In 1913, Fritz Haber and Carl Bosch developed the Haber-Bosch process to industrialize the production of ammonia, which, in addition to its terrible use in the development of explosives during the First World War, was also used to synthesize nitrogen fertilizers for agricultural fertilization. For some, the massive use of nitrogen fertilizers will have saved humanity from famine after the war. For others, these fertilizers have on the contrary degraded the agricultural soils by mineralizing the humus and by disturbing and unbalancing the life of the soil.
It is clear that today, the subject of nitrogen fertilization is still relevant. Between the efficiency of nitrogen use by plants, which can be further improved, nitrogen losses in the system (leaching, volatilization…) and nitrous oxide emissions, which are severely contributing to climate change, nitrogen management has never been so important.
Digital tools are presented, among others, as one of the solutions to support the reasoning of nitrogen fertilization in agriculture, and this is perhaps one of the most historical applications of these technologies. Technologies, methods and approaches have evolved significantly since their inception and have led to advances in nitrogen reasoning, there is no doubt about that, but this digital ecosystem is still quite unclear to anyone looking into it. The proposed tools are rather uneven in terms of quality, they are not audited, and the underlying models and approaches are not always transparent. Although we cannot put all the ecosystem in the same basket, we must admit that there is still a lot of work to be done in terms of explanation, popularization, and synthesis.
Several structures wished not to appear in the list of interviewees, judging the first draft of my dossier too critical and not nuanced enough. The tone of the dossier has since been softened. I let you judge for yourself. Feel free to share your comments.
This report on nitrogen fertilization is also an opportunity to promote all the knowledge that is beginning to be capitalized on the directory of digital tools for agriculture. In addition to serving as a collaborative watch, this platform is now used to take a step back on the digital tools in place and to identify trends.
As usual, for readers of the blog, this article is based on video interviews with industry players (whose names can be found at the end of the article) whom I thank for the time they were able to give me. Several articles, reports and seminars have allowed me to complete the feedback from the interviews.
Enjoy reading!
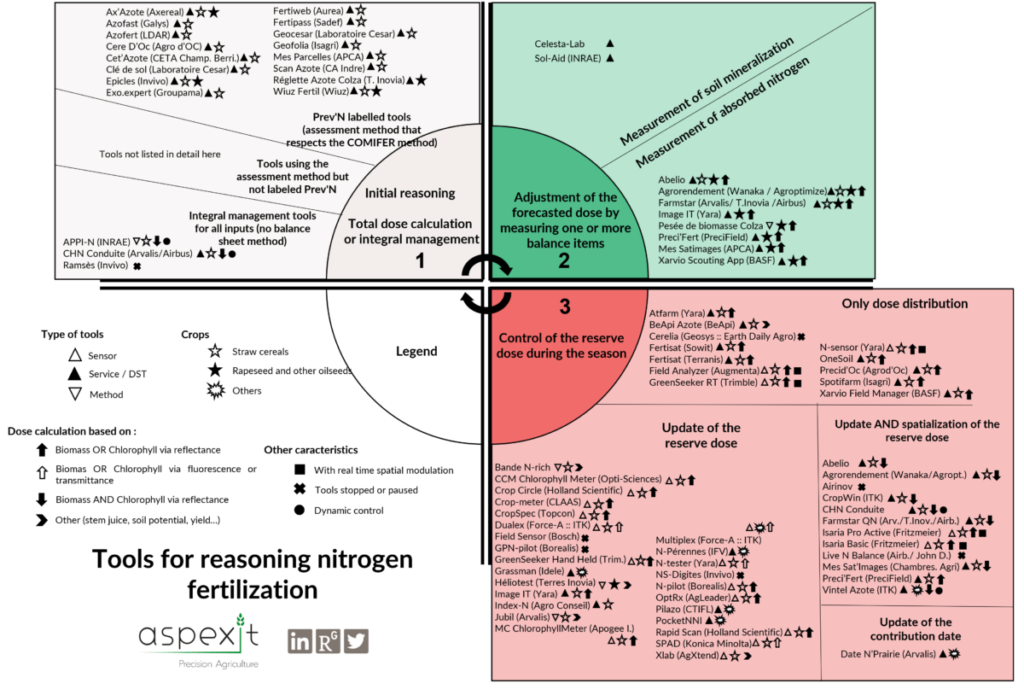
Some reminders (or not) about nitrogen fertilization
Far be it from me to give you a detailed course on nitrogen fertilization – I wouldn’t be able to, I wouldn’t have the legitimacy to do so, and that’s not the purpose of this blog file anyway. The objective here is rather for me to give you a feel for the main concepts and to give you an idea of nitrogen fertilization as I understand it. In this section, we will go back over some of the main principles that you should have in mind and, for those who may have been traumatized by them in the past, we will find it hard not to say hello to the nitrogen cycle… We will try to give it back its noble letters.
A quick look at the nitrogen cycle
It goes without saying that nitrogen, chemical symbol N, is one of the most important elements for plant growth. It is found absolutely everywhere – in nucleic acids (DNA), in proteins (and enzymes of all kinds), or in adenosine tri phosphate (ATP), which provides the energy needed for the functioning of cells. Remind you of something? Chlorophyll, the protein at the heart of one of the most exceptional processes we know of on Earth – photosynthesis – is therefore completely dependent on the nitrogen content of plants for its synthesis. This is why some digital technologies measure the chlorophyll intensity of plants as a way to have access to their deficiencies and/or nitrogen status, we will have the opportunity to talk about it later in this file.
Unlike animals and humans, plants are said to be autotrophs for nitrogen, that is to say that they are able to produce sugars, amino acids or proteins for their food directly from nitrogen. We are totally unable to do this and are therefore completely dependent on plants to produce these elements that we will then ingest either directly or indirectly because they will have been eaten by animals that we will then eat.
When we look a little closer, the main challenge around nitrogen is not really related to a lack, but on the contrary to an excess. Our atmosphere is almost 80% nitrogen, or rather di-nitrogen (N2). So if you’re looking for it, you know where to find it. The difficulty lies in our ability to ensure that this nitrogen, once supplied to the plant in a form it can assimilate, does not leak into the rest of the system – into the soil, water and air – but remains as close as possible to the plant. So keep in mind that just because nitrogen is present in large quantities does not necessarily mean that it is available to plants in those same quantities…
The nitrogen cycle and its underlying mechanisms are highly complicated (Figure 1). Nevertheless, without understanding all of its intricacies, it is important to have a fairly general view of it.
The first thing to keep in mind is that the forms of nitrogen are important (organic nitrogen, ammonia, nitrites, nitrates…). The vast majority of plants are not able to fix nitrogen from the air and plants are not able to assimilate all forms of nitrogen. We agree that it is a pity because it would allow to simplify the nitrogen cycle… Second thing to keep in mind, in the nitrogen cycle, there are nitrogen inputs (fertilizer inputs) and outputs (nitrogen leaks into the groundwater, nitrogen release into the air, nitrogen runoff…). Yes, otherwise it would not be a cycle anymore… And here again, the forms of nitrogen are important because all these inputs and outputs of nitrogen will not make the same forms of nitrogen appear.
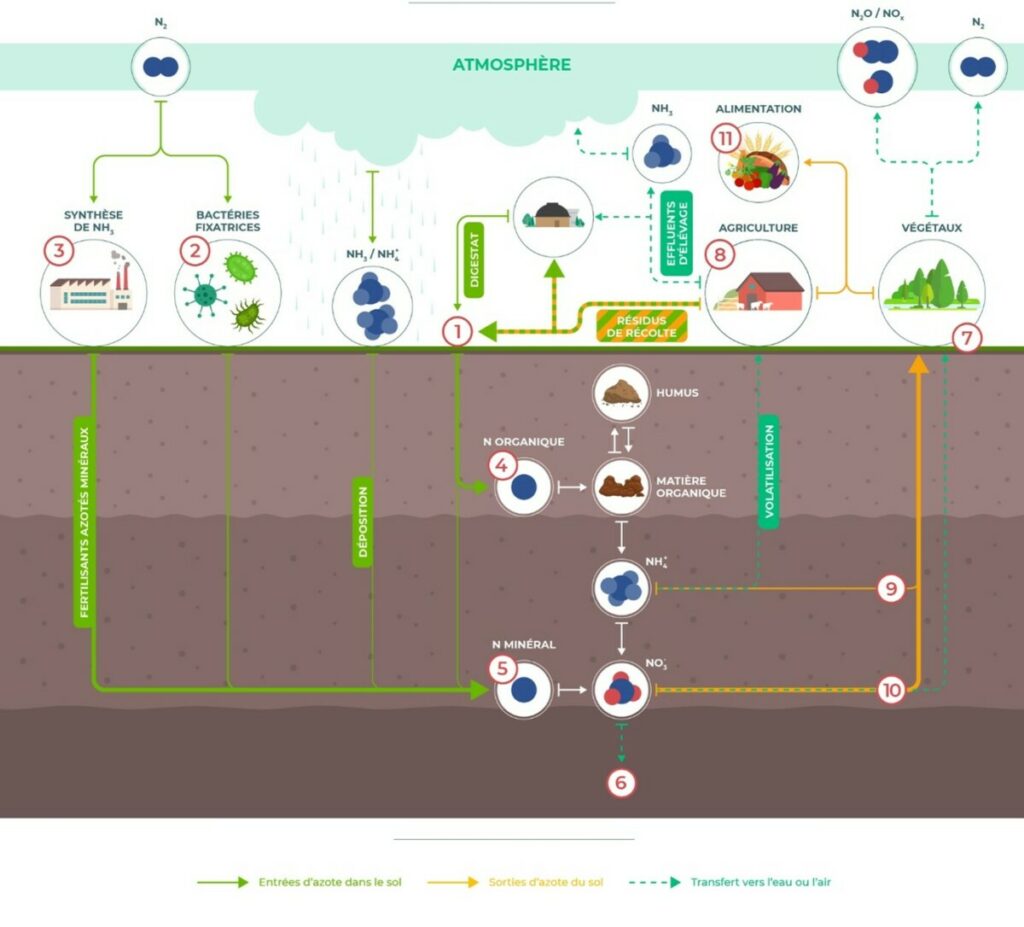
Figure 1: The nitrogen cycle. Source: Info Metha
Plants are able to take up nitrogen in water-soluble ionic forms: ammonium (NH4+), nitrite (NO2-) and nitrate (NO3-). However, the main assimilation is in the form of nitrates. But where does this nitrogen that enters the system and supplies plants with assimilable nitrogen come from? There are many sources:
- By adding mineral nitrogen fertilizers: When the nitrogen in mineral fertilizers is added in its water-soluble ionic form – for example, ammonium nitrate fertilizers which contain a lot of nitrogen in its nitric form – the nitrogen can be absorbed very quickly by the plant. This is the main reason why ammonium nitrate is considered to be the most effective mineral nitrogen fertilizer. For mineral fertilizers that provide nitrogen in other forms (urea nitrogen or ammonium nitrogen), the nitrogen will take a little longer to be absorbed since it will have to go through a whole bunch of chemical reactions – depending on the conditions of the environment – in order to be made available in forms that can be taken up by the plant. In particular, urea will have to be hydrolyzed to ammonium by soil enzymes. In turn, ammonia nitrogen (NH4+) will have to be converted into nitrate (NO3-) by a reaction called nitrification carried out by nitrifying bacteria in the soil. Just note that nitrification actually consists of two substeps: nitritation to go from ammonium (NH4+) to nitrite (NO2-) and nitration to go from nitrite (NO2-) to nitrate (NO3-). Note also that free nitrogen in the soil is mainly in the form of nitrate ions because ammonium ions are rapidly nitrified by soil bacteria (except when the pH is very low). In particular, the war in Ukraine has increased the price of ammonium nitrate considerably, and many farmers have turned to cheaper urea fertilizers.
- By adding organic fertilizers: when you add organic fertilizers (liquid manure, manure, crop residues left on the ground, roots, sewage sludge, poultry effluents, liquid digestates…), you add organic matter which contains organic nitrogen in the proteins of these organic fertilizers. And it will be necessary to decompose this organic nitrogen to make it available for the plants. Who does this? Soil micro-organisms – bacteria and fungi ! We are talking here about mineralization of soil nitrogen because these micro-organisms will break down the nitrogen contained in the organic matter of organic fertilizers and transform it into mineral nitrogen, in particular ammonium (NH4+), which will be able to follow the same chemical reactions as those described in the case of mineral fertilizers. Keep in mind that there is a so-called “primary” mineralization, which decomposes fresh organic matter very quickly, and a so-called “secondary” mineralization, which decomposes the more stable organic matter in the soil (humus).
- By fixing nitrogen from the air: many bacteria and cyanobacteria are present in the soil and participate in fixing nitrogen from the air to transform it directly into an ammoniacal form (NH3) available to the plant and which can also follow a nitrification process. Some plants – leguminous – are able to fix nitrogen from the air thanks to nodules in their roots. These nodosities are a kind of modification of the absorbing hairs of their roots. They are created by the action of symbiotic bacteria fixing nitrogen from the air present in the soil. The amount of nitrogen that can be contributed by these symbiotic and/or non-symbiotic fixations is subject to debate; some people see only a few tens of units of nitrogen (10 to 20) while others would see much higher contributions.
- By the atmospheric deposition of nitrogen: Part of the nitrogen emitted into the atmosphere by the combustion of fossil fuels [nitrogen oxides: nitric oxide, nitric acid, nitrous oxide] and by agricultural activities [in particular the emissions of ammonia NH3 by volatilization that we will review shortly] can be redeposited in a liquid or solid form on terrestrial (and marine of course) ecosystems and be made available again to the plant by nitrification.
- By the contribution of irrigation water: we speak here of fertirrigation when the irrigation water is loaded with nutrients, whether nitrogenous or otherwise. This fertirrigation also allows for fine adjustment of nitrogenous inputs since they are already in ionic forms soluble in water.
Again, remember we’re talking about the nitrogen cycle. Some nitrogen flows into the system – we’ve just seen them – and some flows out of the system, thus lowering the nitrogen content of the system. Again, there are many sources – and sometimes surprising ones:
- By nitrate losses through leaching and runoff. Nitrates are particularly mobile – much more so than ammonium, for example – and when it rains, these nitrates can leak into the water table (this is called leaching) or leak onto the soil surface by runoff especially if the soil is not covered. These leaks are the most widely publicized and are the cause of diffuse nitrate pollution and water eutrophication.
- By the export of crop residues: when crop residues (straw, wood, etc…) are harvested, the nitrogen contained in these residues (we speak of exported nitrogen) also leaves the system and is not returned to the soil
- By the phenomenon of denitrification: we have seen above that nitrifying bacteria are able to transform ammonia nitrogen into nitric nitrogen and nitrates. “Denitrification”, carried out by denitrifying bacteria, is the process through which these bacteria transform nitric nitrogen into gaseous nitrogen, mainly in the form of nitrous oxide – N2O. We will talk about this later, but nitrous oxide is a greenhouse gas with a much higher warming power than carbon dioxide.
- By losses of nitrogen gas during nitrification: the chemical reaction carried out by nitrifying bacteria releases nitrogen oxides (nitric oxide and nitrous oxide) but the phenomena involved are not well known.
- By the phenomenon of nitrogen volatilization: most of the volatilization occurs following the application of fertilizers. A part of the ammonium ions contained in fertilizers is not converted into nitric nitrogen by the nitrifying bacteria of the soil, and is transformed into gaseous ammonia NH3 which dissipates into the atmosphere. As mentioned above, this ammonia can then be redeposited on the soil by atmospheric deposition – ammonia being a very reactive gas. Note that since this redeposited ammonia can be re-deposited through nitrification/denitrification, the IPCC [Intergovernmental Panel on Climate Change] considers that 1% of the nitrogen emitted as NH3 is subsequently converted to N2O through nitrification/denitrification following deposition of this NH3. Volatilization is very dependent on the properties of the fertilizer and the local agro-soil-climatic conditions (it is recommended to bury the fertilizer to limit this volatilization phenomenon). All this volatilization has of course an effect on air pollution (with in particular the discussions and debates around the toxic particles PM2.5 or PM10).
