctly. While the questions in the EU Code checklist may help the farmer to be inquisitive, (van der Burg, Wiseman, and Krkeljas 2020) find that these questions do not provide guidance on how to determine whether or not the contractual arrangement proposed by a service provider or agribusiness is acceptable. The checklist seems to place an unfair burden on farmers to research the terms of use of their agri-food partners. For example, the checklist asks a series of questions such as “Is there an agreement in place?”, “What obligations are in place?”, “Will my data be used for any purpose other than to provide a service to me, the originator of the data (i.e. the farmer)?”, “Will I be informed of security breaches?”, or “Can I get my dataset back from the system in a usable format? Given the way the questions are formulated, most of the questions in the checklist are designed to encourage farmers to find out for themselves. (van der Burg, Wiseman, and Krkeljas 2020)
In the field of the Agtech ecosystem, relationships of trust are not necessarily already in place. In many contexts, digital technologies will bring about a fairly fundamental change in the social network around farms (discussed above). Farmers have traditionally had trusting relationships with many actors such as advisors, cooperatives, farmers’ associations and input suppliers. In these relationships, farmers knew what to expect. With the introduction of software industry actors, farmers are invited to engage in new and often more abstract relationships than they are used to. Contractual agreements between human actors can only play a limited role in fostering relationships of trust that remain largely virtual (van der Burg, Wiseman, and Krkeljas 2020). While start-ups and relatively small technology companies often engage with farmers in real life through face-to-face collaborative arrangements, it is expected that these relationships will become increasingly digitised and therefore abstract as these companies and the number of their customers increase. Contractual agreements with large technology companies are unlikely to be sealed with a traditional handshake and will rarely be face-to-face. According to the authors, the increase in the number of people sharing data in the network, as well as the increasing geographical distance between them, will lead to the development of tools that digitise the informed consent process. However, the theory of informed consent gives significant reason to doubt that these efforts will by themselves lead to trust. This digitisation can also serve as a protection for the companies that make the digital technologies, as they can always refer to the farmer’s consent to justify what they do with the data. Trust in digital technology is more likely to be built if it is introduced in contexts where relationships and collaborations are already established, or if innovators invest a lot of time and effort in fostering trusted social relationships before introducing their technologies. These relationships will require ongoing attention and maintenance. If these relationships are non-existent at the outset, it is questionable whether a contract alone can create trust. In more abstract relationships, it may make sense to broaden the code of conduct and give the partners in this unequal power relationship a richer range of topics for reflection and discussion, in order to develop broader conditions for their mutual engagement.
Many data licences involve the use of a ‘click-wrap’ agreement (where clicking on an ‘I agree’ icon signifies consent to the terms of a software licence), and this is often how farmers enter into and accept data licences for agricultural technology. Data licences embedded in digital agricultural technologies are usually complex and standardised licence agreements, generally non-negotiable and presented on a ‘take it or leave it’ basis when the technology is adopted. The terms of use of the technology are therefore agreed at the time of downloading an application or starting up a machine. Often a data licence also provides links to other policy documents, such as the privacy policy of the agricultural technology provider. In some cases, it is the privacy policy, rather than the terms of use of the data licence, that determines who can access the data generated under the agreement (Wiseman et al. 2019). Add to this the fact that often the licensing agreements that govern the sharing and use of their agricultural data will be governed by the law of the country where that company is registered, creating uncertainty about the level of protection offered to farmers. (Wiseman et al. 2019)
The GDPR empowers data contributors with new rights of access to data and introduces the broader notion of consent (which must be freely given and be explicit about what the data is requested for, with a clear demonstration of agreement. Where consent has been obtained for the processing of personal data, the controller must be able to demonstrate that the consent is freely given, specific and informed. Consent shall be purpose-limited, i.e. it shall only allow processing for explicitly specified purposes. The consent obtained must be intelligible, easily accessible and written in clear and simple language. This is to eliminate any confusion as to whether or not consent has been given, and whether it can be implied by a particular action (or inaction). Data subjects must also have the right to revoke their consent at any time and it must be as easy to withdraw consent as to give it. The expanded notion of consent is complemented by the right of individuals to transfer their data from one controller to another (right to portability) and to have their data deleted (right to be forgotten). In summary, the GDPR imposes new responsibilities on data controllers and processors to be more transparent in their dealings with individuals when their data is collected and managed. (Wiseman et al. 2019). These changes on the interest for contributors show the shift of interest away from data aggregators, and much more towards contributors. (Wiseman, 2018). One can only hope that these changes will also take place for non-personal data, and all the more so for agricultural data.
Control and ownership of data
The issues of mistrust around data in the agricultural digital domain are sometimes understood as a privacy issue, as farms are private businesses and therefore farmers should have control over data that contains information about their farms. These issues are also sometimes framed in terms of data ownership – with farmers asking who owns the data and therefore who has the right to monetise and profit from the value of the data. (van der Burg, Wiseman, and Krkeljas 2020).
Digital technologies make the ownership of agricultural data quite blurred. Often, farmers assume that they own the primary data as they collect it on their farms, while intermediaries create the algorithms that combine and interpret the data from many farms and use it to generate useful agricultural recommendations. These intermediaries are considered the owners of the calculated data. When thinking about data, it is important to note that, while many people think that data attracts a form of ownership that is generally covered by the intellectual property regime, the reality is that ownership rights in data will only arise if copyright law can protect the data (or if the database is protected under the EU Database Directive). Not all data or collections of data will benefit from copyright protection. Despite this, there is still much debate about the ownership of agricultural data. Copyright law can protect data tables or data compilations where the selection and arrangement of the data is the result of human labour, skill or effort. However, it is important to note that it has long been recognised that raw data, information or simple facts are not copyrightable subject matter (Wiseman et al. 2019). At present, it is easier to protect inventors of technologies and databases on the basis of current laws, than to protect the property rights of farmers. (van der Burg, Bogaardt, and Wolfert 2019)
In Australia, where the researchers work, (Wiseman, 2018) explain that the two areas of law that people primarily refer to on data ownership are privacy law and intellectual property law; specifically copyright law. Although farmers consider their agricultural data to be personal data, privacy law in Australia does not see it the same way. There, the privacy law does not give ownership of the data to those who collect it or those on whom it is collected. This law regulates how personal data is collected, used, and disclosed. Personal data is information or an opinion about an identifiable, or reasonably identifiable, person, whether or not the information or opinion is true, or whether or not the information or opinion is collected in a material form (Wiseman, 2018)
On the contrary, non-personal information, such as business information and agricultural data are managed by the law of contracts. The distinction between personal and non-personal data is very important for agriculture industry and their stakeholders, because the privacy law in Australia does not extend to non-personal data. Since privacy law currently does not provide any protection for how agricultural data is collected, stored or exchanged, it must be brought under the umbrella of intellectual property, particularly through copyright law. Copyright ownership of aggregated data or databases gives those who own it the right to exploit and commercialize the data. While copyright law does not protect information, ideas or simple facts, the collection of data that is the result of an original contribution by the data aggregator may be protected by copyright law. Determining what is protectable by copyright law is complicated. But it is even more important to address it because the law is very clear that the contributor of the data is not necessarily the one who owns the data. Applying that to agricultural data, it’s clear that the farmer, as a data contributor, will not necessarily have the rights to the data produced. (Wiseman, 2018)
A discussion of who owns the data, however, raises more questions than it answers. It is important to note that even when data is protected by copyright law, copyright ownership can often be changed by contract. For example, when a contractual arrangement that addresses ownership of collected data is entered into between a farmer and a third party (such as a consultant, service provider, or agribusiness), it is the ownership provisions in the data contract that will often trump the copyright law position. Given that copyright law allows contracts to override its ownership provisions, it is useful to take a closer look at the nature of the many and varied relationships in agricultural data and data contracts between data providers (i.e., farmers) and agricultural digital technology providers (i.e., data aggregators). It is these contracts, rather than the laws that were discussed above, that will govern agricultural data relationships, as these contracts are the primary means of controlling, managing, and sharing agricultural data (Wiseman et al. 2019).
In addition, some authors point out that the use of the term “ownership” is strange and unusual with respect to data, because owned physical objects can be used by only one person at a time, which is not the case for data. The use of data by one person or entity does not prevent others from using it as well. According to some authors, patents, intellectual property rights, and copyright laws would not provide a good starting point for identifying what farmers’ property rights mean and why they should be protected. This is because patents only cover the creation or discovery of a process or machine, but data is not invented by farmers and is not a new process or machine in and of itself. Agricultural data does not qualify as an original work of authorship that could fall under copyright. However, it would be possible to consider agricultural data as trade secrets and use them to attribute ownership to farmers (Wiseman et al. 2019).
For some authors, the language around data ownership distracts everyone from more important issues such as the collection, control, and access to that data. In simpler terms, it is possible to own data-to own it-but actually have little control over who and how that data is used. (Wiseman et al. 2019). A far more interesting and relevant question, then, is what those who collect agricultural data do with it once they have retrieved it (Jakku et al. 2019). There is indeed a concern that proprietary platform approaches, or big business in the sector, by controlling the digital data collected, may further concentrate ownership and control of food systems, production, trade and consumption, and threaten food sovereignty. (Klerkx and Rose 2020) (Klerkx, Jakku, and Labarthe 2019) (van der Burg, Bogaardt, and Wolfert 2019). When companies are the main actors controlling the direction of technology development, they tend to make decisions based on their own interests, which can strengthen their power (Clapp and Ruder 2020). This is what we defined above as the Big Data divide (see section on multi-speed agriculture), or what others define as the digital data divide-a divide between those who provide the data and those who control, aggregate, and share it (Wiseman et al. 2019). Agribusinesses can effectively use agricultural data to influence or inform decisions in the stock market, profile farmers, and sell these profiles to third parties-input suppliers, researchers, governments, NGOs, banks, or even insurance companies (van der Burg, Wiseman, and Krkeljas 2020). Agri-businesses or structures that retrieve crop data can use it to play on international futures markets (Duncan et al. 2021). When the software that runs a farmer’s seed drill or the seeds planted are subject to intellectual property protections, it is questionable whether the farmer really still owns the equipment he or she would have purchased. The farmer is essentially getting a license for essential parts, which he sometimes cannot even repair himself. Farmers clearly lack knowledge about the extensive rights granted to data aggregators in the contracting relationships they find themselves in. This leads to producers thinking about the abuse of power these data aggregators may have over them regarding their agricultural data. (Wiseman, 2018)
Another general concern of farmers is that their data is regularly shared or disclosed to third parties, making it difficult for them to know who knows the details of their business ventures (Wiseman et al. 2019). Theoretically, the right to use the data can be granted to many different stakeholders. Nevertheless, because of the non-physical nature of data, it is relatively difficult to track who is allowed to share the data and what data is shared (EU, Code of Conduct, 2018). In her panel, Emily Duncan observed that farmers surveyed were more willing to share their data with other farmers and research institutions, and less willing to share it with technology and service providers (Duncan 2018). Farmers generally seemed to have a positive attitude toward data sharing as long as they benefited themselves, but some farmers found it problematic that other actors or companies benefited from the data they generated, without clear benefits to themselves. (Duncan 2018). Farmers’ discomfort with data sharing is conceptualized as a response to the changing power relations that digital technologies (and the companies that make them) affect in the social network surrounding farms, raising questions about the fair and equitable distribution of benefits and responsibilities within that network (van der Burg, Wiseman, and Krkeljas 2020). This raises the larger question of what data users are likely or willing to share, and with whom, or which structures have the right to access the data and which do not. Some authors in the humanities and social sciences have, for example, distinguished between different data streams. “Localized” data are generated on a farm and used on the farm, “imported” data are generated off the farm for use on the farm, “exported” data are generated on the farm for use off the farm, and “ancillary” data are generated and collected on and off the farm, primarily for use off the farm (van der Burg, Bogaardt, and Wolfert 2019).
It should be noted, however, that the ability to reuse data can open up a whole host of questions about what information must be given before entering into a contract. It is consistent with the spirit of informed consent to go back to the data originators to ask for their consent regarding the reuse of the data. However, it is also questionable whether it is practically possible and desirable to do so for each new use of the data. (van der Burg, Wiseman, and Krkeljas 2020).