- By assimilation, immobilization or microbial organization: This phenomenon is the direct competitor of the mineralization of organic matter. Plants and soil bacteria are in competition for nitrogen because bacteria need soil nitrogen to degrade soil organic matter (fresh matter and stable matter) and carry out mineralization. Bacteria therefore use part of the nitrogen reserves of the soil to work, and thus immobilize part of these reserves. Note that this nitrogen is not lost and that it is stored in the form of stable organic matter (humus) and can thus be remineralized later (we spoke above of secondary mineralization).
To continue the link between mineralization and microbial organization (assimilation), you may have heard of the carbon-nitrogen (C/N) ratio or balance. When a fertilizer is brought to the soil with a high C/N ratio like straw (thus a fertilizer that contains a lot of carbon and little nitrogen), the soil fauna will be stimulated and will multiply to actively try to mineralize this organic matter brought in rich in carbon. To do this work, the soil bacteria will also need nitrogen, which they will have to find in the soil reserves since the fertilizer contains very little. If all the nitrogen in the soil is consumed or if there is not enough, the soil microorganisms will find themselves in a state of shortage and will have consumed all the nitrogen that the plants could have assimilated. This is the phenomenon of nitrogen starvation. On the other hand, when a fertilizer is supplied to the soil with a low C/N ratio, as is the case with a mineral fertilizer (i.e. a fertilizer with little carbon and a lot of nitrogen), the soil microorganisms will not go looking for the soil’s nitrogen reserves because there is already more than enough in the fertilizer supplied, but will go and modify the C/N ratio. It is therefore not surprising that everything is a question of balance between carbon and nitrogen.
With all that we have talked about, it is important to understand that the amount of nitrogen present in the soil in mineral form can vary significantly depending on the mineralization of nitrogen in the soil, its assimilation by the plants, or even by all the existing leaks in the system. You will therefore understand that a soil analysis at a given time is certainly interesting but also insufficient in view of the dynamics of nitrogen in the soil throughout the season. We have just talked about the C/N ratio and the interest of having a balanced vision of it. Nevertheless, this type of indicator (and others for that matter) is still too often used to give a static account of the overall organic capital of the soil, whereas it is a whole flow and different forms that should be considered (potentially mineralizable nitrogen, stable carbon, labile carbon, free and bound organic matter, etc.).
A macro landscape of nitrogen fertilization status
Nitrogen inputs to crops have increased considerably since the 1960s (Figure 2), although very large inequalities in access persist around the world (the vertical scales in Figure 2 are very different), with Western and Asian countries, for example, consuming far more nitrogen than African countries (many subsistence farmers depend on the nitrogen capital of their soils and do not have access to commercial nitrogen fertilizer).
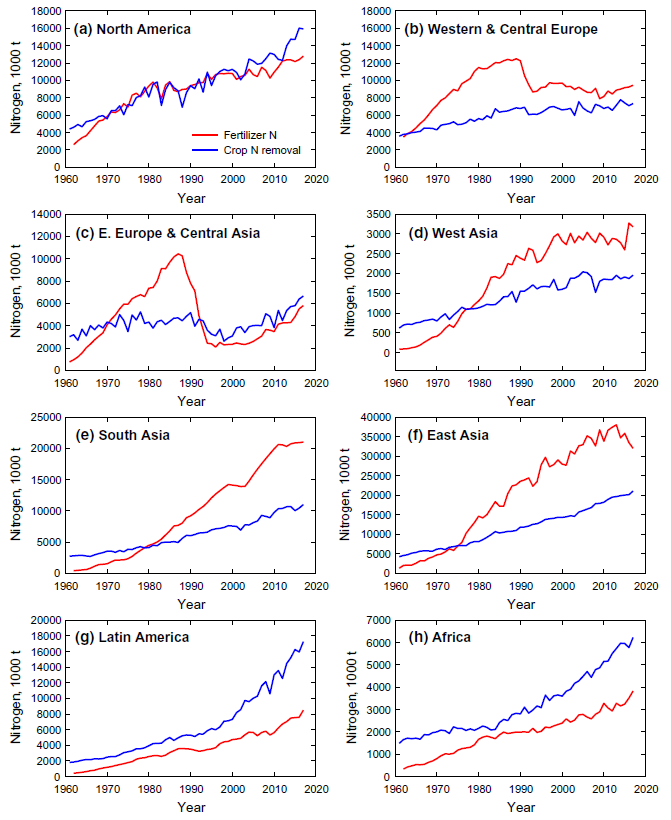
Figure 2: Trends in nitrogen inputs (red) and nitrogen export in crops (blue) by region, 1961-2017. Note that not all vertical scales are the same. The data are national-scale estimates of nitrogen inputs from fertilizer, manure, and biological nitrogen fixation, and nitrogen export from harvested crops. Grassland and forage crops are not included. Source: Cassman (2021) using IFA Nutrient Use Efficiency Database, IFASTAT, 2020
On a global scale, despite the large amounts of nitrogen fertilizer with which we fertilize plants, only a portion of the nitrogen is actually taken up and used by plants upon application (Cassman & Dobermann, 2021) . Put another way, some of the nitrogen delivered to plants is not directly used. Either this nitrogen leaves the plant system and is lost in the soil or in the air, with now widely known consequences (water eutrophication, air pollution etc…), or it is stored in the organic matter of the soil – we will discuss this later. Some authors will even go so far as to say that nitrogen is the next carbon because anthropogenic nitrogen emissions into the environment have been greater than those of CO2 in recent decades. I would like to remind you that among the nine limits of the planetary limit concept defined in 2009 by a team of international researchers, that of the disruption of the nitrogen cycle by human activities has been largely exceeded.
The appropriate term here for this nitrogen uptake efficiency is the Apparent Nitrogen Use Efficiency or NUE.
This NUE indicator varies quite widely around the world and has mainly tended to decrease since the 1960s (from 60-65% down to 45%) and then stabilize even though nitrogen use efficiency is still quite below what is potentially achievable
This utilization coefficient follows the economic development of the countries (Figure 2). The approximation between the red curve (nitrogen input) and the blue curve (nitrogen exported by crops) shows an increase in this utilization coefficient. This is particularly the case in Western countries thanks to better management of nitrogen fertilizers (which increases the relative nitrogen use coefficient), and also thanks, for example, to the high production of soybeans (a legume that fixes nitrogen from the air and therefore requires less nitrogen fertilizers). Note also that Asian countries have greatly increased their consumption of nitrogen fertilizers in the last 30 to 40 years.
In the same vein, Figure 3 shows us that the trajectories of the relationship between yield and nitrogen supply are quite different between the different regions of the world. We can see, for example, that in France, substantial progress was made between the 1960s and 2010. The temporal kinetics in the shape of an “inverted C” reflects an improvement in the nitrogen use coefficient thanks to various changes in practices and methodologies (including the use of digital fertilization reasoning tools).
It is also important to remember that there are biological limits to achieving a very high NUE. Nitrogen is very dynamic and goes through many biochemical processes, which limits our ability to reduce or completely eliminate losses. Climatic and agronomic conditions that are not optimal for plant production are also responsible for low NUE.
Constructing nitrogen budgets (one could make the link to carbon budgets) – especially to know whether the soil is in balance, a sink, or a source – is really not obvious given the difficulty of tracking variations in nitrogen levels in a given soil mass, the amount of nitrogen added to the system through symbiotic fixation, and the losses of nitrogen in the soil-plant system. Taking a step back, however, it is still particularly complicated to relate N use efficiency (NUE) at the plot or farm scale to environmental outcomes at the watershed (sort of global NUE) or groundwater resource scale, due to large gaps in the ability to accurately model crop N uptake, N cycling through soil organic matter, and N losses through leaching and gaseous emissions. While farmers can easily monitor their nitrogen balance, it is much more difficult to estimate an overall NUE because it is confounded with soil N uptake from organic matter mineralization and residual N from fertilizer applications in previous years. However, the NUE is not a component to be neglected; it remains a good criterion for optimizing agronomic practices at the plot level.
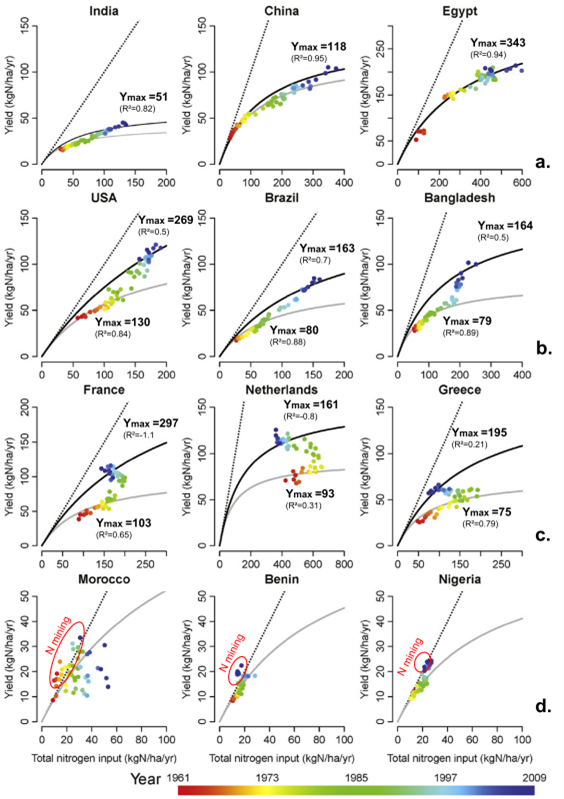
Figure 3: Examples of Yield vs. Nitrogen Supply trajectories followed by countries. (a) Examples of Type I trajectories. (b) Examples of Type II trajectories. (c) Examples of Type III trajectories. (d) Examples of Type IV trajectories. Source: Lassaletta et al. (2014).
How to improve the efficiency of nitrogen use by plants?
The observation is quite terrible: at the global level, the nitrogen supplied is finally quite little valued by plants. Meta-analyses report an apparent nitrogen use coefficient of barely 50% (Lassaletta et al., 2014; Omara, 2019). But how then do we get this indicator up? Although this blog post will still focus much more on the answer provided by digital technologies, we will quickly see in this section that there is no shortage of solutions (other than with digital). Some countries are obviously already implementing some of these practices. The idea here is to give you some background.
In agriculture, fertilizers are mainly delivered to the soil in three different forms: ammonium nitrate, urea, and nitrogen solution. Each fertilizer formulation – regardless of its structure (granules, pellets, powder…) and type (complete fertilizer, starter, blown fertilizer, protected fertilizer…) – has different kinetics and comparing fertilizers is a bit more complicated than just comparing quantities. Ammonitrate – or ammonium nitrate – is considered the most efficient form of fertilizer because it is the most rapidly available to the plant. Urea nitrogen (in urea), on the other hand, needs to be hydrolyzed to be assimilated. As for the nitrogen solution in liquid form, which is still the most used for cost reasons, part of it is quickly volatilized when it is applied if it does not rain quickly (the ammonium NH4 applied is converted into gaseous ammonia NH3, which is released into the atmosphere), thereby reducing the nitrogen utilization coefficient (urea also volatilizes fairly quickly and is more sensitive to volatilization than nitrogen solution). In addition to the cost constraint (urea is also cheaper than ammonium nitrate), it is sometimes environmental conditions that will push the use of a particular fertilizer a little further. In southwestern France, for example, urea will tend to be used more than in northern France because the temperature and heat conditions will favor the hydrolysis of urea to ammonia.
The timing of fertilizer application is also seen as an important area for improvement. We speak of “fractioning” the fertilizer in the sense that it is not applied all at once but according to the physiological stages of the plant, which will make the most of the fertilizer. The most telling example is certainly that of wheat where three to four applications are generally recommended at the early winter stage (wheat does not need nitrogen to grow), ear of corn stage, 2 nodes and the last leaf unfolded stage, which is mainly used to enrich the grain in protein. In viticulture, some will tend to make a last application of foliar nitrogen to influence the organoleptic characteristics of the grapes (we are talking about foliar nitrogen here to make the difference with the more classical fertilizers applied directly to the soil).
Splitting applications allows to spread the risk of exposure to certain losses (volatilization, organization, possibly leaching for some spring crops). It also helps to make up for unfavorable environmental conditions of drought or humidity. In addition to this splitting, the time of application also refers to the time window chosen to maximize the efficiency of the nitrogen, notably by ensuring that the fertilizers can be valorized with rain (hydrolysis, transport of nutrients…) in the days following the application. With increasingly dry springs (and therefore the lack of rain at these times), the use of the nitrogen applied is becoming a thorny issue… We are also beginning to hear talk of burying fertilizers to alleviate the problem of drought, but between the increased work rates and the potential contamination of plots (by weeds), the answer is not completely trivial.
In mineral fertilizers, we are also seeing the appearance of so-called “enhanced efficiency” fertilizers (Li et al., 2018). For example, we speak of coated fertilizers (such as coated urea – coated with sulfur or polymers) to avoid atmospheric losses by volatilization with performances announced as equivalent to ammonitrate, even if the application of these fertilizers raises questions in terms of spreading regularity. Urea is for example much lighter than ammonitrate and therefore poses constraints in terms of ballistics when it is spread especially if it is windy. These coatings allow a more or less slow release of the fertilizer content and thus limit their volatilization (this is also the case for some uncoated fertilizers). There are also fertilizers known as bio-inhibitors. These are not really slow-release fertilizers like coated fertilizers, but rather fertilizers that inhibit the microbial processes that transform nitrogen into forms that can be assimilated by plants and thus transform nitrogen slowly (or relatively slowly) in the soil environment (for example, urease inhibitors and nitrification inhibitors). These bio-inhibitions, especially of nitrification, are nevertheless extremely complex in view of the nitrogen dynamics within the system that change throughout the crop growth cycle (depending on environmental conditions).
We have already mentioned this, but fertilizer inputs could also be better controlled if they were provided at the same time as irrigation – we are talking about fertirrigation – or simply followed by an irrigation to favour their valorisation. However, keep in mind that not all plots are irrigable.
From an agronomic point of view, do we still need to present the importance of plant cover and crop rotations? Plant cover crops in a rotation, especially if they contain legumes, will allow a significant amount of nitrogen to be returned to the soil. Nitrate trap cover crops (CIPAN in French) will limit the transfer of nitrate into drainage water (the phenomenon of leaching that we have discussed in the nitrogen cycle).
Some research is focused on genetics, with several ideas in mind: creating nitrogen-fixing nodules that are independent of plants, reproducing legume nodules on certain cereals, or transferring nitrogenase genes into cereals. These answers, which are extremely technological, will in any case have difficulty being commercialized and made operational before several decades (and obviously raise questions of ethics and our relationship with nature).
Recent work also calls for a reconsideration of the current understanding of N dynamics within the system (Guenet et al., 2021; Thomas, 2022). Contrary to popular belief, it appears that most of the nitrogen not assimilated by plants does not escape from the system (leaching, volatilization, denitrification, etc.), but is instead immobilized in soil organic matter and participates in soil biological activity. This endogenous nitrogen participates in plant nutrition, sometimes in a consequent way depending on the environment, but it is not instantly available for the plants. In the opposite case, when the environment offers little endogenous nitrogen, the nitrogen absorbed comes mainly from fertilizers.
Long-term monitoring of soil nitrogen (with nitrogen traced during the first application of fertilizer) shows quite surprising nitrogen fluxes, with releases of stored nitrogen still 30 years after the application of fertilizer (Thomas, 2022). The dynamics of nitrogen over time, between immobilization and mineralization, would thus appear to be much greater than previously thought. It should be understood that a good part of the nitrogen of one year would come from the mineralization of nitrogen stored in previous years. It would also seem that nitrogen inputs (mineral or organic fertilizers) stimulate these nitrogen flow dynamics and the renewal of organic nitrogen because they would allow the system to be continuously recharged. Frédéric Thomas gives the rather telling image of a train for which the last wagons must be loaded so that the first ones become available (Thomas, 2022). By shifting from a logic of quantities to a logic of flows, and by ceasing to focus solely on the recovery of nitrogen from fertilizer inputs, these phenomena could be better considered. The debate remains open as to whether the renewal of organic nitrogen in the soil is sufficient in itself to meet the demands of crops.
In general, these questions highlight the need to take into account all sources of nitrogen (synthetic, manure/residues, deposition, symbiotic and non-symbiotic nitrogen fixation, soil organic nitrogen, etc.) when designing nitrogen fertilization strategies. The magnitude and variability of these phenomena are obviously site-dependent (soil temperature, soil water status, sunlight conditions…), each parameter influencing positively or negatively the fluxes we have discussed.
Most efforts to improve the apparent nitrogen use factor have focused on the dosis, timing, form, and placement of fertilizer rather than on methods to improve ecosystem functioning to maintain carbon and nitrogen pools that facilitate mineralization and nitrogen supply over time. However, these two considerations are not antagonistic; they are different, even complementary, efficiency levers. Fertilizers must be seen as a more holistic management tool to increase plant nitrogen uptake, decomposition, and carbon supply, and as additional energy for soil microorganisms-for example, to stimulate soil microbial communities to mineralize certain carbon pools more rapidly. Some authors also consider that classically used indicators such as the apparent N use coefficient miss some important factors around N dynamics in the system: the consideration of many different forms of N (and we have seen how many there are), the ability to consider the synchronicity between available N and N demanded by plants, or the understanding of how plants respond to the state of N in the soil (Ladha et al., 2020). The nitrogen use coefficient remains an integrative factor – and this is already very good – but it lacks, by construction, to discriminate more precisely these elements.
Predictive balance method and integral nitrogen management
Nitrogen requirements vary greatly depending on the crop (short cycles versus long cycles, spring crops versus winter crops, straw cereals versus oilseeds, annuals versus perennials…). Nitrogen will not be assimilated or used at the same time of the crop cycle and for the same development needs. The dynamics of nitrogen flows and stocks will therefore differ greatly from one plot and one crop to another. We will take here mainly the example of cereals, but many concepts can be found for other crops as well. Note that nitrogen in perennials is even more complicated to manage because it is not only a matter of estimating annual needs (as for cereals, for example) but also of taking into account the vigor of the stock with the phenomena of storage and remobilization during the season (Verdenal et al., 2021).
In France, the recommendation of a nitrogen dose to be applied to the plots is mainly calculated using a method called “balance”; this means that the inputs and outputs of nitrogen in the system are balanced (I refer you to the nitrogen cycle) in order to arrive at something balanced. The balance equation thus theoretically makes it possible to calculate the dose of fertilizer to be applied so that the balance of inputs and outputs is balanced. We often talk about “the” balance method – especially the COMIFER method – but there are actually several. You may have heard of the soil-surface balance method (of which the CORPEN balance and the global nitrogen balance are examples), which considers the soil-plant system as a black box and is only interested in external fertilizer inputs and outputs from the system by the plant (nitrogen exports). The global nitrogen balance method is more often used in mixed crop-livestock contexts. Then there is the soil-system balance method, which is a mass balance (this is the COMIFER method with a balance of all the inputs and outputs of the system). Finally, there is the method of apparent balances (or mineral balance) which can be seen as an accounting approach to fertilization (we consider the inputs and outputs of nitrogen from monetary flows of input purchases and product sales). This being said, for the remainder of this document, when we talk about balance sheet methods, we will mainly refer to the mass balance method.
It is often referred to as the balance forecasting method (with the objective of calculating a so-called “forecasted” dose or X dose) in the sense that the forecasted dose is calculated at the beginning of the season and therefore the dose to be applied is forecasted in advance. The forecast balance method is used to build a manure forecasting plan (PPF) which is supposed to be carried out by the farmers to reason their fertilization. The forecasting method is interesting because it tries to take into account all the components of the nitrogen cycle. However, it is complex to implement – at least from a theoretical point of view – because it requires the best possible quantification of the value of each of the terms in the balance equation (nitrogen residue at the end of winter, humus mineralization in spring, nitrogen provided by organic inputs, plant needs, etc.). And these terms are all the more difficult to measure precisely as they are dependent on the pedo-climatic context of the plot, and they can vary both in space, sometimes within a plot and in time on very fine temporal scales. It is in fact to respond to in-season management that the majority of digital technologies have been designed. We will talk about this later.
On the side of nitrogen inputs to the system, certain compartments of the balance, especially those related to the soil (such as the mineralization phenomenon), are difficult to evaluate and are often estimated based on values from experiments. Nitrogen residues are important to measure because they give an idea of the state of the soil at the start or end of a cycle, but they do not provide information on the intensity and course of mineralization processes over time. Some advocate the use of potentially mineralizable nitrogen [PMN] to monitor soil mineralization kinetics. The terms for the soil compartments can be subject to uncertainty, and this is particularly problematic when they have to be extrapolated to plots that are different from those on which the experiments took place.
On the side of the system’s nitrogen output, the plant’s nitrogen requirements are often calculated from yield response curves to nitrogen, themselves obtained in experimental contexts. The plant’s needs are thus calculated from a forecasted yield objective (often the Olympic average – average of the yields of the previous five years by removing the yield of the best and worst year) at the end of the season weighted by a factor obtained experimentally. While the approach is relatively simple to implement, it suffers from the fact that first, this nitrogen-yield relationship is highly dependent on other soil nitrogen dynamics and second, other environmental and genetic factors can influence crop yields in addition to nitrogen. The multiple sources of variation can thus lead to a diversity of response curves, which hinders the understanding and predictive ability of these curves. This type of approach, although imperfect, has nevertheless allowed progress to be made in terms of both nitrogen use efficiency and the management of nitrate flows in water.
To reconsider the plant’s nitrogen requirements, some advocate using the nitrogen nutrition index (NNI) – a ratio between the nitrogen measured within the plant and the critical nitrogen below which plant growth is impaired. The NIN would be preferred because it integrates both nitrogen supply and demand by taking into account changes in nitrogen concentration related to plant biomass (referred to as the dilution phenomenon). Note that the critical nitrogen is obtained from a critical nitrogen curve (or dilution curve) for different levels of biomass (the calculation of the NNI therefore requires access to both the biomass and the nitrogen status of the plant). In simpler terms, it is a curve of nitrogen points corresponding to a lack of nitrogen limit. By directly quantifying the nitrogen status of the crop, there would no longer be a need to explicitly consider the availability of nitrogen in the soil. This NNI could also be used in absolute terms, with experimental references, or in relative terms with respect to over- or under-fertilized control strips although these approaches could also be criticized in their ability to effectively ensure over- or under-fertilization throughout the season.
You can also keep in mind that in viticulture, the balance sheet method is not used very often because it is difficult to know the immobilization of the end-of-cycle nitrogen in the reserves. Some reserves will be effectively remobilized from the leaves to the stumps before the leaves senesce. For the moment, viticulture prefers to calculate a dose based on a production objective. In any case, the vine is not driven at its maximum vegetation for reasons of leaf-fruit balance, the idea being to apply a minimum dose to reach the nitrogen response level. Excess nitrogen is anyway detrimental to the crop as well (too much leaf, diseases, over-fermentability of the mouts…) not to mention the environmental problems.
It is therefore important to understand that the method of predictive balances is an a-priori approach, potentially completed by a diagnosis in vegetation with control tools, numerical or not, which we will discuss again very soon. We have just discussed the fact that the precise estimation of mass balance parameters (inputs and outputs) was far from obvious. But agronomically, this balance method also suffers from a few pitfalls: the different terms of the balance equation are measured independently, the interaction between certain cycles (notably water and nitrogen) is not taken into account, the yield objective is often calculated from a rather short yield history and, in the case of wheat, it is often the last inputs that are managed. Despite the limitations that I am raising here, let us put things into perspective by remembering that these methods will have made it possible to make significant progress in the reasoning of nitrogenous fertilization.
In contrast to this method of balances, we are beginning to see the emergence, particularly for wheat, of approaches based on the integral management of nitrogen during the season (particularly the CHN model from Arvalis or APPI-N from INRAE in France). The change of paradigm is important and requires reconsidering the classical vision of nitrogen fertilization. In this integral management, the intention is no longer to reason with a yield objective (on wheat, nitrogen only has an influence on the number of grains and we can therefore question the relevance of a calculation based on a multi-year yield as is currently done with the Olympic average) and a global nitrogen dose to be applied, but rather to have an approach oriented towards diagnosis throughout the crop cycle (end of winter – spring) of the plant’s needs. This integral control thus frees itself from the provisional fertilizer plan in favor of multiple diagnoses/prognosis of the growth and nutrition of the crop in real time. These approaches are based on target trajectories of nitrogen nutrition indices (NNI) that allow for real-time adjustment of nitrogen fertilization management. There are many strategies between minimal or optimal NNI trajectories that allow triggering inputs as soon as the plant moves too far away from the target trajectories, for example because of a lack of nitrogen supply from the soil. Contrary to the so-called static balance methods, this integral control is a dynamic approach where the idea is to take into account in real time the evolution of the system and the external parameters (biomass, water balance, weather…). This integral approach adds that the control is all the more justified in situations where the uncertainty on the nitrogen supply by the soil and/or on the valorization of previous nitrogen inputs is important. These approaches project nitrogen needs over time and simulate all inputs and losses within the system (mineralization, leaching, etc.), unlike balance sheet methods, which tend to correct an instantaneous state.
Oh my god, but is this the ultimate approach to fertilization that we were all waiting for (well, ok, I’m exaggerating a bit…)? Looking through the looking glass, this approach has of course also some drawbacks. The switch to full control does not change the fact that some soil terms are still complex to characterize (again, I’m thinking here of mineralization of organic matter). To be efficient, the model also needs to have access to what was done, or in other words, to everything the farmer did (how much fertilizer was applied, what form of fertilizer, and especially the date of application…). Since these approaches also take into account environmental dynamics, it will be necessary to ensure that climatic forecasts are also of high quality to be sure that fertilizer degradation or leaching or runoff phenomena are correctly taken into account. In short, it is certainly not a panacea, but agronomically it is very solid. We will have the opportunity to talk about all this again when we talk about digital tools.
Some regulatory elements
From a regulatory point of view, you will certainly have already heard of the 1991 Nitrates Directive whose objective is to reduce water pollution caused by nitrates of agricultural origin. These directives are implemented at the territorial level by the regional nitrate expertise groups (GREN acronym in French). Please note that the methodology of the forecasted balance is retained within the framework of the Nitrates Directive and that maximum doses of nitrogen may be recommended. Farmers whose plots are located in vulnerable zones of the Nitrates Directive are obliged to draw up a provisional manure plan (PPF acronym in French). The regulatory dose of inputs calculated in the PPF can be exceeded if justified (by an agricultural advisor or by using a management tool – we will discuss this further).
Perhaps less well known is the NEC directive, which sets emission ceilings, in particular for a certain number of pollutants that may be of agricultural origin (nitrogen oxides – nitric oxide and nitrous oxide, and ammonia off-gases). At the European level, beyond the regulatory tools already in place, it is also the national strategic plans (NSP) of the Common Agricultural Policy (CAP) that will be able to draw more or less ambitious trajectories on nitrogen dynamics within our ecosystems (I invite you to read the sharp criticism of the Environmental Authority on the lack of ambition of the French NSP Agriculture…). Other plans are either already underway at the European level – such as the Farm to Fork strategy of the Green Deal, which sets the objective of reducing nutrient losses by at least 50% while ensuring that soil fertility does not deteriorate – or will be considered in the short or medium term (zero pollution action plan, action plan for integrated nutrient management…).
Digital tools to support nitrogen fertilization
A general overview of digital tools
Nitrogen fertilization is certainly the historical application case in which digital tools have taken an interest. The players in the sector have been busy imagining measurement instruments (sensors) and digital services of all kinds to support the reasoning behind nitrogen fertilization and to propose nitrogen recommendation maps. However, the ecosystem of these digital tools in agriculture is still very vague, as I keep repeating, and the case of nitrogen fertilization is no exception. It is absolutely necessary to centralize knowledge and to clarify what digital tools do and do not do, since the approaches are so different. I’ll say it again here: on the subject of nitrogen fertilization, it’s very clear that the entire digital ecosystem cannot be put in the same basket. Nitrogen fertilization is a case study that is now so democratized that one sometimes has the impression that all the players are addressing it correctly. Far from it….
Digital technologies are mainly discriminated on technical aspects, making a clear distinction between proxy-detection tools (i.e. sensors that perform measurements close to the plant, with a hand-held sensor or on an agricultural machine, for example) and remote detection tools (i.e. sensors that perform measurements far from the plant with instruments mounted on drones, airplanes or even satellites) Even if this distinction has the merit of being clear, it remains (very) clumsy for several reasons. First, digital tools for nitrogen fertilization are not just measuring instruments; they also include services or decision support tools, admittedly using more or less measurements from sensors, but understanding that these tools are different is important. Secondly, this distinction provides absolutely no information on the capacity of these tools to really serve the challenges of nitrogen fertilization. This distinction is technical, not agronomic. Playing on only technical aspects tends to abstract us from the complexity of reality and sometimes prevents us from understanding what digital tools are really able to explore.
In the rest of this paper, I will obviously mention these technical aspects of proxy and remote sensing, but they will not be central. Instead, I will show to what extent the digital tools finally intervene on very specific aspects of the reasoning of nitrogen fertilization, and that these tools on the market are definitely more different from each other than they seem. An initial survey of digital technologies was commissioned by the French Ministry of Agriculture a few years ago (Ministère de l’agriculture, de l’agro-alimentaire et de la forêt, 2016). Among other things, this 2016 report presents fairly detailed worksheets for several digital tools.
In this blog post, I have broken down the organization of digital tools for nitrogen fertilization here into three main families, each re-categorized into several subgroups (Figure 4). The figure is certainly more adapted to field crops (and mainly wheat, barley and rapeseed) than to other crop productions, but I think that the latter will also manage to find their little ones there. You will find :
- Tools for the initial reasoning of fertilization. You will find tools for calculating the total dose at the beginning of winter and those for the integral management of fertilization. The tools for calculating the total dose include mainly include web services or software for making predictive fertilization plans and calculating a predictive dose (or X dose). These tools may or may not have the Prev’N label, awarded by the COMIFER (French Committee for the Study and Development of Reasoned Fertilization). I would like to emphasize that this label does not attest to the quality of the tool as such, but rather to the respect of the mass balance method as described by the COMIFER (I invite you to reread the section on the forecast balance method a little earlier in the dossier). IMPORTANT: I have added here the tools based on an integral nitrogen management. These tools are not designed to calculate a total dose (these approaches do not use the balance method). These integral management tools are not labeled Prev’N. We could have classified these management tools in a fourth category to separate them from those that calculate a total dose, but the idea was to show that all the tools were used from the beginning of the season (to add a temporal dimension to the graph with stages 1, 2 and 3). The integral control tools, as is widely recalled in the dossier, are used throughout the season.