Digital technologies in agriculture differ radically from conventional technologies, such as the farm machine or tractor, in that they are constantly evolving and are characterized by ever increasing size and complexity. The idea that farmers, although contributing to the development of the tool, do not have full access to it, seems paradoxical if we refer to conventional technologies (Lioutas et al. 2019). Given that farmers are providing their data to third-party technologies and platforms, it seems logical to assign them the right to demand justification for what is being done with that data. Questions like, “How are they interpreted?” or “Why are these algorithms used and not others?” seem natural enough to ask and should be easily answered. But it makes just as much sense to ask how the data, and how it is interpreted, can contribute to common goals, and what are useful ways that partners in a data-sharing network can cooperate to achieve those goals (van der Burg, Bogaardt, and Wolfert 2019)
Even though our lives are tied up with computers, the meaning of the data we produce can seem like a marginal dynamic in a violent and oppressive world. Although the offer of free software services is too good to be true, as an offer it is still good, at least for many people. Yet as long as users of contemporary technological services participate in and remain dispossessed of the value emerging from the data our daily activities generate, we not only risk new forms of corporate control of society, we also acquiesce, according to Alistair Fraser, to a loss of any notion of sovereignty (Fraser 2019).
While the codes and regulations produced have tried to build confidence in digital tools and services – and have raised some issues and problems around data use – they have not provided much clarity around the legal position of ownership of farm data from farms. The law and understanding around ownership of control and access to agricultural data is still uncertain. (Wiseman, 2018)
The fact that many distribution and production companies are acquiring digital technologies shows that it is very important to look at how agricultural data can be, and is currently being used. We need to look at contract relationships, because data licenses set the rights to the type of data collected on farms, and how it is controlled, exchanged and accessed. People are concerned not only with the rights of third parties to access data, but also that farmers – the contributors – can also access and move the data they have contributed to (Wiseman, 2018)
Who will get the lion’s share?
Farmers are becoming important data holders. Data is in fact, at least for some industry players, the new cash crop, because when aggregated, it has value for seed and chemical companies, agronomists, cooperatives, farm insurance providers, and equipment manufacturers. Individual farm-level data have limited value to the individual farm, but aggregated data pooled across many farms have the potential to reveal undiscovered patterns and insights that cannot be determined by simply looking at individual farm data (Zhang et al. 2021). The fact that raw data has no intrinsic value because it is not aggregated or put into context is an argument regularly made by players in the Agtech ecosystem. One might also ask whether this argument is not also a way of collecting more and more data while at the same time giving oneself a clear conscience?
(Zhang et al. 2021) suggest that farmers are able to appreciate the potential value of the agricultural digital dataset, but would be concerned about who would benefit and whether the use of their data might put them at a disadvantage to companies with whom they could share their data. And (Jakku et al. 2019) added that for the majority of farmers on their panel, the benefits of these digital technologies are likely to be realized more in the long term or downstream, and that in many cases these benefits appear uncertain. Realizing the revolutionary value promised by big data technologies may thus depend on the willingness of farmers to share their agricultural production data. (Zhang et al. 2021)
In a way, since many of the services that generate data today are free, there is a tacit understanding that the free use occurs precisely because the data is collected and eventually sold. In this view, users are simply involved in a simple form of exchange: they can use the latest software and keep in touch with other people in exchange for some degree of privacy for companies that keep claiming to be innocent – or, if not, at least committed to using customer information to improve their services or to provide better or more relevant advertising. Alistair Fraser explains that with this data collection, there would not only be a dispossession of control over that data (Fraser 2019). Rather, data hoarding would be a crucial step in a larger, persistent, and ubiquitous process, whereby the activities of all users in networks of social relationships generate data with a possible exchange value greater than the sum of their individual parts, without ever receiving their share of that value. Taking control of data can – but will not necessarily (as the many stories of failed startups demonstrate) – generate profits (Fraser 2019).
Perhaps the central question in the discussion of data access is not so much who owns the data, but rather who owns the value of the data (Lioutas et al. 2019). The power imbalance observed within a community might thus be more about unequal access not to big data but to the value that emerges from it. Whether framed as a matter of surveillance capitalism or data colonialism, an important dynamic of digital life concerns the maldistribution of opportunities to convert data curation into profit. The asymmetries of digital life mean that there are companies in the industry that are able to make the most profit from this data (Fraser 2021).
Emily Duncan explains that as precision farming technologies continue to become more detailed and specialized, farmers feel that those selling precision farming technologies are benefiting much more than they are (Duncan 2018). Indeed, underlying this is the idea that this complexity provides opportunities for companies in the sector, namely selling technologies but also providing data interpretation services. Even if agricultural data is presented as open, farmers are not necessarily equipped to perform the right kind of analysis that can add value to their data. A series of points on a graph is one thing, but knowing how to analyze or create new models (and having the computing power and time to do so) is another.
A key task for researchers is to clarify what “value” means to farmers and other community members, and then to examine how power dynamics affect access to that value. A first step toward this end should be identifying the actors who jointly produce big data and the value that is extracted from it (Lioutas et al. 2019). The constant flow of data through digital technologies and the complex nature of the interactions between the actors who share it complicate the translation of data into value.
The importance of trust relationships
For (Gardezi and Stock 2021), the conceptualization of trust in the social sciences can be classified into two broad dimensions: strategic trust and moralistic trust. Strategic trust is knowledge-based trust and depends on past experiences and the accuracy of shared knowledge or tools. Moralistic trust, on the other hand, does not depend on rational expectations, personal experiences, or the perceived trustworthiness of others (whether people or objects), but depends on the moral values of their culture. Moralistic trust is inherited through cultural transmission and becomes a form of rational action through values, where actors come to believe that most people share their core moral values. According to (Gardezi and Stock 2021), studies and reports examining farmers’ engagement with digital technologies have primarily conceptualized farmers’ trust in these technologies as being based on strategic trust, where farmers consciously weigh the risks and benefits of using technological systems and then decide to adopt them accordingly. The findings of their work would reveal that contrary to this strategic trust view, farmers would not trust these technologies through a strategic assessment of its risks and benefits to their farms, but through the cultural transmission of moral values championed as superior by companies in the Agtech ecosystem. The authors call for existing and future guidelines for ethical digital technologies to seek to examine the value of these technologies through the lens of moralist trust. For example, future research could highlight the intentional and unintentional impacts of the algorithmic rationality of digital technologies that creates a sense of trust not in the efficacy of these technologies but in the moral values of the community of those who use these technologies (Gardezi and Stock 2021).
The use and sharing of data is not neutral (Carolan 2017). This means that building trust is a subjective exercise that requires platforms and data collectors to acknowledge their own biases and limitations. Another crucial aspect of building trust among farmers is the recognition that just because data is accessible does not mean it is ethical to retrieve and use it. Acting ethically with data requires accountability, even when privacy is not an issue (Zhang et al. 2021). A farmer interviewed by (Jakku et al. 2019) provides an interesting addition in that the industry was reportedly particularly bad at explaining why access to agricultural data was important to them. For this farmer interviewed, this is strange since since since the industry is not clear about their intimate reasons for collecting and using the data, there would be no reason for him to trust these industrialists. One would then have to take industry’s word for it (Jakku et al. 2019). The expected loss of farmer autonomy, as well as the vulnerability to potential secondary use of data by algorithm experts for their own benefit, would be much less frightening, however, if data providers and analysts were transparent about their choices and negotiated them with farmers (van der Burg, Bogaardt, and Wolfert 2019).
For (Wiseman et al. 2019), the willingness to share data may be intrinsically linked to values of trust toward those with whom the data are shared, with trust defined as a willingness to accept some risk and vulnerability toward others, and the reasonable expectation regarding the actions and intentions of others. For the authors, a deeper understanding of the legal framework around digital technologies, particularly the terms and conditions of agricultural data licenses, and in the fact that service and technology providers keep data confidential and do not share it with third parties, can increase farmers’ trust in data sharing (Jakku et al. 2019) (Wiseman et al. 2019). Agricultural technology providers, many of whom now come from industries outside the agricultural community, have introduced lengthy and complex software licensing agreements that govern how farmers’ data will be collected, managed, and shared with their agricultural digital technology providers. The scope and breadth of terms of software licenses embedded in agricultural equipment (e.g., sensors, robotics, drones, tractors, and farm machinery) are rarely discussed or even mentioned at the time of sale. (Wiseman et al. 2019) notes that many farmers were surprised by the fact that simply turning on their machines or downloading the technology meant that they had agreed to a wide range of terms and conditions that regulate access to and use of data generated on their farms. Indeed, there is a concern about cognitive dissonance, i.e., a disconnect between what the contracts say and what the farmers think they say. That said, the authors caution against a one-size-fits-all approach to agricultural data sharing. A one-size-fits-all approach to data sharing in the agricultural context will be inappropriate at best, and at worst, detrimental to those providing the data (Wiseman et al. 2019).
Rethinking the adoption and evaluation of digital tools in agriculture
Tracking the process of digital tool adoption still lacking
Tracking the adoption of emerging technologies is fundamental to understanding and stepping back from ongoing dynamics. We can detect major trends, perceive weak signals, and highlight factors that facilitate or limit a given adoption. Adoption studies in Agtech are well underway. At the time of writing this blog article, many technical and scientific studies have been published in the literature but very little work has been done to try to synthesize and harmonize this knowledge. We can nevertheless cite a recent, fairly substantial work of aggregating scientific knowledge (Lowenberg-Deboer and Erickson 2019). In their synthesis, these authors point out that, from the confusing mass of data collected from surveys that used several different sampling methods and were analyzed in various ways, a picture of rapid adoption of geo-positioning and related technologies emerges, and slower adoption of all intra-parcel modulation technologies. Adoption of data collection technologies such as yield and soil mapping and remote sensing, was also relatively slow.
In response to this lack of harmonized knowledge, we recently concatenated the results of numerous adoption reports from both technical and scientific literature into a dynamic and interactive interface on our Digital Tools for Agriculture directory. This synthesis work has allowed us to identify some strong limitations. First of all, it is clear that despite the large number of studies, it is not easy to identify trends. This is mainly due to the fact that all these studies approach the adoption of digital tools in different ways. The target audiences, the study framework, the adoption characteristics studied, the technologies followed; almost nothing is harmonized (Tey and Brindal, 2021). This leaves us with studies that are very punctual in time and space, and finding links between these studies remains a rather difficult task and subject to interpretation. (Lowenberg-Deboer and Erickson 2019) even call for standardization of adoption studies. Comparable data on the adoption of precision agriculture tools across major agricultural countries would make it much easier to diagnose key issues and constraints. For most other technologies, the Food and Agriculture Organization of the United Nations (FAO) provides guidelines on how to collect data through the World Census of Agriculture (WCA) program. (Lowenberg-Deboer and Erickson 2019) question whether it would be so much the WCA to add precision agriculture technologies.
A second important limitation is that the main adoption studies focus almost exclusively on the adoption phenomenon in a rather narrow way, and by considering the adoption factors studied independently. It is now well recognized that a farmer’s commitment to a new technology is not simply a technical issue. Rather, technology adoption involves an active consideration of values, beliefs, identities, goals, and social networks. Knowing this social context also requires knowledge of the institutions that interact with farmers and farming communities, including technology developers and producers, industry organizations, brokers and marketers, supply chain partners, supermarkets, and consumers (Fleming et al. 2019). The farmer may have varying degrees of risk aversion (to using digital technologies) but the financial structure of the business may impose an additional source of risk – financial risk, for example. Similarly, components such as innovation, adoption, linkage, and assimilation interact to the extent that complex technologies (e.g., intra-parcel modulation) may require expertise beyond that of the operations team (Pathak, Brown, and Best 2019).
Focusing only on overly narrow adoption factors such as age, farm size, or even the cost of digital tools does not capture the full nuance of trends that influence digital technology adoption. An example on the age of farmers. Many studies point to the age of the farmer as a barrier to adoption and argue that certain technologies would be harder for older farmers to learn. Yet technologies have sometimes been found to help older farmers stay on the farm longer through environmental improvements. (Vik et al. 2019) further add that for most farmers, knowing that there is a successor who wants to continue production contributes positively to their quality of life and job satisfaction. Because farms are businesses, many studies of digital technology adoption have assumed, explicitly or implicitly, that adoption of these technologies would be strongly influenced by profitability, as well as risk, resource availability, and other economic benefits, thereby missing the much greater complexity behind digital tool adoption. One of the assumptions often made implicitly is that innovations are economically beneficial and that farmers are economically rational actors (Bryant and Higgins 2021). Criticisms are emerging, however, of the very linear way of viewing the adoption of digital tools (Bryant and Higgins 2021).