- Tools for adjusting the predictive dose at the end of winter, which mainly include digital services, decision support tools (DST) and smartphone applications for recalculating one of the terms in the predictive balance equation at the end of winter. It is mainly the crop’s nitrogen requirement that is recalculated (at the end of the balance sheet), especially for rapeseed crops, from a measurement of absorbed nitrogen
- Tools for the management of the dose in reserve during the season, which include the vast majority of sensors (pedestrian, machine-mounted, airborne) and decision support services and tools (DSS). These tools are used either (1) simply to update the reserve dose of the balance sheet (these are almost exclusively sensors) by estimating, more or less precisely, the crop’s nitrogen requirement, (2) to update the reserve dose AND to build a spatialized map of the nitrogen recommendation of this dose on the plot (this does not mean that this updated dose or this map will be used in the field), (3) to update the date of application of nitrogen fertilizers, and (4) simply to construct a recommendation map by distributing spatially the initial reserve dose based on an indicator (often vegetation). My use of the adverb “simply” is in no way a disparagement but allows me to be very clear about what the tools do or do not do.
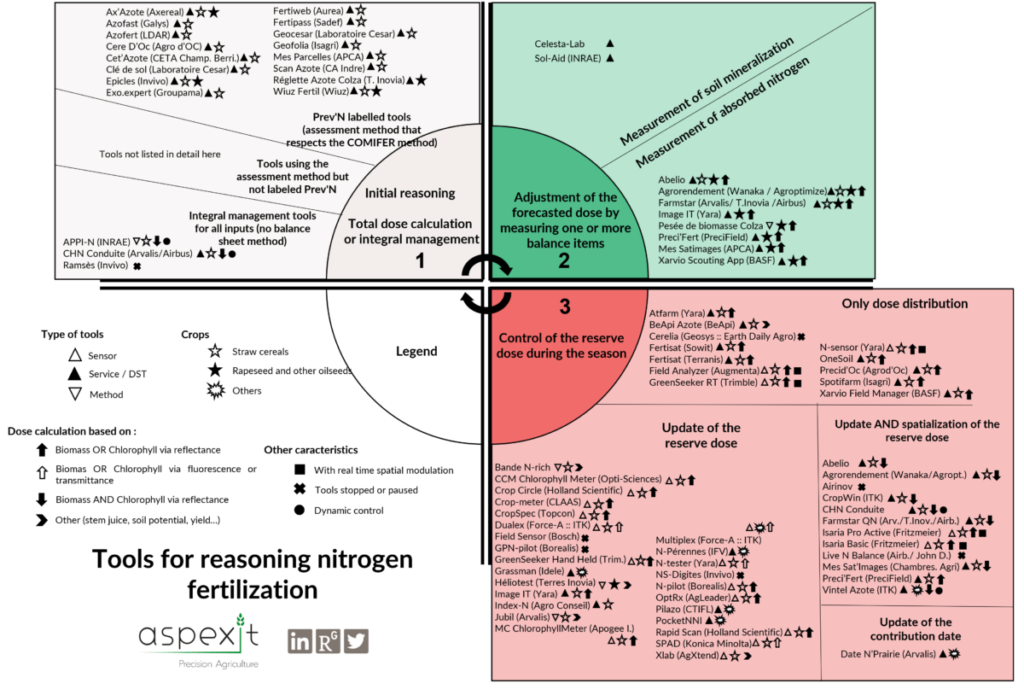
Figure 4. Digital tools for nitrogen fertilization reasoning
I emphasize that these three families are not mutually exclusive, but you may be surprised that some tools appear in some categories and not in others. But that is the reality.
In order not to confuse the reader, I have deliberately not added to the figure the digital tools that can support nitrogen fertilization as a whole, but that are not directly used for its reasoning. One can think in particular of :
- Agro-equipment (section cutting, right-left spreaders, modulation agro-equipment…) and geo-positioning technologies (GNSS, DGNSS, RTK… – I refer you to another blog article) to ensure a fine spatial application of fertilizers. Also note the smartphone application from the Scanopy company to modulate fertilizer application according to tractor speed
- Smartphone applications to help adjust spreaders (e.g. Fertitest [Sulky], MySpreader [Amazone]…)
- Calculators and Swiss Army knives to simplify conversions and dose calculations (e.g.: Fertilising with organic products [Arvalis])
- Digital technologies to apply in good weather conditions and with good timing (examples : Hygo [Alvie], Taméo [Arvalis], all connected weather stations…)
- Traceability tools to track fertilization activities in the field (plot management software, traceability devices… I refer you to another blog entry)
You can find all these tools on the directory of digital tools for agriculture (a little self-promotion is always good)
The following sections go into more detail on the particularities of the approaches of the different digital tools
How to measure the nitrogen requirement of plants with digital tools?
In the context of nitrogen fertilization, the vast majority of digital tools are interested in estimating the nitrogen needs of plants or their nitrogen deficiency. Some tools will try to measure a nitrogen content directly (for example, the “Jubil” method will try to measure the nitrate present in the sap directly – Jubil is more a method than a digital tools but it helps claryfing the situation). Others, on the contrary, will try to estimate it by derived means, relying on parameters more or less strongly linked to the nitrogen content of plants. You may have first thought of chlorophyll, and then you would have been right. The main measuring instruments use the concepts of reflectance, transmittance or fluorescence of the plant to measure a chlorophyll rate. Without going into a detailed physics course, the light that reaches any object (here the plant) will be partly reflected by the object, partly absorbed by the object, and partly transmitted by the object (it will pass through the object). By studying spectra (of reflectance, absorbance, or transmittance) of the plant, we have access to the response of the plant to light. This is particularly interesting since each object has a specific spectral signature.
In particular, the light reflected by the vegetation in the visible region of the electromagnetic spectrum is mainly influenced by the chlorophyll pigments present in the leaf tissue, pigments that have been found to be related to the nitrogen concentration in the plant. Keep in mind that reflectance sensors will tend to measure chlorophyll status at the scale of an entire plant canopy, whereas transmittance sensors will characterize chlorophyll status at the scale of the leaf which is why you will hear the term chlorophyll clamp. Reflectance sensors can be either active (i.e. they have their own source of light energy that they send to the object they want to measure) and others are passive (i.e. they only collect the sunlight reflected on the object to be measured). Chlorophyll clips, based on the principle of transmittance, are considered as active sensors : one side of the clip sends the light, the other part of the clip under the leaf recovers the transmitted light.
The principle of measuring instruments based on chlorophyll fluorescence is slightly different. When a plant is in optimal conditions, it will tend to favor its primary metabolism and synthesize proteins (nitrogenous molecules) containing chlorophyll and few flavonols (carbon compounds). In a state of nitrogen deficiency, we will tend to observe the opposite. The follow-up by fluorescence of the ratio between the content of chlorophyll and flavonols thus makes it possible to follow a nitrogenous state. Keep in mind that, although all three approaches seek to estimate a nitrogen state using all chlorophyll, these methods do not measure exactly the same thing.
By measuring only chlorophyll, we can have access to the current state of nitrogen in the plant but we can hardly predict its future state nor indicate the amount of nitrogen to apply as fertilizer (and nitrogen is not necessarily the limiting factor in production anyway). In reality, it is necessary to understand that this nitrogen state is very dependent on the genotype of the plant, the type of crop (the physiological bases for detecting nitrogen needs are very different between crops with indices linked to the amount of tillering in wheat or to the growth rate in corn), its growth stage, or even environmental conditions. And that, not to simplify the problem, the relationships are often largely non-linear.
Add to this the complexity of using reflectance, transmittance or fluorescence measurements. The measurements provided are not always easy to interpret. The confounding effects are numerous. On water for example: the availability of water influences the absorption of nitrogen by the plant and, consequently, the nitrogen content of the leaves. Still on water, the reduction of cell turgor has an impact on light transmittance caused by variations in intercellular air spaces in the leaf tissue. Several research works (Diacono et al., 2012; Colaço & Bramley, 2018; Munoz-Herta et al., 2013; Padilla et al., 2018) have also shown that the measurements of these sensors were influenced by as many factors as:
- the soil effect (between pure vegetation pixels and mixed pixels that aggregate spectral information from vegetation and soil). Fluorescence and transmittance techniques are interesting to compensate this soil effect,
- the thickness of the leaf (especially for transmittance),
- the time of day when the measurement is made, which always affects evapotranspiration and canopy temperature
- the orientation of the leaves (the wind could therefore also have an influence)
- the irradiation conditions (especially for passive sensors),
- the distance between the sensor and the plant,
- the spatial footprint covered by the sensor (some sensors have a wider view of the plant than others),
- the orientation of the sensor (some sensors have a view from above, others horizontal, others oblique),
- the face of the measured leaf (between for example the adaxial or abaxial face of the corn),
- the location of the measurement on the plant (in vines for example, the nitrogen content is not the same on the leaf or the blade).
- the effect of the edges of the field (embankments, trees…) which influence the measurements close to the outside of the plot with effects of shadow for example
- ….
The most important work is certainly that of weighting the nitrogenous state according to the growth stage of the plant. This stage is usually considered through its biomass, a kind of indicator of the vegetative state of the plant at a given time. Tools that do not estimate the biomass of the plant will necessarily need to calibrate their sensors beforehand with experimental results in real conditions (with regularly repeated nitrogen response curves). Numerical tools that, on the contrary, try to approximate a biomass state by a measurement (mainly reflectance), will generally do so with a leaf index (often the LAI – Leaf Area Index). The combination of biomass and chlorophyll indices will provide access to an amount of nitrogen absorbed by the plant, a nitrogen nutrition index (NNI discussed above) and ultimately the nitrogen status of the plant. Indicators such as the NNI were developed to account for nitrogen dilution processes (the fact that nitrogen and other mineral element levels decrease in plants as they grow) and thus allow for better comparison of crop nitrogen status independent of differences in crop biomass.
I have not mentioned it until now so as not to lose the reader too much, but you may have heard of the concept of inverse radiative transfer models, which are mainly cited for remote sensing services. These approaches try to estimate the biophysical parameters of a crop (LAI for example) from the optical reflectance measured by sensors, which will then be used to build a nitrogen recommendation map. The term “inverse” refers only to the fact that, in the initial experimental models in the field, we know the nitrogen status of the plants and we try to know the reflectance of the plants at that status. We speak of an “inverse” model because the exercise here is, on the contrary, to use the information contained in the spectral bands of the sensor to find a nitrogenous status of the plant. We also speak of radiative transfer in the sense that the light sent by the satellite sensor reacts to contact with the atmosphere, the plant and the soil. It is therefore necessary to correct the signal according to these effects of interference (I let you for example dig the vegetation models SAIL and PROSPECT which seek to model all this). We could very well have inverse radiative transfer models with proxy-detection sensors but in this case, we could (at least) remove the atmosphere model. All these transfer models used then simulate reflectances and the application of cost functions (minimization, maximization…) allows to converge the inverse model and to explain the reflectance spectra obtained. One of the interests of working with inversion models is to be free of the positioning of the bands and the characteristics of each sensor in order to use different satellite constellations.
For reflectance sensors, keep in mind that the vegetation index used (a combination of spectral bands measured by the sensor) can be very different between sensors. The most known vegetation index – NDVI (Normalized Difference Vegetation Index) remains the most used but the spectral combinations are almost infinite (NDRE, TCARI, OSAVI… I let you dive into the corresponding literature). Some indices are potentially more relevant at certain times of the season than at others. For example, some will have more difficulty in discriminating nitrogen saturations at the end of the cycle, and others in discriminating small nitrogen variations. It is nevertheless important to understand that even when the vegetation index used is identical between tools, the value of this index can be different simply because the spectral bands measured by the tools are not exactly at the same wavelengths. All these measurements remain derivatives and it is fundamental to keep in mind the allometric relationships that govern nitrogen fluxes and dynamics within plants and soil.
We have not mentioned electrical methods based on nitrate-selective electrodes or electrical impedance spectroscopy technologies – but these methods are not really emerging from an operational point of view. The measurements would still be too noisy and not precise enough. As far as I know, I have not seen any tools based on isotopic markers of nitrogen either (while in vineyards for example, when leaves assimilate nitrogen, roots are enriched in nitrogen isotope 15 – and the ratio of isotope 14 to isotope 15 changes). I leave it to those who are interested to go and dig into the matter.
Proxy-detection and Remote Sensing
Proxy and remote sensing tools are distinguished by their ability to cover larger or smaller territories at different time steps. The spatial footprint of remote sensing tools (airplane, drone, satellite) is obviously larger and revisit times can also be more regular insofar as the satellites revisit the same place more or less regularly. Proxy-sensing measurements for nitrogen fertilization (smartphone applications, pedestrian sensors, and sensors on agricultural machines) are therefore, in general, more time-consuming than their remote sensing cousins, not only because of the low spatial coverage of these first measuring instruments, but also because proxy-sensing sensors may require a larger number of measurements (several dozen leaves for example in the case of certain transmittance sensors). The differences in spatial coverage also affect the representativeness of the measurement in the sense that the sampling location may be chosen, voluntarily or involuntarily, to orient a nitrogen state measurement in one direction rather than another. Let us also add that the costs are not the same in the sense that remote sensing services can potentially be distributed or shared among several actors in the same territory.
On the measurement as such, I insist on the fact that these sensors do not measure the same thing. We can simply think of the fact that sensors embedded on aerial means generally have a vision from the top of the crop whereas proxy-detection sensors will rather have an oblique vision, on the side, or even from the bottom of the crop. A classical vegetation index will therefore have a tendency to measure the porosity of the plant in proxy-detection and a more global vegetation state in remote sensing. These measurements will obviously be correlated but, again, they will not necessarily characterize the same phenomena and/or processes, especially since the spatial footprints of these tools will be different.
This does not mean that proxy and remote sensing tools are antinomic, they can very well be used in a complementary way. Proxy-sensing measurements can be used, for example, to calibrate a dynamic crop model or to re-parameterize a model locally when a model does not take local parameters sufficiently into account or does not put enough weight on certain model parameters. Proxy-detection tools are also a good way for support or advisory structures to gather farmers in the field and obtain technical feedback (by variety, stage or location), to self-criticize and share results.
Between Distribution and Spatialization
Another important distinction of digital tools for nitrogen fertilization is spatialization and distribution. Don’t think of it as farm machinery just yet, and don’t think of it as within-field modulation. To be clear on the terms, you must see behind the term spatialization the idea of modeling, or at least the fact that there was at one time or another the desire to model a nitrogenous state (again, not all digital tools can be put in the same basket). The nitrogen dose is thus spatialized following a diagnosis at different points of the plot, taking into account the state of the cover.
For the distribution, the practice is simpler and only consists in reorganizing an average dose (or pivot dose) according to a vegetation index (this is the case in the vast majority of cases, but some digital services will rely on other indices – e.g. soil-based indices for example), a sort of distribution of nitrogen around a pivot dose. We are therefore assuming a direct relationship between the vegetation index and the nitrogen status of the plot (I will let you reread the previous section on measuring the nitrogen requirement of plants). In this case, we must agree that we are dealing with an average nitrogen status that is re-distributed in space and not with a measurement of the nitrogen status of each plant at any point in the plot. The distinction may seem fine to you, but it is very important. Distribution and spatialization can both lead to a nitrogen recommendation map but once again, the result of this map has nothing to do with the two methods.
Static and dynamic nitrogen pilots
The majority of current digital tools have been designed to control nitrogen during the season (Figure 4). If we were to go even further, we could say that more than in-season control, it is a control of the last fractioned inputs of the season (especially for wheat). This control could be considered as static in the sense that the technologies with a static approach correct, as it were, an instantaneous state of nitrogen. The forecasted nitrogen dose calculated at the beginning of the season is updated (or not) and, if it is, it is generally only once. This a-priori management (once again because the dose is calculated statically at the beginning of the season) raises questions because it closes its eyes to all the hazards (climatic, biotic…) that could affect the yield and that would arrive after the calculation of the forecast dose. These static approaches do not allow to control the consequences of climatic hazards during the season since these hazards are simply not considered : they have not occurred yet. Keep in mind that these static approaches have allowed (and still allow) progress in terms of nitrogen use efficiency
Without necessarily speaking highly of the more dynamic management approaches (we have already spoken a little about them at the beginning of this blog article, and there are still very few approaches based on these concepts), we can nevertheless understand that controlling nitrogen fertilization for a longer period of time allows us to be less subject to climatic hazards. You could very well retort that there is nothing to prevent the use of static approaches by using digital tools to update the nitrogen status of plants on a regular basis. Certainly, and this is what some tools do, updating yield objectives using vegetation maps during the season (and thus taking into account a frost event at the end of winter for example). But this is not the case for all tools, and if you only use proxy-detection technologies, I wish you luck because it will take you some time to do it on each of your plots. Static approaches will generally use charts to link a biomass and/or chlorophyll measurement with a nitrogen nutrition status (although some will criticize the fact that many tools do not really have access to the nitrogen status of the plant).
Dynamic (or integral) nitrogen management models (such as CHN from Arvalis or APPI-N from INRAE in France) are interesting because they try to simulate nitrogen stocks and flows within the plot system. These models simulate the phenology and physiology of the plant, and integrate the environment (water supply, soil supply by mineralization…). These crop models are a representation of the world. They are by definition imperfect and simplistic. These dynamic approaches are not able to take everything into account (diseases, thermal stress, excess water…) but take advantage of dynamic data such as satellite images to secure the models and offer spatialized recommendations as close as possible to the needs of the plants.
However, I would like to digress here and point out that the spatialization of crop models is important to take into account because many of the phenomena measured and/or modeled can vary both in space and in time, but these variations are not necessarily taken into account. For example, there is nothing to prevent the mineralization of organic matter or the supply of water from being variable within a plot. Considering only one type of soil in the plot is therefore a simplification of the processes involved (some models are beginning to try to take into account useful reserve maps). The spatialization of crop models also raises questions in the sense that these dynamic approaches were initially built to work on a plot scale (one model per plot). If one were to imagine applying these models at the pixel scale, for example (i.e. by changing the spatial support for the model), an adaptation would perhaps be necessary, in particular to better validate the spatialized crop model outputs. In particular, the spatial calibration of the models would have to be organized, and the validation of the model results would have to take into account the fact that some of the input data of the model, as well as the outputs, are not independent of each other – which would require going beyond the classical statistical framework (Pasquel et al., 2022).
Compared to static models, these dynamic crop models can be queried at any time since they project the plant’s nitrogen requirements over time. These models are therefore no longer dependent on the acquisition of a satellite image at the right time (at the right stage of development, without clouds, etc.) and will make it possible to control all nitrogen inputs (I remind here for example that on wheat, the majority of the current digital tools control mainly the last inputs). For the moment, the user queries the model when he/she wishes and is left to judge the most appropriate time windows for the application of nitrogen fertilizers. However, the triggering criteria would already include thresholds of expected precipitation based on forecast climate data. In the long term, the objective will be to be able to constrain the model on development stages in order to optimize the efficiency of nitrogen use even more. By doing away with the forecast balance and yield objectives – which in reality only work for average climatic years since it is often the Olympic average that is considered – the dynamic approaches always get a little closer to what actually happens during the crop season. Nevertheless, it is clear that the end-of-cycle climate risks, which are beginning to be more and more recurrent with the current climate deregulation, are difficult to apprehend. Climate uncertainty is important, because the models are very complex, and these uncertainties are necessarily reflected in the models that use them. But can we really do much better with our current knowledge?
Let’s also add that one of the main assumptions of static forecasting approaches (especially for wheat) is that we try to maintain a non-limiting nitrogen nutrition throughout the cycle. And it is exactly with the same mental scheme that digital control technologies work during the season: the objective is to feed the plants so that no nitrogen deficiency appears. Dynamic approaches allow us to break out of this paradigm by allowing us to deficiency wheat as long as it does not affect its yield potential (and research has shown that wheat can be deficient at certain physiological stages without any problem). Lower Nutrient Nutrient Indices (NNI) at the beginning of the cycle allow, for example, to avoid a surplus of ears.
Crop models are extremely numerous (CHN, APSIM, STICS, DSSAT, Ceres-Maize…). These models allow to formalize knowledge and to understand (by inverting them, by optimizing parameters) the determinism of a function or a parameter. These mechanistic models can be used for recommendation (we have shown the example with CHN-Conduite) but these models are complicated to set up for two main reasons. The first one is the difficulty to parameterize these models. These models are dataphages, they generally need several dozens of input variables (climate, soil, response of the plant to its environment, phenology, varieties…). The connection to plot management software or to existing databases certainly facilitates data entry, but in any case questions the capacity to retrieve fine data (at least on the scale at which the prediction is supposed to be made). The second constraint, intrinsically linked to the first, is computational. Running mechanistic models on very large data sets (because they are highly resolved spatially and temporally) will necessarily require finding tricks (cutting the plot into homogeneous zones to avoid working on media with too high a resolution) or degrading the quality of the model (by limiting the input data) to make the tool operational.
Mechanistic and statistical models
There have always been arguments between supporters of mechanistic and statistical models, although the answer may be somewhere in between, with hybrid approaches that combine system modeling (mechanistic) with regularly updated data and simplified relationships (statistical). We have already talked about it, but some numerical tools propose a nitrogen control based on rather simple statistical relationships – for example a direct linear relationship between a biomass or chlorophyll state and a nitrogen state of the plant, a relationship that would have been observed in experimental conditions. And this relationship can be all the more dangerous (because it necessarily considers nitrogen as a limiting factor) if the tool is not calibrated by variety, by type of soil, or by physiological stage (some tools will be calibrated beforehand, while others will be calibrated in real time as data are collected – we are thinking, for example, of certain onboard sensors).
Some statistical models will go further by updating, for example, yield potentials during the season based on historical vegetation maps (and empirical relationships between biomass and yield) by taking advantage of regular updates of satellite data; the idea being to overcome one of the limitations of the forecasting method, which is based on a yield objective calculated at the beginning of the season based on an Olympic average (with a yield objective that is therefore not updated during the season) Still others have preferred to reconstruct yield response curves to nitrogen from yield history and crop data (stages, biomass, etc.) using neural network approaches over several seasons.
Mechanistic models could be criticized for trying to model a system in a clean way so as to generalize conclusions, whereas N stock and flux dynamics are extremely site-dependent (and also vary in space and time). Indeed, some research argues for challenging the idea that agricultural decisions should follow pre-established mechanistic frameworks in which digital technologies are only used to locally estimate specific attributes used as inputs to mechanistic models; in a sense to locally calibrate mechanistic models (Colaço & Bramley, 2021). Given the complexity and variability of the relationships to be modeled, some authors advocate instead multivariate approaches based on data from several different sensors.
Tactical and Strategic Piloting
Finally, one could also have fun distinguishing between approaches that are in the order of the farmer’s nitrogen strategy (i.e., a projection of the farmer on his plot and what he wants to do with it, with a strategy of dose, product or even date of intervention) and tactical management approaches that must be understood as an adaptation of the strategy according to the year’s hazards (climatic, biotic, human, etc.), a sort of adjustment of the forecast. Generally speaking, we could say that the contribution of digital tools, particularly technologies based on canopy reflectance, tends to be on the side of tactical management of nitrogen fertilization. The technologies are effectively used to distribute or spatialize a forecasted dose (updated or not) and ultimately do little to rethink the farmer’s fertilization strategy.
Nevertheless, digital tools – be they sensors, services or decision support tools (DST) – all internalize a lot of assumptions and in fact all have a vision of what nitrogen fertilization should look like. Some, for example, will seek to achieve yield targets with the least amount of nitrogen possible. Other tools will focus on either balancing or maximizing yield and will make nitrogen recommendations accordingly. Some technologies will increase the doses applied to areas with high biomass, considering that these areas are the most fertile and should be pushed to their maximum, while others will seek to reduce the inputs to these areas, considering that their nitrogen status is not deficient. Others will seek to manage fertilization with a technical or technico-economic optimum (which is increasingly questionable with the price ratios between grain and fertilizer following the increase in fertilizer costs). Others will rather consider having a homogeneous biomass and a stable protein rate on the plot. In short, even if the farmer will obviously have control over his fertilization strategy, it must be kept in mind that the recommendations made by the tools are not neutral and that these technologies underlie assumptions and methodologies that everyone must try to understand. This is why the use of several digital tools in the same context may result in significantly different recommendations (we will talk about this later).
Let’s try to take a step back
What to think about the management of nitrogenous fertilization by digital tools?
One might have expected that the sudden rise in fertilizer prices following the Russo-Ukrainian war would have led to a substantial increase in demand for digital control tools. This is obviously not what most of the interviewees told me. Maybe the price of nitrogen is not yet high enough (I might go into hiding after writing this) or maybe there are other reasons (and there are plenty of them) that do not yet encourage the use of digital tools to manage nitrogen fertilization. The price of nitrogen fertilizers will still have more than doubled between 2021 and 2022. The effects are perhaps still too recent to see real trends, or even weak signals. Patience and length of time are more important than strength or rage. To be continued in the coming months….
A look at the logistical challenges
Although nitrogen fertilization is the subject most often dealt with in the field with digital tools, it cannot be said that all farmers are fond of them. One explanation could be logistical issues. Although some sensors and digital services have integrated agronomically sound statistical and/or mechanistic models, their operational use in the field is not optimal (even if all are making efforts in this direction) And that’s maybe where the problem lies. The services are not end-to-end enough and do not necessarily integrate the operational constraints of the farmer who does not necessarily want to spend time converting, loading or transferring data. The diversity of data formats sometimes hinders software and services from communicating with each other and providing nitrogen recommendation maps directly on agricultural machines (I refer you to another blog entry on interoperability and data standards in agriculture). Some manufacturers have nevertheless made their sensors directly compatible with spreaders, especially for sensors on board machines that offer real-time dose modulation. With the Isobus standard, it is indeed possible to work and communicate directly via a console in the tractor between the on-board sensor and the spreader attached to the tractor. In these cases, the on-board sensor can take control of the implement attached to the machine. In addition, some tools require farmers to subscribe to receive updates of the developed models into the sensors (e.g. to update varieties), which again can generate logistical problems.
Whether by distribution or spatialization, most digital tools allow the generation of nitrogen recommendation maps. However, it is still necessary to be able to understand the maps and to be equipped with modulation equipment to be able to make the best use of them (among the farmers who receive recommendation maps, few actually use them to spatially modulate their nitrogen fertilizer inputs). Spreaders are certainly more and more precise (reduction of splitting/overlapping, improvement of the restarting of spreading layers, management of field edges, right-left modulation…) but these spreaders have a significant cost. Some operators propose to simplify modulation (for example by simply modulating with the tractor’s speed of travel using a smartphone application). Other smart guys won’t bother either and will print a modulation map in paper format and use the geolocation of their smartphone to modulate manually.
Some in-season monitoring services – notably those based on satellite imagery – are criticized for providing advice too late, sometimes well after farmers have applied fertilizer (which does not necessarily make them very happy). In addition to the organizational improvements that this type of service could incorporate, we discussed that tools based on dynamic steering approaches could help to address this logistical issue. Since these dynamic tools project nitrogen requirements over time, these technologies are no longer dependent on satellite data at a specific point in time and could therefore deliver recommendation maps at a later point in the season. Note that this does not prevent this type of service from having logistical limitations, depending on the company….
Being a bit prickly, one could nevertheless wonder if the fact that the advice sometimes arrives a bit late in the season is really a problem. Receiving a service much later than requested is certainly annoying, but the question to ask is whether the request is agronomically sound. Farmers are looking for advice early enough to take advantage of rainfall to make the most of fertilizer applications in the field. Looking at rainfall records, one can sometimes see that April/May is not necessarily drier than February/March and that farmers’ fears may be unfounded. And the physiological stages at which crops can still receive valuable nitrogen are not necessarily past when the advice arrives, even if late. With climate change, however, the cards could be reshuffled.
To come back to dynamic models, these approaches are very solid agronomically but raise real operational questions as to their use in the field (and static approaches are already having trouble spreading significantly over the territory, so if we start to move on to dynamic approaches…). To be efficient, dynamic approaches need to integrate the farmer’s realizations, i.e. to know when, where, and how much nitrogen fertilizer the farmer has applied to his plots. The models are not able to know what the farmer has already done as an application. They do not know whether the inputs have been valorized or not by the plant (the models generally make the assumption that all the nitrogen provided has been valorized – without taking into account the residual stocks that have not yet been valorized, which is not really acceptable). When we see the current difficulty of plot management software to collect qualified data, we can really wonder if farmers will take time to provide this information if everything is not fully automated. And it would also imply that the farmer fills in his traceability in time to feed the model in real time, gloups… The data entry remains anyway problematic even for the current tools of piloting during the season. The plot data to be entered in order to obtain a provisional fertilization plan are not negligible. Nevertheless, one may wonder if it is not important to spend time on it to have quality fertilization plans.
The power of crop models also lies in their ability to optimize all nitrogen fertilizer inputs on plots according to crop development and environmental conditions. These dynamic approaches are thus able to propose a division into several fractional contributions (in greater number than is currently carried out in the field) – and this for different plots, and varied precocity – to ensure that the nitrogen status is controlled as finely as possible. Dynamic models will therefore have to be restricted or constrained to fit into feasible logistical organizations, and it will thus be necessary to ensure that, under these constraints of reduced complexity, dynamic models are still of interest compared to other approaches that are simpler to implement. Nevertheless, the interest of dynamic models is sometimes not so much in the advice as in the evolution of the advice over time, which allows the farmer to better understand and anticipate what is happening in order to organize himself.
Digital tools labelled but not audited
You will certainly be surprised to learn that digital fertilization management tools are not audited for the moment (some of them have been tried and tested for quite a few years now). However, some of them have been tried and tested for quite a few years now, and all of the digital technologies shown in Figure 4 cannot of course be lumped together. The tools for calculating the forecasted dose at the beginning of the season may or may not be labeled by the COMIFER (French Committee for the Study and Development of Reasoned Fertilization – we have talked about the Prev’N label) but this label only indicates whether the method used respects the framework of the COMIFER balance sheet method and not whether the results are legitimate. As for the tools for adjusting the dose at the end of the winter or the tools for steering during the season, there is simply no audit – either for the tool itself or the underlying method. And if you have read the second major section of the blog post, you will have seen that the approaches behind these digital tools are quite different, to say the least. At the regional level, each regional nitrate expertise group (GREN – French acronym) sets its own regulations and potentially the digital tools that can be used to comply with the Nitrates Directive. Since the tools are not audited, it is legitimate to ask questions. COMIFER has launched a working group to address the issue.
I can’t help but add a small comment about the complexity of the nitrogen fertilization reasoning tool ecosystem. As you can see from the general infographic (Figure 4), it is hard to know where to start. The list of Prev’N labeled tools is already very large (and will quickly grow) and actually contains many services that are actually the same (or almost similar) – some companies have wished to re-propose a service under their name. Pedestrian and embedded sensors are no exception either, as some dealers and/or tool providers will resell white-label technologies under a different name. The desire of the players in the ecosystem to differentiate themselves (especially in terms of their marketing methods) is legitimate, but sometimes it makes understanding the reasoning behind nitrogen fertilization more complex than anything else.