Rather than uniform technology adoption and impacts, it is then fundamental to realize the importance of context and diversity in technology adoption and its associated impacts (Schewe and Stuart 2015). On the use of robots, for example, (Lowenberg-DeBoer et al. 2019) propose to think about determining the optimal robot size for a given type of farm and set of tasks, thereby considering that each farm is different. (Vik et al. 2019). Given the complexity and multidimensional nature of adoption processes, it is not surprising that studies on the adoption of precision agriculture technologies that examine only some of the many interacting factors have tended to produce variable and often contradictory results (Pathak, Brown, and Best 2019). Indeed, the latter authors call for a new literature review to synthesize all of the published literature on the adoption process of precision farming technologies within an innovation diffusion theoretical framework (Pathak, Brown, and Best 2019).
More attention to other drivers of adoption and the changing nature of relationships in the agricultural industry following the use of digital agricultural technologies is needed to understand the broader scope of adoption trends (Duncan 2018). The adoption of digital tools is often more than a “plug and play” process for farmers. Successful use of these new tools depends on many aspects such as matching the technology to the use, adapting the tool to the farm, learning data-driven decision making, and social learning within the farmer’s practice network (Callum R. Eastwood and Renwick 2020). Technology adoption is a process that takes place over time. Factors that explain one step in this process may not explain all steps in the process. Digital technologies are experienced and used differently not only by different actors, but also by the same actor at different times (Lioutas et al. 2019).
The role of farmers using digital technologies should not be underestimated or ignored. In the current still very techno-centric mindset, the focus is on creating technologies, rather than developing a system involving learning and knowledge development among users (C R Eastwood, Chapman, and Paine 2009). The development of relevant digital technologies relies on the recognition of farmers as co-developers of knowledge about digital tools, and that successful adoption of digital tools requires a systems approach rather than a focus on the technological tools themselves. Farmers need to be recognized as experimenters and innovators, and the status of “farmer researcher” advocated by several actors may be particularly relevant here.
Not adopting a technology is not necessarily a sign of resistance to change, or of a slower adoption process by farmers who are lagging behind. It may be a consistent choice for farmers that fits their practices and goals, towards a different technology trajectory. The adoption and use of a technology may also not be the result of individual pioneering behavior, but the result of a production model interacting with a socio-economic system that encourages, or even imposes, these technologies (E. Schnebelin 2021).
Who are digital tools in agriculture really for?
If the rhetoric around digital technologies emphasizes the tools themselves over farm management, the new over the old, performance over farm stability, innovation over adoption, intended uses over actual contexts, and the evolution of farmers into agricultural technologists, then who is agri-technology for (Duncan et al. 2021)? In their discourse analysis, these authors emphasize that industry experts and tool providers define appropriate technology use, not farmers. If the Agtech ecosystem reinvents itself to attract investment to new technologies that promise revolutionary advances in agriculture, while neglecting how farmers are embedded in economic structures that already limit their choices, (Duncan et al. 2021) call for reflection on how digital tools align with corporate financial and technological interests. Emily Duncan and her co-authors suggest that by positioning farmers as using digital tools in inappropriate or unexpected ways, the context of precision agriculture use does not actually match farmers’ needs. The stigma is indeed generally placed on the farmer (Bryant and Higgins 2021). The lack of adoption of digital tools is explained by a lack of willingness on the part of producers to change their attitudes, behaviors and practices. Ultimately, there is little questioning of technologists and digital tool providers. By not accepting that the tools developed may not be useful to farmers, one can only rethink one of the assertions of the work of Emily Duncan and her team: Who are digital technologies in agriculture really for?
The question of who adopts is also open. If digital tools were aimed at farmers, limited rates of farmer adoption should be at the forefront of concern. Yet this is clearly not what (Duncan et al. 2021) observed in their analyses. Consultants, traders, suppliers, and other advisors might be the most appropriate category of adopters. This assertion that those involved in the work around the farmer might be the most appropriate adopters of precision agriculture technologies suggests that individual farmers are wary of the risk of investing in a capital-intensive technology without a clear return on investment. By positioning limited adoption rates as a challenge to be overcome by turning to other types of users, or by directly implicating farmers as not understanding the benefits of these technologies, the authors question whether these tools would instead be a big benefit to the vendors and various stakeholders promoting them (Duncan et al. 2021). This is reinforced by the positioning of the challenge of using precision agriculture as a technical issue of data and equipment standardization to be addressed by manufacturers, rather than as a legislative or governance issue of allowing farmers to make their technologies work for them (Duncan et al. 2021).
Somewhat surprisingly, the Agtech ecosystem is composed of a host of startups with few prior ties to the agricultural world (Duncan et al. 2021). And even internally, in the composition of employees, one may be surprised at the small component of working agronomists. The Agtech ecosystem facilitates the entry of technology companies into the agricultural field. Several authors tend to see the technologies in the Agtech ecosystem as experimental technologies for the technology and finance industries. Indeed, agricultural land would be considered a model because of its heterogeneity, variability, and ability to generate real big data, and thus could be seen as a nice playground for further developing research on artificial intelligence tools (Duncan et al. 2021). While this is an interesting view to ponder, I would tend to think that many companies in the Agtech ecosystem truly believe that they are helping agricultural industries by developing these technologies. Nevertheless, I think that these actors, often coming from an engineering background and having followed a long educational path, have a real need to justify their education and skills, something they seem to find by participating in creating the innovations and building something new for the agricultural sector. For these companies, the orientation of their technologies towards the agricultural sector would also respond to a deeper need for research and a search for meaning in their daily work.
Agtech: A very large and abundant ecosystem
The ecosystem of digital tools in agriculture is extremely abundant. Both a strength and a weakness, this large ecosystem offers a wide variety of tools and services, but makes it particularly complicated to understand. How do you find your way through this maze of tools? How do you choose a tool or service when more than a dozen players offer very similar solutions? As a consultant or technician, how can I synthesize and popularize such a large ecosystem for farmers who might be in demand? In my opinion, this complexity is largely responsible for the lack of interest that digital tools may have for agricultural professionals. In mid-2020, I had tried to aggregate and synthesize knowledge through very visual infographics. I showed a simplified, very visual overview, but I quickly realized that the infographics were not very informative in content. There was no link between the tools, very little explanation about the tools as such, except maybe a very macroscopic classification of the Agtech sector. After a second attempt – trying to make these infographics interactive in a tree format – had also proved unsuccessful in sharing knowledge of the Agtech ecosystem, we then turned to an online directory, in the form of an e-commerce site (we do not sell tools or do affiliation), which would provide sufficient architecture and infrastructure to encapsulate and embed all the digital tools and services of the field. The Digital Tools in Agriculture platform that we launched in mid-2021 :
- Was developed with open-source technology,
- is free and open access,
- collaborative and participatory,
- and sustainable over time.
Each user of the platform can thus have access, through a set of business and technological filters, to a large reference of digital tools and services. By connecting to the platform, users can become part of a community, giving their opinion and comments on the tools presented, adding a digital tool to the database, or proposing modifications to tools already listed.
Towards a better evaluation of digital tools
Today, science must prove that digitization does indeed have positive effects on society. The contribution of digitization to the fight against food insecurity remains a hope rather than a given. Moreover, the assumption that the food problem can be solved by simply increasing food production is rather mild to suggest that investments in digital technologies will have the desired results (Lioutas, Charatsari, and De Rosa 2021). The lack of relevant data does not allow policymakers to assess the magnitude of the various negative impacts expected from digitalization. As a result, there are many empty spaces regarding the collateral effects that the penetration of digital technologies in agri-food systems may have.
The Agtech ecosystem model still remains too focused on a presumption of innovation and market growth, without articulation of how this will occur. (Duncan et al. 2021) (van der Burg, Bogaardt, and Wolfert 2019). Today, a growing body of academic work confirms the positive potential of digital tools for agriculture. Nevertheless, technology research traditionally focuses on the technical aspects of technology implementation, without considering its potential social externalities (Lioutas, Charatsari, and De Rosa 2021). Yet digitization is not isolated from other societal, political, and behavioral changes around agriculture. More specifically, agricultural digitalization, by transforming actors and sectors, affects and is affected by these changes. This intercorrelation clouds our ability to predict the future trajectories of digital tools applied to agriculture. Robust methods are therefore needed to assess the long-term benefits of digital technologies for agri-food systems under conditions of complexity (Lioutas, Charatsari, and De Rosa 2021). We still lack clear measures of success. (Whitfield, Challinor, and Rees 2018) explain this complexity as follows: “can an increase in yield that contributes little to greenhouse gas mitigation, or an overall reduction in emissions that reduces water use efficiency be considered climate smart?” A critical question is whether these digital tools can actually drive agriculture toward a sustainable future. Despite the considerable amount of literature addressing this question, our current understanding of how to use digital tools to achieve sustainability in agri-food production is still in its infancy (Lioutas et al. 2019).
On digital technologies more generally, (Vinuesa et al. 2020) discuss the fact that although many studies suggest that artificial intelligence can potentially serve as a catalyst for many sustainable development goals and indicators, a significant fraction of these studies have been conducted in controlled laboratory environments, based on limited datasets or using prototypes. Extrapolation of experimental results to societal-scale impacts is quite precarious, as considerable differences can exist between experimental and real-world settings. (Lioutas, Charatsari, and De Rosa 2021). James Lowenberg-DeBoer gives a similar example on agricultural robotics. With autonomous equipment, the motivation for large equipment is much lower and optimal farm size is likely affected. Various research methodologies have been used to study economies of scale and optimal farm size, but given the lack of real-world experience on farms with autonomous equipment, early studies are likely to use mathematical programming models for the entire farm (Lowenberg-DeBoer et al. 2019).
(Vinuesa et al. 2020) add that although artificial intelligence seems to them to be more of an enabler than an inhibitor of sustainable development goals, there are at least two important aspects to consider. First, self-interest can be expected to drive the research community and the artificial intelligence industry to publish positive results. Second, uncovering the detrimental aspects of artificial intelligence may require longer-term studies, and at present we do not have many established evaluation methodologies available to do so. Even if the solutions are mathematically optimal (given a certain range of selected parameters), more research would be needed to assess the long-term impact of these algorithms on equity and justice, precisely because of the unknown factors that may come into play (Vinuesa et al. 2020).
Digitization may also challenge the way we analyze literature and undertake experiments. The volume of knowledge generated is now impossible for scientists in one field to keep up with, leading to a narrower disciplinary focus, which in turn risks missing potential solutions outside of one discipline. Digital technology is developing faster than scientific validation, compromising the ability of science to advise industry on the validity or utility of these technologies in the value chain (Shepherd et al. 2018).
Several authors emphasize the need for more consequential studies on the cost-effectiveness of digital tools-especially for small and medium-sized farms-to help farmers understand the true return on investment of PA technologies (Duncan 2018) (Vasconez, Kantor, and Auat Cheein 2019). This type of analysis can help farmers decide when to invest in a digital technology, including the option to invest now or wait a few years (Rojo-Gimeno et al. 2019). These analyses are even more important because the topic is complex and multi-factorial. In the field of agricultural robotics, for example, the economic study can be critical because some activities can only be carried out in certain seasons, and the robotic equipment must thus not be idle the rest of the time, at the risk of no longer being profitable over the long term. In addition, there is a general context of use and changing legislation that must be taken into account when considering profitability in a global way. For example, some European Union countries require that a human operator be physically present in the field where a harvesting robot is working. Such regulations would significantly reduce the economic benefit of agricultural robotics (Lowenberg-DeBoer et al. 2019). Some food safety regulations in Europe require a person to be present during milking, which is not possible with the 24-hour cycle of milking robots (Callum R. Eastwood and Renwick 2020)
Digital technology and our relationship with the living world
Culture and Nature: a risk of anthropomorphism
The famous efficiency of modern industrial agriculture depends on ignored and externalized costs for the environment and society. In this logic, nature plays the double role of source and dumping ground. On the one hand, it is seen as an unlimited provider of materials and processes necessary for agricultural production (e.g. soil fertility, water, pollination). On the other hand, it is seen as a dumping ground for externalities that occur during production (e.g., soil depletion, contamination, loss of biodiversity, greenhouse gases) and for natural resources that, while important, are not compatible with short-term profit maximization. This tendency to crisis stems from capitalism’s reliance on the four “cheap” – labor, food, energy, raw materials – to sustain each accumulation cycle (Ravis and Notkin, 2020). Input prices are kept artificially low by finding previously unmarketed spaces, appropriating unpaid labor in the service of commodity production. Most labor must be unpaid for profit to be possible – labor that has historically been performed by an unpaid, outsourced nature. However, economic growth leads to an increase in demand for these “cheapies,” then risking higher prices to ultimately threaten profits. The result is a perpetual search for new frontiers of appropriation. Historical capitalism has been able to resolve its recurrent crises because territorialist and capitalist agencies have been able to expand the zone of appropriation faster than the zone of exploitation. In other words, new frontiers of nature have been found or created quickly enough to keep input values low enough to maintain relatively stable rates of labor exploitation, and thus profits, over time. The danger for capital is the eventual exhaustion of all these frontiers (Ravis and Notkin, 2020).
The externalized costs of industrial agriculture are deeply contradictory in that they mask the deterioration of the very biophysical foundations of agriculture. To simplify, standardize, and mechanize agriculture, and to increase productivity per worker, per plant, and per animal, a series of biophysical barriers must be overcome. Indeed, modern agriculture has been accused of conceiving of plants and animals solely as inputs into an industrial process (Sparrow and Howard 2020). Efficiency gains thus depend on many unaccounted for, non-renewable and actively destructive solutions, with fossilized biomass playing an indispensable role in this process (Ravis and Notkin 2020). Nature is seen here simply in a utilitarian role, only for what it brings us and not for what it is in itself. This perspective is based on a Cartesian dualism that views nature and society as independent entities, ignoring the fact that nature and society are deeply intertwined and historically co-produced. Society does not only act on nature, it develops through the network of life. Nature is seen as something that must be controlled and dominated to satisfy human needs. Thus, the interactions between nature and societies are based on a reasoning that justifies and normalizes the appropriation, exploitation and, ultimately, the destruction of nature. (Gras and Cáceres 2020)
Technologies are essential for making nature accessible and usable by capital, since they embody the capacity of science to make it legible, knowable and measurable for capital. At the heart of this dialectic is the mediating role of technological innovations in capitalist agricultures: the development of procedures, mechanisms, devices, and organizational schemes to achieve leaps in efficiency and to extend the reach of capital over undercapitalized and uncapitalized nature. It is precisely in these undercapitalized and uncapitalized territories that the ratio of capital investment to economic gains is highest.
Taking into account the energy cost of Agtech tools
Insofar as digital technologies are often presented by Agtech actors as one of the technical solutions for meeting the food challenges facing agriculture while limiting environmental pressures (see the first part of this paper on the analysis of Agtech discourses), we cannot ignore the environmental assessment of digital tools themselves. Are we really interested enough in the impact that digital technology has on the environment? Is it worth it, or are the gains brought by digital technology significant – and positive – compared to what digital technology costs the environment? To avoid becoming completely schizophrenic and amplifying a problem that digital technology is supposed to solve, it is essential to tackle this issue head on. Digital technology is far from being energy neutral, and all the talk about the cloud and the immateriality of digital technology is helping to distance the collective consciousness from the environmental impact of digital technology. It’s a common feeling, that the bits of data – those 0s and 1s – are somehow detached from the material constraints that complicate the rest of the world. But digital technologies, big data, and artificial intelligence do not represent the reversal of matter. If only that were true, we could substitute cloud computing for petrochemicals and soil erosion (Carolan, 2017). The digital tools and services deployed within Agtech are not coming out of nowhere. Sensors, Connected Objects, data centers, networks, antennas, advanced agri-equipment, all of these technologies require resources and energy to operate (Clapp and Ruder 2020). And this is without taking into account the global increase in electricity consumption that is expected if massive use of digital solutions is to take place (Vinuesa et al. 2020). This increase in energy consumption would obviously not be exclusive to the agricultural domain, but agriculture would also have a significant impact. Interested readers can check out a previous blog post where I discuss this topic in much more detail.
Culture and Society – Our relationship to agriculture and the land
Digital technologies, and perhaps especially robots, are likely to impact the way people think about the natural world, food and agriculture. Research has begun to explore how consumers feel about food being produced and consumed in radically different ways, indicating that views on food production could be very different, as could attitudes about food choice and consumption or how the format of farms might change (Klerkx and Rose 2020). Many societies value the idea of a connection to the land. If agriculture one day becomes primarily a matter of deploying and supervising robots, these cultural narratives may be impossible to sustain (Sparrow and Howard 2020).
The vast majority of consumers are already far removed from the farms and production processes of the food they eat, and most are notoriously ignorant of what it takes to put it on their table. A vague cultural sense that food is produced by robots may further distance consumers from the realities of food production and facilitate consumption patterns, such as excessive consumption of red meat, that are now widely recognized as environmentally destructive (Sparrow and Howard 2020). Because of the standardization of food products necessary to facilitate early applications of robots (see the section on algorithmic rationality), there may also be a concern that consumers will come to expect even more strongly that all food is perfect, leading to increased food waste as fewer items are deemed fit for sale. (Sparrow and Howard 2020). Nonetheless, the public could become dissatisfied with the way food is produced, as other potential social implications, including concerns about perceived impacts on animal welfare or the use of digital technologies, could lead to public scrutiny (Rose et al. 2021).
(Lioutas and Charatsari 2020) worked specifically on the example of short circuits. For short-haul consumers, the farmers who distribute their products have an image of being hard-working, respectful of nature, and have the production of high-quality food as their top priority. Most consumers in the authors’ study tend to believe that the introduction of digital technologies into farming practices could irreversibly change this image, although the conceptual basis for such a belief remains rather unclear according to the authors (Lioutas and Charatsari 2020). Short circuits are based on an ancient way of producing food, which has an inherited value as it connects food producers, food and consumers. Food products are seen as the result of the seamless interaction between farmers and farms and are perceived by consumers as more pure (even agricultural products from conventional practices) than products sold through traditional distribution channels. The use of more sophisticated technologies in food production is seen as a threat to this purity. Although buyers seem to recognize the need for technology in agricultural practices, digital technologies are seen as exogenous invasions that can disrupt the harmony of farmer-food-consumer relationships (Lioutas and Charatsari 2020). This view is shared by some of the farmers in their study. It is interesting to note that both consumers and producers see digital agricultural technologies as a threat to the unconventional character of short circuits. Short supply systems are seen as an alternative to what some consumers call industrialized agriculture, or as relatively isolated islands within larger agri-food systems. Since digital technologies are seen as belonging to this industrialized world, farmers and consumers seem to fear that their introduction into short supply chains may transgress this unconventionality. (Lioutas and Charatsari 2020) nevertheless remind us that the fact that consumers and farmers consider digital technologies incompatible with short circuits does not mean that these technologies have nothing to offer to alternative food networks. However, their adaptation to the characteristics of highly intensive agriculture makes their contribution to alternative food production and distribution systems questionable.
Animal welfare and ethics
Since 2015, the animal is now recognized as a “living being endowed with sentience” in the Civil Code. This new regulation has restored values such as animal welfare or animal ethics to their rightful place, and it is clear that digital technologies that address animal production often refer to them. Nevertheless, when animal welfare, rather than animal rights, is seen as the central issue in animal husbandry, (intensive) animal production is generally seen as an acceptable, even desirable, form of animal use.
Even under standardized laboratory conditions, the notion of animal welfare has proven to be multifaceted, not easily defined at the species level, but dependent on the contexts, qualities, and life histories of particular animals, environments, and the humans around them. Despite all these hidden choices and variations, animal welfare tends to be used as if it were a (generic and objectifiable) quality of animal species. Both farmers and animals as active and idiosyncratic beings are absent from most approaches to welfare. Notions of animal welfare that would correspond to the experience of farmers are easily overlooked in public debate and policy making. Not only farmers but also animals are subject to the same limitations: animals are not just passive recipients of welfare. If one thinks that animal welfare could be better decided by consumers in supermarkets, one might fear that it is defined in ways that allow for easy commodification. On the other hand, if animal welfare is seen simply as a matter of regulation and enforcement by the state, there is a concern that the parameters that can be counted and measured objectively will be the ones most emphasized.
In this blog post, we have focused primarily on crop production – at least that is where the majority of the proposed examples have been drawn from. Nevertheless, it is interesting to note that, in the context of digital technologies in agriculture, many parallels can be drawn between the examples drawn from crop production and those applied to animal production. For example, we can mention in a non-exhaustive wa
- the limited application of digital tools for extensive livestock systems (see the section on the “precision divide”)
- the risk of treating live animals only as parts of a larger machine, or in other words, the risk of a tamagoshisation of animals (see the section on “algorithmic rationality”)
- the risk of diminishing the role of the caretaker and isolating the farmer if the caretakers and advisors visit the farm less often (see the section on “the advisor and his relationship with the farmer”)
- the risk of less interaction between the farmer and animals (see the section on “the change in the farming profession”) and of a change in the farmer’s knowledge (see the section on “some knowledge emphasized over others”)
- the risk of reducing animal welfare to readily available observations and the need to know what is measured and what is not (see the section on “the accuracy gap”)
- whether or not digital tools are actually being used for animals (see the section on “who digital tools are really for”)
- the fact of using digital technologies to give back its own identity to an individual in a large group or, on the contrary, to target a divergent [herd management by exception] (see the part on “agro-ecology and digital”)
- the use of digital tools in or on the animal, and the associated risk of physical injury (well, the parallel is harder to draw for the moment, except for granting special status to plants in the years to come, who knows!)
For interested readers, this short section focuses on animal welfare, and shows excerpts from the very nice article by (Driessen and Heutinck 2014) on the case of milking robots.
In their work, the use of the milking robot revealed that cows did not want to milk themselves (Driessen and Heutinck 2014). Offering cows the opportunity to be milked and relieve their udders would clearly not have been enough to get the cows to come within the optimal time frame. For the authors, this finding is seen as a sign that the promise of cow autonomy following the introduction of a milking robot was not fulfilled. Some sort of feed reward or forced delivery seemed necessary to avoid the need to fetch cows on a recurrent basis. Several authors pointed out the paradoxical nature of the discourse around milking robots, between the fact that the system generated more freedom for the cows and offered a more natural way of milking, and the efforts needed to get the cow into the robot and the actions to be taken for cows that refused to perform the required behavior. Note in passing that it would be excessively ironic if the device that was supposed to free the cows actually locked them in a barn. The cows’ newfound freedom would actually come at the price of being disciplined.
The authors emphasized that the cows (collectively) were not simply passive end users in the development of the automated milking system. Being relieved of their milk load was indeed not a sufficient motivation to visit the robot. In addition, the authors emphasize that the robot developers had to adapt to the cows’ behavior. For the authors, these elements indicate the active involvement of the animals in the technological design process, and the big mistake of excluding the possibility of an agential and significant form of domesticated bovine subjectivity. The milking robot forces cows to unlearn some of their herd mentality. Instead of being entirely individualized by the robot, it turned out that once the cows had learned to milk themselves and had become accustomed to the system, they moved from the pasture to the robot in regular groups of a few cows. The new character of the cow, which no longer associates the farmer with being driven into the milking area, also gives rise to different human-animal relationships. With a robot, the relationship between farmer and cow shifts from caring for the animals to allowing the animals to care for themselves. Thus, what a cow is, and how best to relate to it, is the result of ongoing processes of socio-technical change. And if, especially in the case of the modern high-yielding dairy cow, it is difficult to say where the technology ends and the animal begins, this does not exclude a true ethical position towards them. The evaluation of milking robots can only be understood as part of a broader change in practice and in terms of a complex process of co-evolution between partially material changes in technological systems and the behavioral practices of farmers and cows in a moral world where ethical norms and meanings of normative concepts are changing. The terms in which to evaluate emergent practice seem to have changed with the new device: new roles are configured, new experiences are generated, and human-animal relationships change in character. And perhaps even the nature of the animals – and humans – involved is altered in the process. (Driessen and Heutinck 2014)
In animal welfare debates, the question “but what do cows really want” can only be answered by interacting with them. A cow in a stanchioned stall may well want different things than a cow in a free stall, as they are expected to live a different lifestyle, with very different relationships with their herd and with humans. Still, one might worry about an animal getting used to bad behavior and believing that it feels good. In turn, a robot embodies a different conception of the good life (or perhaps just an acceptable life, or at least a life of reduced suffering) for dairy cows. While individual freedom has never been a major concern regarding herd animal welfare, it has become prominent in discussions of robotic farms. Thus, the co-evolution of ethics and technology is not simply a matter of marketing rhetoric accompanying a new device, but is part of a sincere process of reinterpreting and reorganizing practices in light of emerging norms and behaviors.
So, is this form of automation part of the ongoing process of scaling up and down the role of humans in agriculture, ultimately making farmers disappear? Or, on the contrary, does it help farmers to improve their working conditions and lifestyles? Again, (Driessen and Heutinck 2014) invite us to step back and consider these dichotomous positions too simply to subtly assess the implications that follow. Being a dairy farmer is not always conceived in terms of completely asymmetrical power relations in which only humans are in control. Rather, each material arrangement of human-animal relations is accompanied by different experiences of freedom and control for both the farmer and the cow. Although hiring a milking employee tends to be cheaper than a robot, a good employee is hard to find and would require very different management skills. Something not all farmers are able to acquire. The robot could also be a way to continue farming after a certain age when manual work becomes more difficult, and in the absence of a successor (we have already mentioned this point earlier in this blog post). The robot creates a new role within the family for farmers and a new division of domestic tasks. The farmer with a robot can also use the time saved to find a part-time job, to take up leadership positions in the cooperative organizations that are still numerous in dairy farming, or to work in teaching or local politics.