Between cost and return on investment of these management tools
If the costs of digital services are affordable (this does not mean that their price/quality ratio is satisfactory, I leave you alone to make your choice), the same cannot always be said of field measurement instruments. All kinds of pedestrian sensors generally require several hundred or even thousands of euros and the on-board sensors can cost up to several tens of thousands of euros. Farmers on the lookout will have been able to take advantage of some subsidies, including payments for environmental services and for niche crops, to purchase some of these materials.
In general, the return on investment of digital tools is not well considered. Most actors present a rather simplistic calculation of an economic gain in yield or a reduction in input costs. The cost of the technology is often given little consideration, yet it is not negligible. It is already the cost of the tool or service itself, of potential subscriptions or updates, of repairs, or even of the additional need for modulation equipment or other material necessary to use the digital tool. But it is also an investment in time (setting up the tool, managing bugs and daily use) and potentially in training (farmer himself, farm worker…) that will also require money. A solid economic analysis should take all these criteria into account to assess more seriously if the economic return of these digital tools is there.
Digital control concentrated on only a few crops
It must be noted that, in the context of nitrogen fertilization, the use of digital tools to manage crops is very unequal. It is mainly straw cereals (durum wheat, soft wheat, hard wheat, barley) and oilseeds (especially rapeseed) that are under the scrutiny of digital technologies. This inequality of representation can be generalized to all digital tools for agriculture (especially if we add vineyards).
Given the agronomic complexity of these approaches, dynamic nitrogen management models are currently parameterized for certain very specific crops (for example, the CHN model of Arvalis is currently only interested in wheat). Some crops such as grapevines are still poorly represented because the models are not able to correctly consider row and inter-row effects. Integral control is still in its infancy for rapeseed. In addition to the financial means that are still mainly oriented towards wheat, oilseed rape remains a complicated crop: the optimal fertilization can vary from 0 to 250 units of nitrogen, the levels of growth and absorption of nitrogen can vary from several tens to hundreds of units, oilseed rape loses its leaves during the winter, oilseed rape is a more or less indeterminate species with compensatory faculties in its elaboration of the yield, and the stand is relatively not very homogeneous within a plot. Experimental references are still lacking and there are no quick enough measurement tools to estimate the nitrogen nutrition index (NNI) of rapeseed. The objective is, in the same way as for wheat, to establish NNI target trajectories and to position oneself according to them. For sunflower, too, few tools have been developed to manage nitrogen fertilization, perhaps mainly because sunflower has a strong capacity to explore the soil and is easier to find nitrogen, which means that it needs less fertilizer. If it were possible to easily characterize an NNI on sunflower (to go further than the current simple Heliotest method based on the nitrogen strip principle), it would also be possible to dynamically control the crop’s fertilization.
And as these models are complex, they require time to be adapted to various production contexts (cold and humid sectors, others hot and dry), and to integrate rotations (with legumes and protein crops…). Adapting them to other crops is devilishly complicated (and will require long agronomic work to correctly consider the physiology and allometric relationships of these other plants).
It must be said, however, that nitrogen requirements are quite varied between plants and it is understandable that digital tools for fertilization management have focused on crops with the highest nitrogen requirements. We could also add that work on new crops is also conditioned to some extent by the fact that these crops have an outlet and that nitrogen management is sufficiently profitable (for the moment from an economic point of view, but we could hope that it will also be profitable from an environmental point of view if the cost of negative externalities is also taken into account). Some actors are willing to move forward on nitrogen management on grassland if the market is sufficient. Grassland remains a complicated subject with the diversity of practices applied (permanent, temporary, mowed-grazed, only mowed, productive, only for extensive breeding…). On spring crops, cycles are short and farmers sometimes put a large part of the inputs at the time of implantation, leaving little room for management. Perhaps we sometimes have too much of a tendency to reason in terms of surface area and crop rotation and not take a little more distance on the subject.
Digital tools for reasoning nitrogen fertilization are mainly applied to homogeneous crops. I am not saying here that the digital services are simplistic, some of them based for example on inverse radiative transfer models require very solid skills in agronomy, signal physics and image processing, but the digital tools are for the moment within relatively simplified agronomic frameworks. For example, the presence of companion plants is complicated because the satellite will not distinguish between the main plant and the companion plant (and this is therefore a problem if we want to control the nitrogen of the main crop) and it is difficult to avoid taking into account the relative abundance of companion plants in the canopy. Questions also arise with lower altitude flights (drone, airplane) but perhaps in a different way (even if the discrimination between the main crop and the companion crop is not necessarily obvious) in the sense that the companion crops also return part of the nitrogen to the soil.
In the same way, varietal mixtures are starting to become more widespread in agricultural practices, but the models are not easily able to take them into account because they are based on phenology. If two varieties do not have the same phenology and sensitivities, there may be stage shifts and the result of the modeling could be significantly altered. However, working on other crops would allow us to have access to references that are currently not well known. For crops of interest, the yields are generally known, so the nitrogen absorbed and/or the carbon stored can be known. On the other hand, on non-valued crops (CIPAN, self-consumed grasslands), it could be interesting to know a little better what is happening. Keep in mind that work is underway to begin incorporating this diversity into digital nitrogen reasoning technologies.
What do digital tools compare with?
If we want to judge the relevance of a digital tool for managing nitrogen fertilization – whatever the angle we are trying to analyze – we must necessarily be able to compare it to a reference. But what is this implacable reference? Does it really exist? One can legitimately ask the question. Is it a measurement in the oven and a measurement of stem juice? Is it an over-fertilized or under-fertilized control strip in the plot to estimate the response of nitrogen depending on the plot and the season? Is it a microplot or wide strip experiment to place in a farmer’s production context? If the reference rate tends to be a high nitrogen rate, is it normal to be satisfied with that reference?
One of the advantages of digital modulation tools over traditional methods (microplot experiments and laboratory monitoring) is that they allow modulation of nitrogen inputs according to the spatial variability present in the plots. Conventional randomized complete block experiments generally do not allow the true value of modulation technologies to be assessed because they assume that the effect of spatial variability has been neutralized by randomization in the experiment. The analysis typically performed in these experimental settings ignores spatial variability and/or assumes that it is part of the random error of the explanatory models derived from the experiment. Under these conditions, using numerical tools, the results would really only reflect the benefit of modulation tools over short distances and would miss the opportunity to evaluate the benefits of modulating N inputs over larger scales. One might add that the use of test strips or microplots raises questions about the spatial coverage of some on-board sensors (for example, the coverage is quite large for sensors such as N-Sensor). In a more commercially realistic scenario, variabilities are present both over short distances and over much longer ones. If modulation is not properly evaluated, then its value may be underestimated.
Some experiments take advantage of the use of on-board yield sensors to evaluate the interest of nitrogen modulation on straight line test strips in the field. While the approach is interesting, the resulting data are often analyzed strip by strip (and even sometimes aggregated by strip – for example, yield and nitrogen treatment are aggregated), often for reasons of simplicity or sometimes for reasons of lack of expertise in spatial data analysis. Again, the value of digital tools may be underestimated because spatialized data are not used to their full potential. More complex test strip designs than unmodulated straight lines (in a grid or other way) would allow even more evaluation of the value of digital tools for N fertilization management.
In general, the use of these spatio-temporal data requires a rethinking of the way experimental results are analyzed. Classical statistical tests, which are not made to deal with spatialized data (which by definition violate the principle of data independence), can no longer be used as they are. Nevertheless, the complete exploitation of these spatialized data at high resolution is particularly difficult as the effects are so varied (spatial and/or temporal autocorrelations, anisotropic autocorrelation [oriented in the direction of machine passages]…). The high resolution of these data imposes an important computational demand and the analysis tools are lacking to consider this complexity (for example, it is necessary to redevelop own functions because the existing functions under R or Python are not sufficient).
It is also necessary to pay attention to the confounding effects of the experiments in order to be able to correctly discriminate the contributions of the different means of action (time of application, optimization of the nitrogen dose, modulation). Experimental designs and treatments must not only allow a fair comparison with current practices, but also distinguish the value of the information from the value of the digital tools themselves.
The response of crops during the season is uncertain depending on all the variabilities (climatic, biotic etc…) that are likely to occur. These uncertainties can lead farmers to protect themselves by applying excess nitrogen fertilizers. Conceptually ignoring these notions of uncertainty and risk aversion of farmers may lead to overestimating the value of digital tools and/or underestimating the level of subsidies needed to encourage the adoption of these technologies. One might ask whether the same benefits could be achieved if the farmer simply reduced applied N rates (and therefore assumed a higher risk of under-fertilization) instead of adopting a digital fertilization management toolkit. In this case, would the relative advantage of digital tools still be there?
Traditional microplot experiments are interesting for generating agronomic knowledge. Some consider that, from an operational point of view, these experiments may lack credibility if they do not take into account operational constraints, equipment and farmers’ practices (and microplot trials are, for example, too small to be monitored by satellite), making the results difficult to extrapolate to production contexts (for example, one would expect nitrogen use efficiency levels to be better in microplots than in real conditions). One branch of the scientific literature is beginning to turn to the concept of On Farm Experiments (OFE) to overcome these limitations. Nevertheless, the realization of trials directly in plots, and this directly by the farmers, is not completely obvious either. The design of the experiment must already be thought out in advance, taking care, for example, to ensure that the width of application is a multiple of the width of the harvesting equipment so as not to mix the results between different treatments. The sensors must be calibrated correctly to ensure that the experimental data are reliable and of good quality. It will be necessary to think of the experimentation on the long term and not to reuse the same test strips every year (whether they are over-fertilized, under-fertilized, or modulated) at the risk that the results of the following years will be disturbed by recalcitrant inter-annual effects linked to different fertilization practices. And the experiments will require several trial strips since the strips themselves contain variability. We should also consider that farmers, in testing these modulation approaches, are also experimenting and doing research. Some seize the opportunity to implement innovative practices, already experimentally validated in microplot networks but yet they are given relatively little (if any) money for this, even though they are taking the risk of having a detrimental effect on their production.
If you ask several digital service providers – or if you use different sensors – the recommended dose or the nitrogen recommendation card you will receive will not necessarily be identical. And sometimes significantly different (with similar zonings but reversed application rules), even when the dates of acquisition of the measurements are the same. These differences raise questions and can undermine confidence in the use of this or that digital tool. We have already discussed this, but you should not be surprised. The fertilization strategies inherent to the tools may be different, the calibration of the subjascent models has not been carried out under the same agro-pedo-climatic conditions, and again and above all, none of these tools is audited. It is through field experience, knowledge of the plots and your agronomic knowledge that you will be able to correctly compare the tools. I would also like to add that it is not because the interpretation of a service provider is good in one year that it will necessarily be good in the following years. Soil and climatic conditions change from one year to the next and the agronomic models of the service provider will not necessarily have been parameterized over sufficient ranges of variation.
Unsurprisingly, we will always have to deal with the problem of the representativeness of the measured data – all the more so when the spatial (and temporal) coverage of the digital tool is small (we are thinking first of all of proxy-detection tools). The sampling strategy is therefore particularly important and we have seen earlier in the dossier all the factors that could influence field measurements (see the section on nitrogen measurement by digital tools).
One of the major challenges will also be to ensure that the data collected is truly reliable and of high quality, verified and homogeneous (with well-calibrated sensors because they can sometimes drift over time). The parameterization of digital services (nitrogen balance method, static and dynamic models…) with not very precise data will necessarily impact the quality of the nitrogen recommendation. And in France, the parameterization is all the more important as we tend to over-fertilize. Entering a superficial clay-limestone soil instead of a clay-silt soil can cause the proposed nitrogen doses to vary by several dozen units, and this is all the more worrying as many plots contain different soil types (it is therefore necessary to potentially separate or cut up the plots to deal with different soil types). Some measurements with proxy-detection sensors are not always performed with sufficient care (not enough leaves measured, not necessarily at the right leaf stage…). Once again, the propagation of uncertainties throughout the treatment chain must be considered.
The difficulty of consolidating adoption figures in the field
What is the real use of digital tools for nitrogen fertilization in the field? This is not an easy question to answer, or at least it depends on what we want to know. One might think that by knocking on the door of the major remote sensing service providers, they could tell us how many hectares their service has been subscribed to (and that would be good enough). Nevertheless, with the free availability of some constellations (notably Sentinel), it is difficult to know if these images are not also used occasionally by farmers, advisory structures or any other actor to propose a nitrogen recommendation outside an identified service. Nevertheless, we could make the hypothesis that this case is not the majority and that the estimate of the hectares controlled would not be too biased if we did not take this case into account. Another difficulty arises when one realizes that the recommendation maps provided by the digital services are not necessarily used for modulation (I refer you to the section on obligations of means and results). A theoretically modulated hectare is therefore likely to be significantly different from a practically modulated hectare (and the modulation is sometimes different from what has been recommended…).
If we now look at proxy-detection tools, the answer is also far from trivial. The quantity of measuring instruments (pedestrian sensors, on-board sensors….) can be relatively easy to obtain by cross-referencing the data provided by manufacturers, machine dealers and/or retailers of all kinds (it is still necessary to be able to identify the most important ones). In terms of usage, however, the answer is highly complicated. On-board sensors are often used by agricultural contractors as part of their services (often because the sensors are expensive to buy alone and the service makes them profitable). We might as well say that we would have to go and see all the agricultural contractors to find out how many hectares are controlled by these on-board sensors. The same goes for pedestrian sensors (clamps and others) which are mainly used by agricultural cooperatives or advisory structures to make recommendations. Besides, the fact that a farmer goes to his cooperative with leaves to be pinched (for a follow-up by a chlorophylian clamp for example) does not bring any information on the quantity of monitored plots and even less on the fact of knowing if the brought recommendation will be really used on the ground (to modulate the contributions or not). In theory, it could be assumed that when advice is generated, it is used – but this assumption would need to be validated in the field. In the same way, the number of downloads of a smartphone application to control fertilization is certainly correlated to the number of real users and hectares controlled, but can hardly help to objectify its concrete use.
To make matters worse, some farmers may use a remote sensing service in addition to a local measurement with a proxy-detection sensor, thus artificially doubling the number of hectares controlled because of the counting of the same area by several different tools.
And the modulation of nitrogen inputs in all this ?
Finally, is the modulation of nitrogen fertilizer inputs justified? One could ask the question, as current agricultural practices in the field, without talking about modulation, still have a significant margin for progress. When we talk about modulation, we often refer to intra-plot modulation, but we could very well imagine that inter-plot modulation (not applying the same thing on each of these plots) is already an advance in itself, especially if the practices follow suit. We should perhaps rather see the intra-plot modulation of nitrogen inputs as the icing on the cake once the agronomic principles of fertilization are well advanced and already used in the field. In view of current events and the increase in fertilizer prices, the cherry on the cake could nevertheless become a cherry cake…
From a theoretical point of view, research seems to show that a large part of the terms of the nitrogen balance equation are spatially variable, even at the intra-plot scale (sometimes at very fine scales): nitrogen residues, fall mineralization rate of organic matter, useful reserves, yield potentials. And these phenomena are understandable because many plots are indeed heterogeneous from a physical point of view (linked to cultural or endemic interventions in the plot) or chemical. We can mention, for example, the settling of headlands, the proximity of hedges and trees, different soil depths or different loads of stones that bring a different capacity to provide nutritional elements, hydromorphic and/or superficial zones within the same plot. All this can theoretically justify the implementation of modulation practices. From a practical point of view, modulation makes sense if the spatial variability of soil properties conditions a variability of the optimal nitrogen supply that is sufficiently important to be taken into account. In addition, this modulation must obviously also be considered in relation to the farmer’s fertilization strategies. For example, modulation will be considered differently if the inputs are organic only, mineral only, or organic supplemented by mineral inputs.
The idea regularly heard behind within-field modulation and its its now well democratized slogan the right input, at the right dose, at the right time and in the right place would be that nitrogen inputs would necessarily be reduced on the plots. Although this is indeed the case under certain conditions, it is not a general truth. The extremely varied results obtained in the scientific literature show that the conclusions depend on the agro-pedo-climatic context in which we place ourselves (nitrogen as a limiting factor or not, amplitude of heterogeneity in the plot, nitrate vulnerable zones or not…). Digital technologies and agri-equipment have of course enabled significant progress in terms of application quality (very precise geo-positioning, section cutting to avoid overlapping and spreading outside the plot, right-left modulation, etc.). Rather than talking about reducing inputs, we should consider a better distribution/spatialization of nitrogen in the plots, which, theoretically, should serve to improve nitrogen use efficiency.
The relevance of modulation is also questioned in view of the complexity of the dynamics of nitrogen flows and stocks in the plots. As we said above, many parameters of the balance method are variable. This is interesting. But it is still necessary to be able to measure or model them at that spatial scale, which some approaches are trying to do. If we add the uncertainties related to their estimation and to the (statistical/mechanical) nitrogen control models in place, would we still be in coherent nitrogen status ranges? The study of the propagation of the uncertainties on the whole of the chains of calculation is here important to consider. From a computational point of view, the dynamic crop models will have to be degraded to more aggregated spatial scales (such as zones) to simplify the calculations. If finally very few zones are retained, intra-plot modulation will also be somewhat degraded (compared to the ability to modulate inputs very finely in space).
Modulation also tends to be considered on a single year basis, and the benefits of modulation – often measured with a return on investment (and not always correctly) – are mainly proposed on this time scale. Does modulation really have a long-term benefit, say over 10 or 20 years, when compared to uniform management over the same period? In view of the temporal variability of environmental conditions, agricultural practices, or even the price of inputs and crop sales (temporal variability that is often much greater than spatial variability), the question remains. It all depends on what we are comparing, since to be completely transparent, we would have to compare practices over the long term on economic, environmental, agronomic and social grounds (time to train, mental load, etc.). But do we really want to do this? Judging modulation on the basis of an annual report is not necessarily appropriate. Modulating nitrogen inputs over a single season may not be useful in some cases, but it could be considered as a long-term trend or average (Kayad et al., 2021), especially since the plots show significant spatial and temporal variability.
The modulation market, which is still relatively small in proportion, nevertheless seems to be following an upward trend, notably driven by machinery players who are emphasizing digital modulation technologies and who are beginning to integrate skills and human resources on these subjects (users of the Farmstar service, for example, seem to have a greater demand for modulation files than before). This increase could be explained by a trend in the renewal of agricultural equipment that is moving in the direction of modulation practices. However, this increase does not hide the fact that farmers are still reluctant to practice modulation, perhaps mainly because they do not know enough about it (many farmers are equipped with modulation equipment – sometimes without knowing it – but very few actually use it to the end).
What should we look out for? A few final points for discussion…
Substantial efforts have been made by public and private players to encourage the use of digital tools for managing nitrogen fertilization. However, their adoption in the field is still rather low.
Digital tools are a step and it cannot be said that these digital approaches have not evolved over time, from very simple vegetation indices at the beginning to more hybrid approaches, more complex modeling and more advanced indices now. The current digital technologies, far from being able to be categorized in the same way, will have made it possible to significantly evolve fertilization practices and improve the efficiency of nitrogen use.
Some approaches are becoming more and more advanced and agronomically sound, but the greater complexity of dynamic tools does not always seem to translate into greater accuracy and better economic and environmental results (Mandrini et al., 2021). By simulating a large number of plot data in the United States, the latter authors showed that, on average, the recommendations of static models at the regional scale did not perform significantly worse than those of dynamic models. The results of the study are not extrapolable as such in the sense that the authors concentrated on a part of the United States, in a sector where the plots, according to them, were rather homogeneous (the dynamic models were more interesting on heterogeneous plots), and for corn crops only. But this article has at least the merit of starting to ask the question. Several of my interviewees were categorical about the interest of dynamic management approaches, in the sense that they would allow for a much more accurate estimation of plant nitrogen requirements, and that the French plots would present sufficiently wide agro-pedo-climatic variability.
Still according to Mandrini et al. (2021), a comparison of tools to determine where dynamic models perform best and why is missing. Some would argue that these models would lack local calibration – and this would be especially important given the repeated discussion of how variable the terms of the nitrogen balance equation are at fine spatial and temporal scales (Colaço et al., 2018). The use of multiple measurement instruments and multi-layers of information could perhaps bring significant improvements. In any case, it will remain difficult to attribute improvements to the adoption of specific technologies, as there are so many avenues of progress in fertilizer management that can be confused with each other.
The deployment of crop models is a major step forward in agronomic terms, but we may nevertheless wonder whether farmers in the field are ready to take this step. These models are indeed complex, and their use in an operational perspective does not seem obvious.
We can also question the ability of our current models to make correct predictions in a changing climate. The uncertainty of climate frequencies is propagated in the models that use them. The climate frequencies have difficulty in capturing the climate risks at the end of the cycle over the last few years. Running models on more recent climate periods (and no longer on long histories) could limit the problems by reducing the inertia of climate variability, which is already being implemented by some numerical reasoning tools. It may also be necessary to weight more strongly the last climatic years. But can we really do much better than that at the moment? We can already be reassured that several approaches incorporate climate forecasts. Climate forecasts are extremely complex and the structures that propose reasoning approaches for nitrogen fertilization are not those that develop climate models.
Although digital technologies have advanced nitrogen fertilization practices overall, some nitrogen management tools focus on delivering a simple solution to a complex problem. Many technologies, for example, focus on only one side of the nitrogen balance equation – plant demand for nitrogen. Admittedly, tracking soil N supply is significantly more complicated (accurate estimation of soil N credit depends on soil and climatic conditions that can be difficult to predict), and is something that is not yet measured intensively and/or operationally with digital tools (and even in the lab, not all forms of N are tracked). But the dynamics of N stock and flux within the soil are so important that it is a shame not to consider them more.
Experimental results with numerical tools may be limited to the conditions encountered during the experiment. They can hardly be generalized with confidence to a wide range of situations different from those observed in the experimental setting, especially if the experiments are carried out over a relatively short time (short-term experiments) or simply if important variables around climate and soil have not been considered (for example when too simple statistical relationships are established between a vegetation index and a nitrogen content or a yield level). Some reasoning tools are nevertheless much more proven than others and will have been developed following a significant number of field trials, and this under diversified agro-pedo-climatic conditions. Note, however, that a large number of sites and years of monitoring does not necessarily mean that a sufficient number of years with sufficient variation in weather conditions or contrast between sites has been considered.
If one wanted to be prickly, one could still argue that these approaches do not, however, challenge the management of nitrogen fertilization as a whole. Approaches based on cover crops, diversified rotations, or conservation tillage are not really considered by these tools, most likely because of the agronomic complexity that this would generate. Work is underway to improve the digital tools in this sense.
Up to now, we have focused on digital tools specifically dedicated to nitrogen fertilization, between the calculation of the forecasted dose at the beginning of the season, its adjustment at the end of the winter, and the control during the season. In Figure 4, I presented in a slightly broader way that other digital tools could be used in some way to improve the management of nitrogen inputs. It is difficult to be exhaustive because, in the end, many tools could also be used – perhaps a little more indirectly – to control nitrogen fertilization. This is the case, for example, of the connected weather stations installed on specific plots of land to manage nitrogen inputs according to the weather and hygrometry (and potentially to prepare for working in increasingly dry spring conditions). There are also tensiometric probes placed in the soil and soil moisture or evapotranspiration models to control water inputs at the same time as nitrogen inputs (and we have seen how the water and nitrogen cycles are interconnected). One could also think of producing soil maps by resistivity or electrical conductivity that can be used to build soil potential maps and be integrated into nitrogen management models (to better characterize production potential and yield objectives). NPK probes exist to monitor the evolution of nitrate content in soils, although these tools are perhaps more used for environmental monitoring than for agronomic nitrogen management.
Between obligation of means and obligation of results
There are many practices for improving nitrogen management – both digital and otherwise. One question to ask is why these practices are being used and whether, for all we know, they could be implemented to reduce a fertilizer use and any environmental footprint. Without seeking here to extrapolate results, Houser (2021), in the context of his American study, would rather say that no. The author shows, for example, that farmers who fraction their inputs the most are also the ones who, on average, tend to increase the amount of nitrogen applied. Houser (2021) attempts an explanation: farmers who fraction may be able to access lower fertilizer prices by avoiding mass purchases of fertilizer at times when it would be much more expensive, and thus take advantage of low fertilizer costs to apply more. The study adds that farm size is positively correlated with increased fertilizer use, which might suggest that having to manage more hectares means that farmers are less likely to bother with fine-tuning their nitrogen fertilization practices. The author may nevertheless be criticized for not having looked at performance indicators (notably the nitrogen use efficiency coefficient) and may add that these results only concern the study area. But these first results have the merit of existing.
On closer examination and in discussion with my interviewees, farmers would mainly use digital nitrogen management solutions to de-cap a regulatory dose (and thus apply more nitrogen than planned). However, one could argue that in dynamic nitrogen management models, the logic of de-capping disappears because it is no longer a question of total dose. When we take a step back and see the money invested in research for decades to increase our agronomic knowledge, to set up references, to launch satellites and to deploy digital technologies, it still makes us feel a bit sick… Some farmers would even divert small, particularly vigorous areas in their plots to be used as a basis for a nitrogen advice on their whole farm (and thus to de-cap the doses over the whole plot). For a farmer, limiting nitrogen on cereals could be akin to a form of castration. The extensive use of nitrogen fertilizer is a form of risk reduction strategy on the part of the farmer to ensure, in his opinion, a minimum yield. This strategy, along with collective action dilemmas (if I am the only one to reduce my doses, why should I do it?) and certain political and economic factors that limit farmers’ choices, favors expanding production rather than using nitrogen efficiently.
In their (the farmers’) defense, it must be said that digital tools seem to be driven by regulations and the nitrate directives. And the tightening of regulations is clearly contributing to the growth of digital technologies. The designers of digital fertilization tools and services are rushing to align the calculation of nitrogen doses with the method recommended by the nitrate directive. However, too much regulation kills regulation, and general rules do not always make sense on a more local scale. Some farmers may indeed want to de-cap a regulatory rate because of the use of an Olympic average yield that does not necessarily represent the potential or the yield objective of the plot. For issues of integrated crop protection, and in particular to improve the tolerance of rapeseed to insect infestations in the fall, one way to improve would be to allow the rapeseed to have continuous growth, for example by allowing a small amount of nitrogen to be applied in the fall in order to avoid a break in growth at the end of the year. This nitrogen application, which would make it possible to unbalance the evolution of insect larvae with respect to the growth of the plant, would make it possible to save an insecticide in the spring. For regulatory reasons (in particular the Nitrates Directive), this is not envisaged. The proportion of legumes is also limited in certain production areas (especially for long cover crops), which may seem contradictory to the desire to work with more resilient systems that would need less external nitrogen fertilizer inputs.
Not all farmers carry out a manure forecasting plan (PPF acronym in French), except in the case of nitrate vulnerable zones where it is mandatory (but is it really done every time?), and the majority of PPFs are outsourced to support structures (CerFrance, cooperatives…). It is therefore reasonable to ask how many farmers really decide on their fertilization strategy themselves. Is this delegation not also a way of removing the farmer’s responsibility for a subject that it would seem logical that he should master from start to finish, given its importance? There are several ways of looking at this. The fact of delegating the service can be seen as a mark of confidence or as the assurance of a follow-up, especially since a good part of the files seem to be renewed from one year to the next. The regulatory dimension around nitrogen can be frightening, with the possibility of regular controls, and the administrative dimension can be particularly heavy. And the regulations are all the more difficult to follow since they are governed by each department at the level of the GREN (regional nitrate expertise group – French acronym). The comfort provided by an outsourced service, in terms of reducing the mental load, is understandable.
Could we go so far as to say that digital tools have become regulatory tools? In any case, this is the first observation that seems to emerge. The digital tools reassure and clear the way if there is a failure (if the tool was sold with the promise of yield gains and these gains are not achieved or the year’s yield is poor, farmers can question the management tool). Farmers pay themselves a sort of validation of their balance dose and do not really try to modulate doses. This obligation of means is a pity because these digital technologies could really be used in an agronomic framework with a will to study the evolution of the dynamics of nitrogen stocks and flows over time to finely control fertilization. One could also imagine that digital technologies are seen as tools to reduce nitrogen leakage (in all its forms) into the environment. Perhaps the introduction of strong political measures (mandatory measures such as quotas, technical requirements, or incentive measures such as eco-schemes or eco-conditionalities) or the implementation of a nitrogen quota exchange market (in the form of what is being set up for carbon) could provide some answers.
Just as the use of digital nitrogen management tools can justify the removal of regulatory nitrogen doses, these digital technologies can also earn valuable points for obtaining the HVE (High Environmental Value- French Acronym) label when used in conjunction with a balance sheet method. The number of points accessible through the use of these tools is not gigantic (normally up to 3 additional points) but it is nevertheless not negligible and these digital technologies could allow some farmers to reach the levels of the label (HVE 1, 2 or 3). Even if the progress approach is commendable, we can nevertheless recall that none of these tools is audited… A working group at COMIFER (French Committee for the Study and Development of Reasoned Fertilization – French Acronym) is currently thinking about a more precise classification of digital tools around the HVE label, but the audit of these tools is not yet a reality. So we are still in a logic of obligations of means. A simple invoice justifying the purchase of one of these tools (sensors, services) should allow us to obtain the points without ensuring that the recommended advice has been followed. Finally, I would like to add that the HVE label is still criticized by many actors in the agricultural sector for its lack of ambition. The opinion of the Environmental Authority on the national strategic plan (NSP) of the next CAP, which emphasizes the use of HVE, is quite severe…
Overall soil fertility: the great loser
A very strong bias towards nitrogen
The tropism in recent years on nitrogen is quite fascinating (Cassman, 2022; Houlton et al., 2019). In the majority imagination, it is indeed nitrogen that is at the heart of efficiency. We have become monomaniacal about it, almost schizophrenic, to the detriment of overall soil fertility. This can be seen in the manure forecasting plans – which are mainly used as tools to control nitrogen – but also in the digital tools that focus on nitrogen recommendations. Bottom dressing, trace elements, all all are still too little considered. All this is perhaps less true in viticulture because the terroir dimension – taking into account the soil – is stronger. Nevertheless, still in viticulture, the percentage of inputs in relation to the turnover is lower than in field crops and the wine growers will not necessarily find the need to control the dose precisely. Margins are often sought elsewhere – for example in the marketing of bottles – rather than in fertilizer management.
Nitrogen is still mainly seen as the limiting factor in agricultural production. However, this is far from always being the case (it is necessary to go back to soil analysis). The agricultural sector uses nitrogen because the effects can be seen (at least some of them, since the phenomena at work in the soil are invisible, which is why the overall fertility of the soil is constantly declining). However, there are still too many mistakes: urea and liquid manure not buried, excessive mineral solution, nitrogen applications on hydromorphic soils, complete nitrogen applications before the 2-node stage on wheat without fractioning. A certain number of fundamentals must be taken into account. Nitrogen alone will not be able to solve the challenges of agricultural production and climatic deregulation to which France is subjected. For 30 years, wheat yields in France have stagnated or even decreased, but the quantities of nitrogen applied have not decreased.