We can decide collectively and universally what kind of animal production would be acceptable and cause the least suffering, based on past experience with cow behavior, on what we think is common sense about appropriate ways to house cows, or on scientific studies in a variety of disciplines from physiology to ethology. But to leave it at that might reduce our attention to the forms of suffering that would remain and the potential ways to improve systems. (Driessen and Heutinck 2014) call for “asking the cow,” which can be understood as a combination of measurements of animal science parameters, such as health, heart rate, stress hormones, longevity, and production, but also as a protracted process of human-animal-technology interaction, while experimenting with management and adjusting technological facilities. The cow and her welfare are then seen not as something static to be discovered, but as something emerging with her new environment. (Driessen and Heutinck 2014) thus also invite us to reflect on the fact that evaluating new digital devices as objects with qualities, and enumerating the advantages and disadvantages of innovation, are tasks whose results are, at best, only temporary. This means that an ethical variable such as animal welfare cannot be defined only at the species level, for example for all cows, so that it can be fully determined by animal scientists measuring the parameters and behaviors of a significant number of representative animals. Instead, the welfare of farmed cows will depend to some extent on the characteristics of the breed as well as the abilities, experience, relationships and preferences of the individual animal – and the ability of farmers to adapt to them.
Even so, animal welfare is primarily a matter of feeling. The overriding question is whether it can be determined with digital technologies.
Changing the model and giving meaning
It is up to us to decide on the direction of digital technology
It is up to us, collectively, to decide what technologies do. Digital agriculture is neither inherently good nor bad, in part because, as Michael Carolan reminds us, these technologies must be understood as variable, contingent and diverse (Carolan 2020c). The debate about technologies cannot be binary. The double-edged nature of technology-that is, the tensions between optimism and unintended consequences that typically accompany technological innovation-also fosters contradictory positions. The widely divergent views in the precision technology debate reflect the extremes of this optimism-skepticism dynamic, with proponents focusing on the potential of new technologies to solve immediate environmental problems, while critics worry about the potential to introduce new environmental problems associated with these technologies (Clapp and Ruder 2020). The fact that access to and control of technological innovations can both concentrate power but also create new directions for agency over these technologies sheds additional light on the emerging complexities of the debate (Clapp and Ruder 2020). Some digital assemblages have greater emancipatory potential than others. Similarly, the critical questions about data sovereignty posed by digital technologies should not cause us to completely dismiss all aspects of agricultural digital simply because there is a data flow. Technology will come with us into the 21st century, but there are architectures that allow users to avoid data hoarding (Fraser 2019). This finding is important because it gives us a basis for making judgments about which platforms should be supported (Carolan 2020c).
Digital technologies – whether a platform or a sensor, for example – are often highlighted by the sheer volume of data generated or used. For Michael Carolan, whether these technologies rely on large (Big Data) or small (Small Data) volumes of data does not help us understand whether these digital technologies should be encouraged or challenged (Carolan 2020c). What do these volumetric attributes really tell us about the assemblages of these technologies, particularly in terms of what they enable and inhibit? Not much (Carolan 2020c). The term “Small Data” is beginning to emerge in response both to the fact that some data is relatively expensive or complex to acquire but also in response to the high energy consumption of digital technologies. The concept of “Smart Data” is also emerging, although one might reasonably ask what is behind it. Rather than trying to organize projects around recognizable terms such as “big data” and “small data,” and more broadly around the topic of digital agriculture, Michael Carolan prefers to think and organize these assemblages in terms of what they do, making it a project of political ontology. (Carolan 2020c). In particular, this author introduces the concepts of “strong” data and “weak” data, which he argues are more likely to relegate the politics of data to the forefront. Strong data” maintains the status quo, serving as a strategic containment of potentially more radical futures by enlisting, among other things, productivist imaginaries and visions of a world populated by individualized consumers as opposed to collaborative citizens. Weak data, on the other hand, represents assemblages of data that encourage the reconsideration of alternative futures. Weak data encourages dissent rather than stifles it, and speaks to those digital assemblages that are committed to inclusivity. They go even further by partnering with practices, embodiments, and associations related to the making of an activist citizen (Carolan 2020c).
Pursuing the development of digital agricultural technologies is a choice, a choice that makes certain futures more or less likely (Klerkx and Rose 2020). Innovation systems are not neutral. They may support transition pathways that include only certain future technologies and food systems, while excluding others (Klerkx and Rose 2020). To speak of a political ontology is to realize that digital platforms create worlds. And Michael Carolan sees it as the duty of social humanists to try to understand which worlds are associated with which platforms (Carolan 2020c). It is important to clearly conceptualize not only how these tools are used, but also by whom they are used and what determines their ability to transform agriculture. And then, from that understanding, build more emancipatory, inclusive, and collaborative digital futures. (Carolan 2020c). The ultimate balance of risks and benefits will depend on how robots and technologies are used. Ve are the humans who are responsible for the outcomes of technology deployment (Sparrow and Howard 2020). But it should be noted that whether or not this potential is realized will depend on economic and political choices (Sparrow and Howard 2020). It is the interaction of a technology with the social ecology that determines its environmental, social and human consequences. Once in place, the social system surrounding technologies is notoriously difficult to analyze (Bronson and Knezevic 2016).
Perhaps one of the first tasks would be to determine which challenges require a techno-centric solution (Rose et al. 2021). This also includes thinking about whether agricultural digital is a mission in itself, or rather a support for other missions (Klerkx and Rose 2020).
Toward responsible research and innovation
Creating and anticipating new imaginaries
Many authors in the humanities and social sciences emphasize the importance of both anticipating and assessing the consequences that (should) hit farmers and other network stakeholders and thinking about how to protect them from potential harm, but also thinking about the preferred direction in which the further development of digital technologies should take place (van der Burg, Bogaardt, and Wolfert 2019). It is true that agricultural innovation systems often favor a particular set of technologies, but remain relatively unclear about the values that underlie them. Likewise, it is not really clear how technologies contribute to shaping particular transition paths to sustainability and underpinning current technological and economic paradigms or fostering deep transitions (Klerkx and Rose 2020).
The concept of Responsible Research and Innovation (RRI) is now a recurring theme in the scientific literature, implying that future research should support the co-evolution of technology and society in a desirable way. In proposing to introduce RRI into the development of digital agriculture, the objectives are multiple. It is already about making technologies more explicit about the diversity of transition pathways they envision, about what can be game-changing about them, about the inclusionary and exclusionary choices that are made, and about the cross-fertilizations that can exist in seemingly very different agricultural and food system futures (Klerkx and Rose 2020). This also means being very clear about the problems that these technologies are intended to solve (Klerkx and Rose 2020). Digital technologies are bound to become much more “mission-oriented” in the sense that they would actively seek to stimulate directions deemed desirable, and also actively destabilize existing systems that are not considered sustainable. There is also a need for these technologies to be context sensitive and for us to recognize that technologies are not universal, but evolve with, and are embedded in, different contexts in terms of socio-economic, cultural and political contexts. This may also involve looking at where digital technologies, retro-innovation, or frugal transitional systems meet (Klerkx and Rose 2020). Some authors nevertheless criticize this RRI in digital agriculture for not always succeeding in producing adequate configurations. It would operate via a misplaced emphasis on the fact that agricultural innovation can successfully reconfigure sociotechnical relations in a field, without also pursuing systemic or structural change (Fraser 2021). Alistair Fraser emphasizes the need to reimagine agricultural digital innovation processes to avoid the misconfigured innovations that are evident to him in current developments (Fraser 2021). We should also seek to anticipate the consequences of alternative scenarios, asking whether or not not not implementing digital technologies would create more losers than winners, and if so, asking how and why (Klerkx and Rose 2020).
Responsible transitions are much more likely to occur if we consider the consequences proactively rather than reactively (as is often the case now) (Klerkx and Rose 2020). This is not an easy thing to do, however, as Collingridge’s dilemma points out, in that the impacts of a technology cannot be easily predicted until the technology is widely developed and used, and at the same time, change is more difficult to effect the more established the technology is. Although the eventual use of technology is never fully predictable and thus it is impossible to know in advance what ethical issues will arise, (Ryan et al. 2020) nevertheless believe that the work on ethics in artificial intelligence and Big Data has identified a number of ethical issues that can reasonably be expected. Nevertheless, it is necessary to remain humble in the sense that technological development creates an environment of change when so much is already uncertain. Risk and uncertainty management techniques are necessary to assist in decision making in complex and variable environments and are essential components of technology development, use, and training (Fleming et al. 2021). Foresight exercises, incorporating interrelated factors, such as the future effects of climate change on agricultural production, natural resource depletion, or the social cost of potentially replacing workers with machines, should provide a complete picture of the balance between positive and negative impacts of digital technologies (Lioutas, Charatsari, and De Rosa 2021)
All the imaginable transformations due to digital innovation require us to anticipate some of the social and political effects of digital technologies. One approach to doing this would be to bring the moral compass upstream to the industry, and in particular to the designers of these digital technologies, to interrogate their social and moral values that guide the design and innovation process. This approach would push innovators and other policy actors to not only manage the risks of an innovation after the fact, but to consider the socio-ethical dimensions of technologies from the earliest (upstream) stages of the innovation process (Gardezi and Stock 2021).
In the rush to adopt digital agricultural technologies, there is a risk of forgetting the broader network of other innovations that play an important role and may also affect societies in different ways. (Carolan 2020b) is concerned about the extent to which some anticipated futures exclude alternative futures, potentially making them unthinkable (let alone feasible). The concept of responsible innovation (RRI) should not only be attached to new and emerging technologies, which would bring to the forefront questions about possible alternatives and the direction of innovation paths. (Rose and Chilvers 2018). Alternative visions of food and agriculture articulated by different actors may not involve digital agricultural technologies in the future of agriculture, or seek to use them alongside other concepts such as agroecological systems. Until we articulate inclusive visions of the future, it will be difficult to begin to anticipate the impacts of the transition and how to make them more accountable. Certainly, the pursuit of visions determined by a select group of people (policymakers or other powerful actors) is unlikely to be responsive (Klerkx and Rose 2020). A central focus of RRI, therefore, is to organize reasoned deliberations about technological needs and concerns between historically marginalized food system actors and key government decision makers. One ambition is for RRI to become a rubric for guiding innovation toward socially and ethically acceptable ends (Fraser 2021).
Several authors suggest a framework to guide technology development, including four key dimensions: anticipation, inclusion, reflexivity, and responsiveness. (Rose and Chilvers 2018). There should be many opportunities to assess whether mutually beneficial trajectories are being followed and to respond quickly to problems caused by a new technology (Rose and Chilvers 2018). And this work could take many forms: processes of projecting into the future (foresight exercises, road mapping, scenario testing), multi-stakeholder and interdisciplinary projects (with embedded social scientists), and public engagement (Regan 2021). However, these exercises must be carefully managed to avoid generating negative connotations, disproportionately amplifying risks, and hindering innovation.
Bringing multi-disciplinary actors to the table
Agricultural research is still dominated by top-down, non-inclusive approaches, and rarely includes relevant stakeholders, such as farmers, at an early stage. (Rose and Chilvers 2018). Farmers, positioned as the key agents enabling or preventing technological change, would not necessarily be involved in its design or implementation (Higgins and Bryant 2020). Hassan Ebrahimi and colleagues mention, for example, that the frequency with which each stakeholder group is mentioned in the media supports the conclusion that a small number of stakeholder categories receive the majority of media attention. The authors suggest that there is a bias in public discourse largely in favor of industry and government actors (Ebrahimi, Sandra Schillo, and Bronson 2021). Journalists or government agencies conducting consultations on digital agriculture are thus called upon to review their constitution of a representative sample and systematically identify respondents belonging to the missing categories. (Bronson and Knezevic 2016) blame crowdsourcing tools that, while they draw on farmers’ knowledge, do not necessarily invite farmers to shape the context in which that knowledge is collected. The authors then ask who has a role in deciding the context of data production, storage, and use of particular data tools used in food and agriculture. Or who decides what types of data to collect, given the operation of current digital data collection and analysis tools. (Gardezi and Stock 2021) blame certain digital assemblages for savagely reshaping agricultural work by rendering certain trades, especially unskilled workers, obsolete, but without any real dialogue or debate, especially with those who would be most negatively affected by these trends, i.e., the unskilled workers themselves. According to the authors, thinking that certain digital tools could fundamentally alter the politics surrounding the immigration debate, turning influential actors who for decades were in favor of immigrant labor (e.g., the agribusiness industry) and turning them into closed-border zealots, requires some joined-up thinking.
Digital tool providers, farmers, or even distributors need to be seen as a community of precision farmers or digital tool users, rather than a product-retailer-customer model (C R Eastwood, Chapman, and Paine 2009). Given that farmers and consumers actively and reciprocally co-transform digital technologies into value-we have, for example, given ample examples of this on milking robots-there is a need to focus more on how these two groups-and other actors involved in agri-food systems-design these technologies (Gardezi and Stock 2021). The major role of technology should be to facilitate the explicit intersection of multiple disciplines. (Caquet, Gascuel, and Tixier-Boichard 2020) also note that agronomy, ecology, management sciences, and geography are still only marginally involved in this technological advance, which is primarily driven by engineering sciences, algorithms, robotics, geo-localization by guidance, wireless connection of physical sensors, and coupling of information in a system controlling solenoid valves. (Caquet, Gascuel, and Tixier-Boichard 2020). The challenge of identifying the myriad of stakeholders affected by agricultural technologies, from farmers, farmworkers, and advisors, to manufacturers, retailers, consumers, and rural communities, to the supply chain, is significant. Yet, (Rose et al. 2021) suggest that it should be possible to map stakeholders starting with the farmer’s circle of trust before expanding outward and considering who will be affected by this innovation (Rose et al. 2021).
(Regan 2021) refer to RRI readiness as the ability of key actors to integrate RRI dimensions into research and development projects and initiatives. The authors note, however, that there is a fundamental component to people’s attitudes toward this philosophy: Do these actors believe in the value of RRI? Are they motivated to engage in RRI activities? But (Regan 2021) argue that there is also an external component to this readiness: Are key actors empowered by their social and cultural environment to practice RRI? Do existing infrastructure, relationships, and mechanisms support RRI activities? (Regan 2021). Working with a scientific audience, the authors realized that tensions existed between what scientists perceived as their role as scientists and how RRI exercises aligned with that role. Scientists did not always seem inclined to embrace the values of RRI as a whole. First, because they felt that these values were not rewarded or supported institutionally or culturally. But also because scientists do not always engage with non-academic or lay audiences (Regan 2021). According to the authors, scientists would benefit from incorporating more reflexivity into their work, or in other words, to think critically about their own assumptions, values, and interests, and to actively consider the views and values of others and how they may match or conflict with their own views. Yet the authors emphasize that it is a democratic right for the public to have a say in the decisions that affect them, and their input ensures a more complete and diverse body of knowledge, allowing for decisions that are more likely to be consistent with local needs and priorities. If these notions of reflexivity are not integrated into the imagination of socio-technical transitions, then innovation cannot be responsible (Klerkx and Rose 2020).
Reflecting the society we want
Digital technology at the service of agro-ecology?
The ability of digital and agri-equipment to specifically carry an agro-ecological agriculture model remains a gamble, as these technological developments may also reinforce further industrialization of agriculture, continuing sixty years of development with the goal of productivity gains (Caquet, Gascuel, and Tixier-Boichard 2020). (Rotz, Duncan, et al. 2019) also note that discussions around the advancement and adoption of agricultural technologies continue to be heavily focused on technology, thereby suppressing opportunities for digital technologies to support small-scale agroecological approaches. At present, digital technologies shift the scale of attention and intervention from the plot to an intra-plot level, but they do not challenge the monoculture, the factory field itself, the literal and figurative soil upon which it is built. Yet there is no intrinsic reason why current digital technologies, which allow for finer interventions in food production, cannot be designed to facilitate greater ecological complexity without compromising productivity. This could mean finding ways to enable greater floral and faunal complexity around fields, for example using digital technologies to negotiate conflicts in a multi-species ecological environment. The idea is to value heterogeneity as much as possible, and to move away from the concept of “one size fits all”. Digital technologies must be rethought to encourage, not eliminate, teeming complexity and thus help shape technology in better alignment with the real needs of people and other life forms (Miles 2019). Hill and Macrae’s (1986) classification of strategies for supporting the transition to more sustainable agriculture provides an interesting framework for rethinking the use of digital technology from an “efficiency” approach where the goal of the strategy is to optimize the existing production system, to “substitution” and “redesign” strategies where the goal is to address the intrinsic causes of the problem and rethink the production system.
However, alternative imaginings of the use of digital tools are still too little present. (Lajoie-O’Malley et al. 2020) show, for example, that even in the texts of major international institutions – the World Bank, the FAO – there is no appearance of alternative narratives of the future in which social and political innovation would allow transitions away from the industrial production model. Digital agricultural technologies, according to the authors, could however be deployed in alternative visions, such as agro-ecological and circular food systems or in short supply chain models (Lajoie-O’Malley et al. 2020).
A technology in support of agroecology can be defined by its contribution to informing or controlling the processes that underpin agroecological principles (Caquet, Gascuel, and Tixier-Boichard 2020). Much of the knowledge in agro-ecology can only be taken into account if dedicated agri-equipment exploits it to modulate its action. For example, to take advantage of the complementarities between several plant species in the same plot, harvesters must be able to collect a crop from a composite stand. The cognitive challenges of agri-equipment for agroecology thus concern the characterization of agroecosystem functioning, the coverage of biological regulations, the release of fertilizing elements through the degradation of organic matter, carbon storage capacity, and water retention potential.
(E. Schnebelin 2021) observed that the majority of research on digital farming focused on better understanding the adoption of digital technologies by farmers, without really looking into how digital technologies were profoundly changing farm practices and organization. In particular, the authors questioned whether the development of digital technology benefited all greening paths, or whether it favored some at the expense of others. According to (É. Schnebelin, Labarthe, and Touzard 2021), not all digital technologies under development are considered suitable for organic farming, either for technical or socio-economic reasons: they may not be suitable for the economic model or for farmers’ ways of thinking and decision-making. This may be similar to the precision divide discussed above. (E. Schnebelin 2021) note that the choice of agronomic practices and the choice of tools have an effect on each other, and that the use of technological production tools seems to favor the optimization of the agricultural itinerary rather than its redesign. This finding must be qualified, however, as the authors also observed links between more radical changes in practices and the use of digital technologies (E. Schnebelin 2021). The authors also found that there are several trajectories of digitization with, behind a single usage profile, a variety of logics. The same technology can be used differently, with different objectives. (E. Schnebelin 2021). The authors add that digital technology seems to favor a stronger form of greening: the development of organic farming on large farms. This finding would echo a political and academic debate on the conventionalization of organic farming, with the underlying question of whether digital could be a gas pedal of this conventionalization, to the detriment of more radical organic farming, and smaller farms (E. Schnebelin 2021).
Interestingly, digital tools are not intellectualized in the same way by all actors. Conventional agricultural actors seem to see digitization as a way to create new economic opportunities, while organic actors see it more as a way to develop knowledge (É. Schnebelin, Labarthe, and Touzard 2021). In particular, conventional agricultural organizations stress the importance of developing new types of knowledge within their structure, such as agricultural cooperatives. Jobs and dedicated teams are created specifically for digitization, and awareness and training actions are conducted. Organic organizations, on the other hand, seem to put more emphasis on the need to develop farmers’ skills. (É. Schnebelin, Labarthe and Touzard 2021). The research illustrated another related problem caused by different uses and meanings of the same concept by different actors, with some confusingly trying to pull actors in agricultural and food systems in different directions. In compiling a list of concepts associated with sustainable agriculture and using it to assess how the concept of “integrated farm management” fit into these concepts, authors have pointed to some confusion within the agricultural community. This confusion is important because knowledge exchange activities to promote more sustainable agricultural practices need to deliver simple, actionable messages to farmers without making them feel bombarded by a range of different and potentially competing ideas (Klerkx and Rose 2020). The case of agro-ecology is completely applicable here.
In the agricultural sector, many agtech start-ups propose a model in which the digital service offer is remunerated on the basis of a possible maintenance of yields while saving on inputs (fertilizers and pesticides). These economic models are rather short-term and seem rather fragile. One can already wonder if these models are applicable to orientations towards agro-ecological practices, potentially without inputs. With climate change regularly asserted by climatologists, one can also wonder if the promises of increased yields can be kept. More generally, we live in an extremely changing and uncertain environment. When we place ourselves in a context of precision agriculture, between the volatility of raw material, input and production sale prices, with the observed variability of the climate, with the variability of soil and subsoil within the plots, which are often extremely marked, we could even come to question the relevance of modulated agronomic applications on the plots compared to a uniform but more considered application. It should also be remembered that economic models only hold if one ignores the negative externalities and the free services provided by nature. The agricultural sector is undoubtedly neither the only one nor the worst, but it illustrates the fact that the artificialization of the digital must undoubtedly be extended to the artificialization of our accounting balance sheets.
Data regulation and ethics
A wide range of new technologies is developing very rapidly, significantly affecting people’s way of life as well as environmental impacts, requiring new steering procedures from governments. The problem, according to (Vinuesa et al. 2020), is that neither individuals nor governments seem to be able to keep up with the pace of these technological developments – this is illustrated by the lack of appropriate legislation to ensure the long-term sustainability of these new technologies. A first step in this direction would be to establish adequate policy and legislative frameworks to help direct the vast potential of artificial intelligence toward the greatest benefit for individuals and the environment, as well as toward achieving sustainable development goals (Vinuesa et al. 2020). If public agri-food policy does not develop goals and objectives for how these technologies could be developed and supported by small-scale agriculture and alternative forms of agri-food production, these technologies will remain in the hands of those who can pay, and as these technologies evolve, economic divisions will only widen on farms (Rotz, Duncan, et al. 2019). As Michael Carolan points out, we need to shift gears when it comes to protecting people, which is not the same as protecting a specific job (Carolan 2020b).
Agricultural policies have a heavy task of ensuring that digitization is done ethically, fairly, and inclusively. There is a need for new codes of ethics that protect farmers’ rights to their own data and prevent the concentration of power in technology providers (Lioutas, Charatsari, and De Rosa 2021). These ethical issues become even more important as one can envision artificial intelligence applications that improve the outcomes of the Sustainable Development Goals while not being fully aligned with the ethical guidelines for artificial intelligence. It then becomes necessary for applications of digital technologies that target the Sustainable Development Goals to be open and explicit about guiding ethical principles, also by explicitly stating how they align with existing guidelines (Vinuesa et al. 2020). A one-size-fits-all set of utilitarian ethical principles with artificial intelligence would not be advisable due to the high complexity of our societies.
To talk about governance through algorithms is to put the humans involved in the driver’s seat. Getting under the hood of these algorithms, as Michael Carolan puts it, and pointing out the norms present in digital technologies does not mean criticizing the fact that these technologies are value-laden. No socio-technical assemblage exists without having politics. Those who criticize digital technologies on the grounds that they are biased are engaging in a never-ending struggle – one cannot avoid creating these platforms in the absence of value judgments. The act of pointing out these norms is more about interrogating the kinds of values at work and the design processes that allow access to some voices but not others. To speak of governance through algorithms is to target points in technological assemblages where humans have a say in what digital technologies look like and what they look like (e.g., monoculture or multi-culture orientation) to ensure that these policies are truly participatory (Carolan 2020a). To address these ethical dilemmas in this way, it is important that all applications provide openness about the choices and decisions made during design, development, and use, including information about the provenance and governance of the data used in the algorithms, and whether and how they align with existing artificial intelligence guidelines (Vinuesa et al. 2020). Applications of artificial intelligence that positively impact the well-being of society do not always benefit each individual separately. This inherent dilemma between collective and individual benefit is relevant to applications of artificial intelligence, but it should not be resolved by the application of artificial intelligence itself. It is a problem that has always affected humanity and cannot be solved in a simple way, since such a solution requires the participation of all stakeholders (Vinuesa et al. 2020).
The lack of interpretability of artificial intelligence, which is currently one of the challenges of ongoing research, adds a further complication to the implementation of these regulatory measures. In particular, this implies that artificial intelligence algorithms (which are trained with data consisting of past regulations and decisions) can act as a mirror reflecting biases and unfair policies. This nevertheless presents an opportunity to potentially identify and correct some errors in existing procedures (Vinuesa et al. 2020).
It should be clear that the role of government does not end once innovations are adopted; a period of continuous reflection is needed, which will require updates to legislation, guidelines, and possible support for various technologies in the form of skills training, infrastructure improvements, or perhaps funding (Rose et al. 2021).
Perhaps the best way to regulate new technologies remains to regulate human interactions with technology use, not to regulate the technology itself (Wiseman, 2018). As a result, rather than seeking clarity in the law in relation to data ownership, (Wiseman et al., 2018) suggests that countries – and Australia in particular in their case – play a strong role on the benefits associated with the potentials of digital agriculture by organizing and engaging in debates on the topic. Moving from the question of “who owns the data” to a focus on a critique of how data aggregators collect and handle data would be aligned with something more transparent about data management. According to the authors, there should be a shift away from the issue of data ownership and instead address issues of data governance, and clarify the goals of good data management so that there is a more open culture about the control, access, and sharing of agricultural data (Wiseman et al. 2018).
(Lioutas, Charatsari, and De Rosa 2021) have interestingly presented the digitization of agriculture as a new trolley dilemma, a situation in which certain groups and values must be sacrificed to achieve high importance goals. According to the authors, the current trajectory of agri-food production development threatens the future sustainability of agriculture by endangering the climate, depleting natural resources, leading to overconsumption of water, and finally jeopardizing food security. There is no doubt that such a path endangers poor and food insecure consumers. Digitization, which has appeared as a promising solution to the food problem, raises questions for the authors. Would we be willing to sacrifice ethical and political values, jeopardize the well-being of certain social groups, and endanger cultural and environmental stability to achieve increased production? (Lioutas, Charatsari, and De Rosa 2021)
Michael Carolan insists that trends toward lock-in with digital tools are not inevitable (Carolan 2020a). The author argues that the way we do governance must reflect the types of lock-in we face and the way actors and actants are involved (Carolan 2020a)
Open source and open data
When sociotechnical imaginaries privilege the values of profitability and competitiveness, we should not be surprised that the spirit of collaboration is crowded out (Carolan 2020c). And while some data assemblages reinforce corporate dependencies, we would, according to Michael Carolan, have reason to look to networks that enable the co-production of knowledge and a politics based on inclusivity and a broad understanding of access to data and tools (Carolan 2020c). Using a classification that contrasts top-down versus bottom-up innovation and closed versus open platforms in Canada, Philipps et al. (2019) identify four potential business models of digital technologies: (i) the enterprise model; (ii) strategic networks; (iii) primordial systems; and (iv) perfect competition or the hacking universe (Klerkx, Jakku, and Labarthe 2019). Although Philipps et al. (2019) do not take a position on these four economic models, it is unclear which economic model typology will prevail and serve as a model for agriculture. Nevertheless, the authors call for strong governance over data ownership and control. Several authors point to the fact that with digital technologies, farmers no longer have the option of relying on “do it yourself” strategies to repair or tune devices – proprietary digital software and technologies, protected by intellectual property, clearly play into this. And to highlight the presence of many activist groups of farmers, scientists and engineers working on technologies that they believe will support a variety of agricultural operations through a more open design framework, particularly with attributes of open-source and non-hierarchical framework. A framework that notably engages with a variety of end users and aims to redress historical exclusions from the food system (Bronson 2019) (Carolan 2017) (Kernecker et al. 2020).
One might tend to think that opening up data to as many people as possible is natural, in the sense that since data would be social in nature, it should be shared (van der Burg, Bogaardt, and Wolfert 2019). This openness would allow a larger number of people to use the data and thus to mutualize knowledge as much as possible. Authors defend open access as a way for government or members of the general public to monitor or control whether digital technologies are actually helping to solve societal problems, such as reducing the ecological footprint of farms, reducing waste, or promoting food security and public acceptability of the production system. Data, for some, must be open in order to respect the people’s right to information power.
Not everyone agrees, however. In global health or humanitarian crisis situations, the goal is to save lives; and by that metric, the idea that data should be shared is a powerful exhortation. But for some, the question is not how to mobilize more information, delivered more quickly to more places. It’s about asking what are the most fundamental rights of our humanity. There is considerable tension between different rights – the right to privacy, for example – and especially between what we can do and what we should do to solve global public problems. Climate change is inherently a global crisis. It requires very broad participation to recognize that it is a problem and to develop collective responses to address it (Espinoza and Aronczyk 2021). Free access and openness of data may also not be seen as necessarily equitable, as data may be used to inform the actions of some, while others will not know how to interpret it. This raises the question of whether and to what extent open access actually promotes societal goals, and who would use it (van der Burg, Bogaardt, and Wolfert 2019). This is based on the realization that open data has the real potential to exacerbate as well as mitigate injustices. In other words, we need to be aware of the myriad ways in which social privilege can be embedded in datasets, which means that open data cannot be expected to universally promote justice without additional work. Perhaps it should be considered a “commons.” A digital technology, for example, should not belong to a single entity, but rather to a community. And as part of this shared community, it is essential that not only the code be shared but also the knowledge, time, experience, and stories needed, so that the technological assemblage is distributed equitably (Carolan 2020c). Michael Carolan gives the example of a platform launched in London to provide local consumers with access to local food. The author explains that grey areas emerged once questions arose around access to the platform, including by whom and to what. Not everyone actually had access to the vision of what an alternative food network should look like – consumers were overwhelmingly middle class. And Michael Carolan concludes that the very fact of being a proprietary platform means that the access offered to users is entirely as participants in a supply chain rather than as citizens of a community (Carolan 2020c).
The data intensity of artificial intelligence applications creates another problem. The need for increasingly detailed information to improve artificial intelligence algorithms conflicts with the need for more transparent handling and protection of personal data. This is also an area where more research is needed to assess potential long-term negative consequences. All of the above challenges culminate in the academic discourse on the legal personhood of robots, which can lead to alarming narratives of technological totalitarianism (Vinuesa et al. 2020). If big data and algorithms are indeed transforming our world into a black box society, with hidden algorithms controlling ever more aspects of our lives, then we cannot afford to leave these technologies unexamined (Visser, Sippel, and Thiemann 2021).
Conclusion
Debates around the Agtech ecosystem illustrate deep divisions; proponents and detractors often seem to talk past each other, with each side having a very different interpretation of how the same technologies will affect environmental outcomes. Conceptualizations as broad as digital agriculture are subject to both praise and criticism; a persistent tension between the value of a holistic, non-prescriptive approach and the dangers of a catch-all word used to capture investment and justify questionable agendas (Whitfield, Challinor, and Rees 2018). The narrative that will eventually dominate public discourse is critically important to understanding the environmental consequences of these technologies and their potential role in more environmentally friendly food production. How these debates play out is also important for struggles to emerge around the policy and governance frameworks that will set the rules by which these technologies must operate (Clapp and Ruder 2020). Each narrative has its contradictions or counter-narratives (Fleming et al. 2018), but food systems would nonetheless be much better served if the tensions within the discourse on digital technologies were addressed head-on, rather than imagining that technology will easily, wholly positively, and equitably lead to quantum leaps in agriculture (Duncan et al. 2021).
In recent years, the humanities and social sciences have taken up agricultural technologies. As non-technologists, their contributions are valuable for considering the development of tools in a broader way and for putting words to phenomena at work that we cannot always explain properly. And it is their contributions that have been presented in this blog post. Two main criticisms can nevertheless be made.
While the processes of research and knowledge production in the social sciences and humanities are evolving impressively with digital technologies and data, the development of theory in the social sciences and humanities is much less dynamic and mostly follows traditional paths that lead back to classics like Karl Marx or digital copies of 19th century books from which the humanities and social sciences hope to extract solutions to 21st century problems with digital technologies, big data, or even artificial intelligence (Ossewaarde, 2019; Roth, 2019). Such resurrections of the giants of these social disciplines are certainly as entertaining and self-affirming as they are practical. Yet they fail to address the truly essential question of how deeply the digital transformation of research and knowledge production is changing the epistemic core of the humanities and social sciences. Digital methods not only provide ever larger data sets to test old theories, but they also enable and even call for new forms of digital theorizing. The development of new digital social theories thus remains a laudable goal, the realization of which is complicated, however, by the fact that most social scientists know computers and programming languages as little as illiterate medieval farmers knew the Bible (Roth, 2019). The authors even add that the most erudite sociologists are among today’s (computer) illiterates and, as a result, are completely dependent on the small community of computer literate specialists who understand and shape the digital ecosystem (Roth et al. 2019). Marinus Ossewaarde suggests, however, that we should be careful that in trying too hard to reinvent new theories to address the topic of agricultural digital, the social sciences become too assimilated into the current dominant system of thought and discourse, and lose any critical thinking and creativity to elevate the debate. In a way, the author calls for the humanities not to sell their souls too much either… (Ossewaarde, 2019).
The humanities and social sciences regularly call in their work for a crossover of stakeholders in the elaboration and development of digital technologies, but also for a crossover of scientific disciplines. The concept of responsible innovation and research, which we discussed in the last section of the blog post, being moreover presented as one of the ways to achieve this. The goal is very laudable, but it is particularly surprising that the humanities and social sciences themselves do not really follow their recommendations. Almost none of the scientific literature cited in this blog post has been written by multidisciplinary teams, mixing both technical and social skills to analyze how digital technology interferes with farms. However, there is no lack of expertise…
Soutenez Agriculture et numérique – Blog Aspexit sur TipeeeReferences
Atelier Paysan. 2021. “Reprendre la terre aux machines. Manifeste pour une autonomie paysanne et alimentaire”. Anthropocène Seuil. 274 pages. (Taking back the land from the machines. Manifesto for peasant and food autonomy, Not translated in English)
Bertoglio, Riccardo, Chiara Corbo, Filippo M. Renga, and Matteo Matteucci. 2021. “The Digital Agricultural Revolution: A Bibliometric Analysis Literature Review.” http://arxiv.org/abs/2103.12488.
Bronson, Kelly. 2019. “Looking through a Responsible Innovation Lens at Uneven Engagements with Digital Farming.” NJAS – Wageningen Journal of Life Sciences 90–91.
Bronson, Kelly, and Irena Knezevic. 2016. “Big Data in Food and Agriculture.” Big Data and Society 3(1).
Bryant, Melanie, and Vaughan Higgins. 2021. “Securitising Uncertainty: Ontological Security and Cultural Scripts in Smart Farming Technology Implementation.” Journal of Rural Studies 81: 315–23.
van der Burg, Simone, Marc Jeroen Bogaardt, and Sjaak Wolfert. 2019. “Ethics of Smart Farming: Current Questions and Directions for Responsible Innovation towards the Future.” NJAS – Wageningen Journal of Life Sciences 90–91.
van der Burg, Simone, Leanne Wiseman, and Jovana Krkeljas. 2020. “Trust in Farm Data Sharing: Reflections on the EU Code of Conduct for Agricultural Data Sharing.” Ethics and Information Technology.
Caquet, Thierry, Chantal Gascuel, and Michèle Tixier-Boichard. 2020. L’agroécologie : Des Recherches Pour La Transition.
Carolan, Michael. 2017. “Publicising Food: Big Data, Precision Agriculture, and Co-Experimental Techniques of Addition.” Sociologia Ruralis 57(2): 135–54.
———. 2020a. “Acting like an Algorithm: Digital Farming Platforms and the Trajectories They (Need Not) Lock-In.” Agriculture and Human Values 37(4): 1041–53.
———. 2020b. “Automated Agrifood Futures: Robotics, Labor and the Distributive Politics of Digital Agriculture.” Journal of Peasant Studies 47(1): 184–207.
———. 2020c. “Digitization as Politics: Smart Farming through the Lens of Weak and Strong Data.” Journal of Rural Studies.
Clapp, Jennifer, and Sarah Louise Ruder. 2020. “Precision Technologies for Agriculture: Digital Farming, Gene-Edited Crops, and the Politics of Sustainability.” Global Environmental Politics 20(3): 49–69.
Driessen, Clemens, and Leonie F.M. Heutinck. 2014. “Cows Desiring to Be Milked? Milking Robots and the Co-Evolution of Ethics and Technology on Dutch Dairy Farms.” Agriculture and Human Values 32(1): 3–20.
Duncan, Emily. 2018. An Exploration of How the Relationship between Farmers and Retailers Influences Precision Agriculture Adoption.
Duncan, Emily, Alesandros Glaros, Dennis Z. Ross, and Eric Nost. 2021. “New but for Whom? Discourses of Innovation in Precision Agriculture.” Agriculture and Human Values (June). https://doi.org/10.1007/s10460-021-10244-8.
Eastwood, C R, D F Chapman, and M S Paine. 2009. “Farmers as Co-Developers of Innovative Precision Farming Systems.”
Eastwood, Callum, Margaret Ayre, Ruth Nettle, and Brian Dela Rue. 2019. “Making Sense in the Cloud: Farm Advisory Services in a Smart Farming Future.” NJAS – Wageningen Journal of Life Sciences 90–91.
Eastwood, Callum R., and Alan Renwick. 2020. “Innovation Uncertainty Impacts the Adoption of Smarter Farming Approaches.” Frontiers in Sustainable Food Systems 4.
Ebrahimi, Hassan P., R. Sandra Schillo, and Kelly Bronson. 2021. “Systematic Stakeholder Inclusion in Digital Agriculture: A Framework and Application to Canada.” Sustainability (Switzerland) 13(12).
Espinoza, Maria I., and Melissa Aronczyk. 2021. “Big Data for Climate Action or Climate Action for Big Data?” Big Data and Society 8(1).
Fairbairn, Madeleine, and Julie Guthman. 2020. “Agri-Food Tech Discovers Silver Linings in the Pandemic.” Agriculture and Human Values 37(3): 587–88.
Fielke, Simon, Bruce Taylor, and Emma Jakku. 2020. “Digitalisation of Agricultural Knowledge and Advice Networks: A State-of-the-Art Review.” Agricultural Systems 180.
Fleming, Aysha et al. 2019. “Is Big Data for Big Farming or for Everyone? Perceptions in the Australian Grains Industry.” https://hal.archives-ouvertes.fr/hal-02105890.
———. 2021. “Foresighting Australian Digital Agricultural Futures: Applying Responsible Innovation Thinking to Anticipate Research and Development Impact under Different Scenarios.” Agricultural Systems 190.
Fraser, Alistair. 2019. “Land Grab/Data Grab: Precision Agriculture and Its New Horizons.” Journal of Peasant Studies 46(5): 893–912.
———. 2021. “‘You Can’t Eat Data’?: Moving beyond the Misconfigured Innovations of Smart Farming.” Journal of Rural Studies.
Gardezi, Maaz, and Ryan Stock. 2021. “Growing Algorithmic Governmentality: Interrogating the Social Construction of Trust in Precision Agriculture.” Journal of Rural Studies 84: 1–11.
Garnett, T. et al. 2013. “Sustainable Intensification in Agriculture: Premises and Policies.” Science 341(6141): 33–34.
Gras, Carla, and Daniel M. Cáceres. 2020. “Technology, Nature’s Appropriation and Capital Accumulation in Modern Agriculture.” Current Opinion in Environmental Sustainability 45: 1–9.
Higgins, Vaughan, and Melanie Bryant. 2020. “Framing Agri-Digital Governance: Industry Stakeholders, Technological Frames and Smart Farming Implementation.” Sociologia Ruralis 60(2): 438–57.
Higgins, Vaughan, Melanie Bryant, Andrea Howell, and Jane Battersby. 2017. “Ordering Adoption: Materiality, Knowledge and Farmer Engagement with Precision Agriculture Technologies.” Journal of Rural Studies 55: 193–202.
Ingram, Julie, and Damian Maye. 2020. “What Are the Implications of Digitalisation for Agricultural Knowledge?” Frontiers in Sustainable Food Systems 4.
Jakku, Emma et al. 2019. “‘If They Don’t Tell Us What They Do with It, Why Would We Trust Them?’ Trust, Transparency and Benefit-Sharing in Smart Farming.” NJAS – Wageningen Journal of Life Sciences 90–91.
Kernecker, Maria et al. 2020. “Experience versus Expectation: Farmers’ Perceptions of Smart Farming Technologies for Cropping Systems across Europe.” Precision Agriculture 21(1): 34–50.
Klerkx, Laurens, Emma Jakku, and Pierre Labarthe. 2019. “A Review of Social Science on Digital Agriculture, Smart Farming and Agriculture 4.0: New Contributions and a Future Research Agenda.” NJAS – Wageningen Journal of Life Sciences 90–91.
Klerkx, Laurens, and David Rose. 2020. “Dealing with the Game-Changing Technologies of Agriculture 4.0: How Do We Manage Diversity and Responsibility in Food System Transition Pathways?” Global Food Security 24.
Kuch, Declan, M. Kearnes, and K. Gulson. 2020. “The Promise of Precision: Datafication in Medicine, Agriculture and Education.” Policy Studies 41(5): 527–46.
Lajoie-O’Malley, Alana, Kelly Bronson, Simone van der Burg, and Laurens Klerkx. 2020. “The Future(s) of Digital Agriculture and Sustainable Food Systems: An Analysis of High-Level Policy Documents.” Ecosystem Services 45.
Lioutas, Evagelos D., and Chrysanthi Charatsari. 2020. “Smart Farming and Short Food Supply Chains: Are They Compatible?” Land Use Policy 94.
Lioutas, Evagelos D., Chrysanthi Charatsari, Giuseppe La Rocca, and Marcello De Rosa. 2019. “Key Questions on the Use of Big Data in Farming: An Activity Theory Approach.” NJAS – Wageningen Journal of Life Sciences 90–91.
Lioutas, Evagelos D., Chrysanthi Charatsari, and Marcello De Rosa. 2021. “Digitalization of Agriculture: A Way to Solve the Food Problem or a Trolley Dilemma?” Technology in Society 67: 101744. https://linkinghub.elsevier.com/retrieve/pii/S0160791X21002190.
Lowenberg-Deboer, James, and Bruce Erickson. 2019. “Setting the Record Straight on Precision Agriculture Adoption.” Agronomy Journal 111(4): 1552–69.
Lowenberg-DeBoer, James, Iona Yuelu Huang, Vasileios Grigoriadis, and Simon Blackmore. 2019. “Economics of Robots and Automation in Field Crop Production.” Precision Agriculture.
Miles, Christopher. 2019. “The Combine Will Tell the Truth: On Precision Agriculture and Algorithmic Rationality.” Big Data and Society 6(1).
Nguyen, Geneviève, Julien Brailly, and Francois Purseigle. 2020. “Strategic Outsourcing and Precision Agriculture: Towards a Silent Reorganization of Agricultural Production in France ?” ASSA-AAEA 2020 – Annual Meeting of the Allied Social Sciences Association and the Agricultural and Applied Economics Association 2020(January). https://oatao.univ-toulouse.fr/26006/.
Ofori, Martinson, and Omar El-Gayar. 2021. “Drivers and Challenges of Precision Agriculture: A Social Media Perspective.” Precision Agriculture 22(3): 1019–44.
Pallottino, Federico et al. 2018. “Science Mapping Approach to Analyze the Research Evolution on Precision Agriculture: World, EU and Italian Situation.” Precision Agriculture 19(6): 1011–26.
Pathak, Hari Sharan, Philip Brown, and Talitha Best. 2019. “A Systematic Literature Review of the Factors Affecting the Precision Agriculture Adoption Process.” Precision Agriculture 20(6): 1292–1316.
Ravis, Timothy, and Benjamin Notkin. 2020. “Urban Bites and Agrarian Bytes: Digital Agriculture and Extended Urbanization.” Berkeley Planning Journal 31(1): 100–122.
Regan, Áine. 2021. “Exploring the Readiness of Publicly Funded Researchers to Practice Responsible Research and Innovation in Digital Agriculture.” Journal of Responsible Innovation 8(1): 28–47.
Rojo-Gimeno, Cristina et al. 2019. “Assessment of the Value of Information of Precision Livestock Farming: A Conceptual Framework.” NJAS – Wageningen Journal of Life Sciences 90–91.
Rolandi, Silvia, Gianluca Brunori, Manlio Bacco, and Ivano Scotti. 2021. “The Digitalization of Agriculture and Rural Areas: Towards a Taxonomy of the Impacts.” Sustainability (Switzerland) 13(9).
Rose, David Christian et al. 2021. “Agriculture 4.0: Making It Work for People, Production, and the Planet.” Land Use Policy 100.
Rose, David Christian, and Jason Chilvers. 2018. “Agriculture 4.0: Broadening Responsible Innovation in an Era of Smart Farming.” Frontiers in Sustainable Food Systems 2.
Roth, Steffen, Harry F. Dahms, Frank Welz, and Sandro Cattacin. 2019. “Print Theories of Computer Societies. Introduction to the Digital Transformation of Social Theory.” Technological Forecasting and Social Change 149.
Rotz, Sarah, Evan Gravely, et al. 2019. “Automated Pastures and the Digital Divide: How Agricultural Technologies Are Shaping Labour and Rural Communities.” Journal of Rural Studies 68: 112–22.
Rotz, Sarah, Emily Duncan, et al. 2019. “The Politics of Digital Agricultural Technologies: A Preliminary Review.” Sociologia Ruralis 59(2): 203–29.
Ryan, Mark et al. 2020. “The Ethical Balance of Using Smart Information Systems for Promoting the United Nations’ Sustainable Development Goals.” Sustainability (Switzerland) 12(12).
Schewe, Rebecca L., and Diana Stuart. 2015. “Diversity in Agricultural Technology Adoption: How Are Automatic Milking Systems Used and to What End?” Agriculture and Human Values 32(2): 199–213.
Schnebelin, Eleonore. 2021. “Linking the Diversity of Ecologisation Pathways to Farmers’ Digital Use Profiles.” To be published
Schnebelin, Éléonore, Pierre Labarthe, and Jean-Marc Touzard. 2021. “How Digitalisation Interacts with Ecologisation? Perspectives from Actors of the French Agricultural Innovation System.” Journal of Rural Studies. https://linkinghub.elsevier.com/retrieve/pii/S0743016721002205.
Shepherd, Mark, James A. Turner, Bruce Small, and David Wheeler. 2018. “Priorities for Science to Overcome Hurdles Thwarting the Full Promise of the ‘Digital Agriculture’ Revolution.” Journal of the Science of Food and Agriculture 100(14): 5083–92.
Sparrow, Robert, and Mark Howard. 2020. “Robots in Agriculture : Prospects , Impacts , Ethics , and Policy.” Precision Agriculture 22(September): 818–33. https://doi.org/10.1007/s11119-020-09757-9.
Stock, Ryan, and Maaz Gardezi. 2021. “Make Bloom and Let Wither: Biopolitics of Precision Agriculture at the Dawn of Surveillance Capitalism.” Geoforum 122: 193–203.
Stone, Glenn Davis, and Andrew Flachs. 2018. “The Ox Fall down: Path-Breaking and Technology Treadmills in Indian Cotton Agriculture.” Journal of Peasant Studies 45(7): 1272–96.
Tomlinson, Isobel. 2013. “Doubling Food Production to Feed the 9 Billion: A Critical Perspective on a Key Discourse of Food Security in the UK.” Journal of Rural Studies 29: 81–90.
Vasconez, Juan P., George A. Kantor, and Fernando A. Auat Cheein. 2019. “Human–Robot Interaction in Agriculture: A Survey and Current Challenges.” Biosystems Engineering 179: 35–48.
Vik, Jostein, Egil Petter Stræte, Bjørn Gunnar Hansen, and Torfinn Nærland. 2019. “The Political Robot – The Structural Consequences of Automated Milking Systems (AMS) in Norway.” NJAS – Wageningen Journal of Life Sciences 90–91.
Vinuesa, Ricardo et al. 2020. “The Role of Artificial Intelligence in Achieving the Sustainable Development Goals.” Nature Communications 11(1).
Visser, Oane, Sarah Ruth Sippel, and Louis Thiemann. 2021. “Imprecision Farming? Examining the (in)Accuracy and Risks of Digital Agriculture.” Journal of Rural Studies (July 2020). https://doi.org/10.1016/j.jrurstud.2021.07.024.
Whitfield, Stephen, Andrew Juan Challinor, and Robert M. Rees. 2018. “Frontiers in Climate Smart Food Systems: Outlining the Research Space.” Frontiers in Sustainable Food Systems 2.
Wiseman, Leanne, Jay Sanderson, Lachlan Robb. 2018. “Rethinking Ag data Ownership”. Farm Policy Journal, vol 15.
Wiseman, Leanne, Jay Sanderson, Airong Zhang, and Emma Jakku. 2019. “Farmers and Their Data: An Examination of Farmers’ Reluctance to Share Their Data through the Lens of the Laws Impacting Smart Farming.” NJAS – Wageningen Journal of Life Sciences 90–91.
Yu, Peter K. 2020. The Algorithmic Divide and Equality in the Age of Artificial Intelligence.
Zhang, Airong et al. 2021. “Who Will Benefit from Big Data? Farmers’ Perspective on Willingness to Share Farm Data.” Journal of Rural Studies. https://linkinghub.elsevier.com/retrieve/pii/S0743016721002308.
2 thoughts on “Agriculture & Digital: are we really going in the right direction?”