Farmers are not sufficiently trained on this nitrogen subject – those in vulnerable zones will nevertheless be more sensitive to it – and are too content to follow only the regulatory training. As nitrogen fertilization has been almost exclusively considered from the point of view of the Nitrates Directive and green algae, it is not surprising that many farmers have put a regulatory connotation on it. Farmers may have the impression that they have mastered the management of nitrogen fertilizers, but the fact remains that the sector suffers from a major knowledge deficit in relation to the soil and climate (this is obviously not the case for everyone). The knowledge of soil life, rotations, or global fertility are still overused subjects. And, without talking about necessarily agronomic trainings, some trainings are still too much neglected: getting an agronomic advice or adjusting a spreader (it is even more complicated with protected nitrogen formulations) are so many small tests which, cumulated, will end up boring the farmers.
Nitrogen fertilization is however a very complex issue; the dynamics of nitrogen stocks and flows that we have seen in the dossier should have convinced you of this. And management requires extremely solid skills: management over one year or in forecast, static or dynamic, considering the vegetative stages of the crops and the variability of responses according to agro-pedo-climatic conditions (in areas with acid pH, on hydromorphic soil, when there is a phosphorus deficiency, if there is a general fertility problem, etc.), but also according to the objectives of protein in the grain (if the production is under contract). Once again, the first unit of nitrogen brought in is polluting since we are very far from having a 100% use efficiency.
Few farmers carry out manure forecasting plan with a cross-sectional NPK (nitrogen, phosphorus, potassium) approach. The farmer does not see that he is drawing on the soil stock. I would also add that the use of NPK fomulation is pernicious in the sense that one juxtaposes, in the same formula, elements that have little to do with each other (and that do not call upon the same soil compartments). Farmers are used to buying NPK fertilizers as if they had the same origins and function. It is always easier to maintain a correct situation by properly counterbalancing exports. However, when it is time to start making remedial inputs, the nutrient levels in the soil do not recover so quickly and massive inputs are needed (and when you see the price of fertilizers…). The questions are nevertheless starting to come up – but sometimes too late – when farmers are faced with technical impasses (for example when there is no particular accident during the season but the yield obtained is far lower than expected). For those who are reluctant, those for whom nitrogen fertilization does not need to be changed, it will be necessary to give factual arguments, at the risk of seeing these farmers disappear because they will no longer be able to keep up with the pace from a technical and agronomic point of view.
The ecosystem around the farmers is not necessarily better trained, far from it. Some technicians do not have the time (because they already wear many hats) and do not have the appropriate training because they are still too focused on the sale of these nitrogen fertilizers (remember that the separation of sales and advice relates only to plant protection products). It is perhaps by working in tandem between technicians and agricultural advisors – with separate but complementary skills – that the management of nitrogen fertilization will be improved. In terms of digital tools for management, dealers are not equipped to provide training either (and the dealerships are emptying). Farmers’ needs for tools are not identified and still too few technologies are really adapted (apart from the now classic tools such as guide bars).
Proxy-detection tools can be considered as a nice training and animation tool, and even as a user-friendly tool. Several support structures for farmers are equipped with them (cooperatives, traders…). The measurement carried out in group with a crop manager, at the silo, is a meeting point between farmers. It allows them to exchange on the practices in place, to build references and, more generally, to take a step back on the management of nitrogenous fertilization (democratization of practices, fractioning of inputs…). This is often a time when not only the subject of fertilization is discussed and distributors can take advantage of it to discuss other things.
One could also question the independence of nitrogen advice when it is provided by structures that also sell fertilizer. Without necessarily wanting to look for evil everywhere, the use of digital nitrogen management tools – whether or not they are provided as a service – could lead to an increase in nitrogen fertilizer inputs simply because some companies might tend to recommend higher nitrogen rates because they also often sell fertilizer. The separation of sales and consulting is exclusively for crop protection products (although it does not seem that complicated to reorganize or move some subsidiaries to continue to combine the two activities) and it would be appreciated if this separation could also be extended to the management of nitrogen fertilizers.
There will certainly also be a need to slow down and refocus. Agricultural machines have become so precise that we are no longer able to follow in terms of agronomic advice (some crop protection sprayers are able to consider several crop protection products at the same time), so fine are the spatial scales of work becoming. Can we really appreciate the overall fertility of a soil on a scale of a few square meters?
Between organic and mineral fertilizers
The switch to organic fertilization is all the more problematic because its management is more complex than that of mineral fertilizers. The calculation of the forecast dose is complicated because we do not necessarily know how to correctly quantify organic nitrogen inputs, since they vary much more according to soil moisture, temperature, or the nature of the product used (with full control, we could nevertheless say that we can find out what the plant finds in the soil – and in particular the nitrogen in organic products). With organic fertilizers, the link to the water balance must be taken into account. Unlike mineral fertilizers, where the plant simply has to transpire (to recover the nitrates in the soil), organic fertilizers, which are less mobile, will not be so readily available.
What about organic in all this? Some will say that despite everything, organic agriculture remains dependent on synthetic mineral fertilizers – even if they are hidden – because the spreading of liquid manure on organic soils actually comes from cattle that will have eaten feed (or grass) that will have required mineral nitrogen fertilizers to grow. The nitrogen fertilizer will have been transferred from one place to another. This example introduces the difference between what is called a nitrogen input and a nitrogen transfer. It’s really all a matter of scale. On a farm scale, if I apply nitrogen fertilizer, I am making a direct input of fertilizer and my nitrogen balance increases (or at least the input part of my balance equation increases). On the other hand, if I reason at the scale of a much larger system, and if this nitrogenous fertilizer – in the form of liquid manure, for example – comes from a distant plot, I certainly bring nitrogenous fertilizer to my plot, but the distant plot loses nitrogen, since the cattle will have consumed the plants that accumulated the nitrogen, and I will have exported the cattle’s excrement. On a system-wide scale, the nitrogen balance remains unchanged.
If everyone were to go organic and use only organic fertilizers (even though we have seen that in reality this could be hidden mineral nitrogen fertilizers), the number of cattle would have to be increased considerably. At a rate of a few units of nitrogen per ton of manure, if we had to imagine bringing 150 to 200 units (on wheat for example) of nitrogen on the plots, can you imagine the number of cattle we would need to have? Not to mention that in order to reduce the carbon footprint of agriculture, the contradictory assertion is to reduce the size of ruminant herds… The flows of slurry/manure are not transportable over huge distances either. Nevertheless, they are often used close to the place of production (or directly on it for farmers in mixed farming). To come back to the global fertility of the soils, the farmers will also have to be careful with the nutrient exports of their crops (often corn, ryegrass) which can deplete the soil stocks if they are not properly considered.
One might also wonder if grain farmers would be willing to change their way of working and spend time collecting straw, raking manure, storing it, and putting it back on the headland. We have turned the social and economic landscape of farms upside down into large estates, disconnecting agriculture from livestock. We are entering a slope where the decrease in meat consumption is a strong trend, at least for Western countries.
Let’s add that with the current proportion of organic farming in France (which does not deserve a medal), the sector is already facing problems of supply of organic fertilizers, especially because of the many conversions that are starting to come to an end. Some organic pioneers are even starting to think about going back…
Will organic fertilizers be enough to make up for the shortcomings of the plants we currently produce – which have become real production machines? Farmers who really need to maintain yields are turning to organic materials that are close to mineral fertilizers in their assimilation mode and composition (rich in nitrogen and potassium; fertilizers made from bone meal or composted poultry manure…).
In systems with a lot of organic inputs – especially systems with rich plant cover that bring nitrogen to the soil by symbiotic fixation (the presence of weeds like dock or rye grass is a sign of the presence of nitrogen), some farmers wonder how much they could reduce their nitrogen fertilizer inputs. The question is complicated between taking into account mineralization, soil aeration or even wetland management. Remember also that a large part of the nitrogen fixed by the cover crops is captured in the soil in the form of an increase in soil organic matter and therefore not immediately available to the plants. There is always the question of increasing the size of the self-fertilizing flywheel (it is necessary to invest a lot at the beginning for that, always with this organic matter thing) until it is big enough to be able to think of reducing the doses of fertilizers.
On a system-wide scale, nitrogen quantities can also increase as a result of recycling nutrients from the cities to the countryside. If we take a step back, we realize that nitrogen flows are mainly directed from the countryside to the cities. Fertilizer is applied to crops and we ingest nitrogen indirectly through the food we eat. And we excrete nitrogen in our urine and excreta, nitrogen that will return to the agricultural plots via sewage plant sludge spread on the plots. Nevertheless, this process is still largely inefficient and a large part of the nitrogen we excrete is lost in all the treatments in place and finally returns only little to the countryside.
Still and always the climatic deregulation…
The intertwined cycles of nitrogen and carbon
We often fail to see that nutrient cycles – especially nitrogen, carbon and water – are completely interdependent on each other. We still too often mistakenly think of them as mutually exclusive phenomena.
Take water, for example. Water flows drive three antagonistic nitrogen flows that we saw at the beginning of this dossier: nitrogen uptake by plant transpiration, mineralization of organic matter by soil moisture status, and nitrogen losses by leaching and drainage flows. We could also add fertilizer inputs directly during fertigation. Water can and should be considered both as a resource and as a vector. Managing nitrogen fertilization without controlling the water balance of a plot seems relatively complicated. And this strategy will be even more complex in a situation of exclusively organic inputs, since the mineralization of organic matter will have to be much more important.
As for carbon, there are so many examples of interaction with nitrogen that it is difficult to be exhaustive (Figure 5). Carbon and nitrogen are often already stored in the form of organic matter in the soil, and the mineralization of this organic matter generally affects both elements. As discussed in relation to the C/N (Carbon-Nitrogen) ratio, soil microorganisms need to feed on nitrogen in order to break down carbon and/or nitrogen rich organic matter. Nitrogen is also necessary for the production of chlorophyll and thus indirectly for photosynthesis, i.e. a greater availability of nitrogen will increase the photosynthesis of the plant and thus the quantity of organic matter returned to the soil if the plant is not exported. Airborne nitrogen-fixing bacteria, whether symbiotically associated with legume roots or simply present in the soil, are dependent on the availability of organic carbon resources to support their needs. The energy cost of nitrogen fixation represents a significant part of the energy recovered during photosynthesis (for symbiotic bacteria).
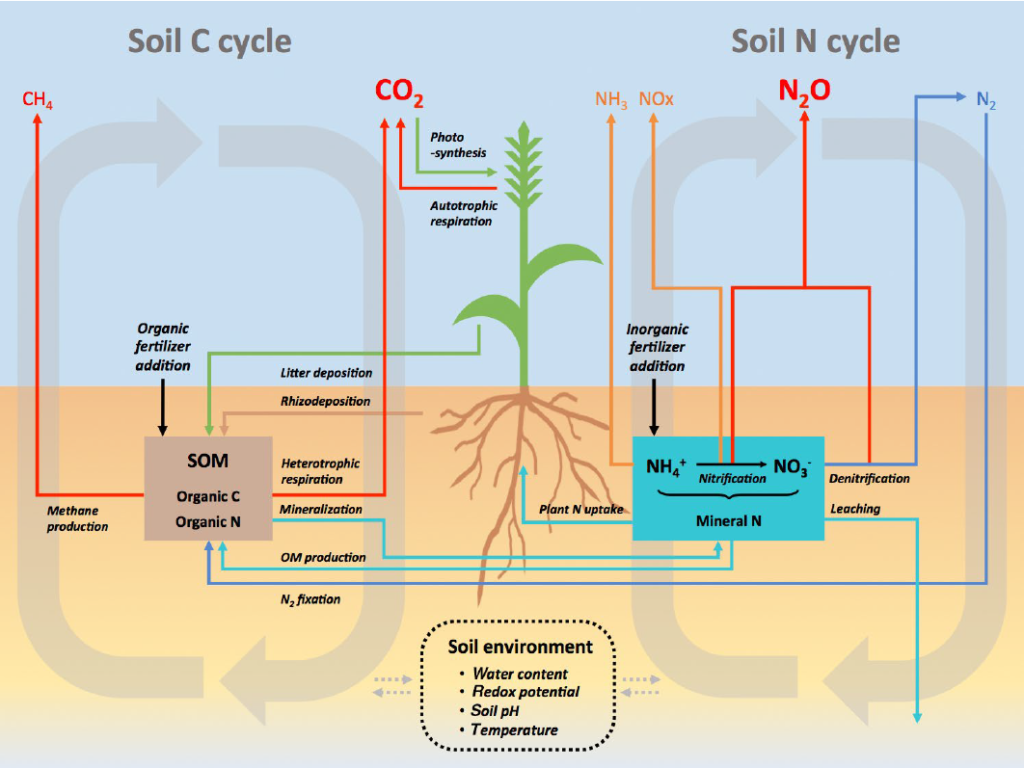
Figure 5. interdependence of nitrogen and carbon fluxes in the plant. Source: Guenet et al. (2021).
These multiple interactions require us to take a step back in managing complex issues, and in particular soil carbon storage in relation to climate deregulation. Stimulating soil organic carbon sequestration can be achieved through soil management strategies. However, we must keep in mind that these strategies can stimulate greenhouse gas emissions (nitrous oxide, methane) and lead to nitrogen losses through leaching. To the extent that a larger biomass – say, of plant cover crops – returns more carbon to the soil, one might be tempted to say that we should not lower nitrogen inputs too much to ensure that these crops grow well and thus return sufficient carbon to the soil. In any case, and we have already talked about this when we mentioned the Carbon-Nitrogen (C/N) ratio, increasing the organic matter rate in soils requires having nitrogen available. To reach a global average Carbon-Nitrogen (C/N) ratio of 15, Guenet et al. (2021) estimated that the 4/1000 initiative (French initiative launched by Stéphane Le Foll), which would allow carbon storage, would require a significant demand for nitrogen inputs (the laws of stoichiometry are impenetrable). However, as we have already discussed, the nitrogen cycle involves emissions of nitrous oxide N2O released during the denitrification stages, a gas with a warming power 300 times that of carbon dioxide CO2. Could the avoided greenhouse gas emissions by storing carbon in soils be completely offset if we are not able to properly account for N2O?
The authors Guenet et al. (2021) are more nuanced but nevertheless add that the effects of carbon storage may be greatly overestimated if N2O emissions are not considered. This is further complicated by the fact that the soil microbial processes that influence N2O emissions are complex, with mechanisms that are often dependent on very fine spatial and temporal variations and on the physical, chemical, and biological properties of the soil; and that our current measurements and modeling do not allow us to properly assess them. Another example of a conclusion that may be counter-intuitive but that deserves to be explored is the fact that reduced tillage, which is widely promoted by the pioneers of conservation agriculture, would also promote the action of denitrifying bacteria and thus the generation of N2O insofar as it would establish anaerobic soil conditions. In addition, because organic matter generally acts as an electron donor in the denitrification process and because the organic carbon content in soils can lower the redox potential of the soil, it is possible to think that soils with a higher organic carbon content can also increase the activity of denitrifying bacteria. Obviously, the prism of reading here is entirely climatic and the subject would require to be dug under different angles (soil fertility…).
Leguminous and companion plants are of course part of the levers for improving nitrogen fertilization (even if a legume does not mean that there will be no release of nitrogen into the environment) in the sense that they will return a certain amount of nitrogen to the soil thanks to the wonders of symbiotic fixation (some will consider returns of the order of a few tens of units of nitrogen – others, much more). One may wonder if these inputs – even if they were much higher – could meet the demands of the plants we currently grow, which are so efficient that they require phenomenal amounts of nutrients. Will the soil really be able to release nitrogen at the rate that plants need it? Will we not be overtaken by genetics?
These cover crops are nonetheless more technical crops (which will therefore require training) if they are to be integrated into a rotation: for example, these crops are sensitive to the fertility of the soil or its moisture conditions (on dry soils, for example), some of them do not necessarily freeze in the winter (and winter conditions are increasingly mild), and they can pose operational limits for questions of site compatibility (especially for companion plants). In the case where the useful reserve is the limiting factor on the plot, legumes could amplify the variability of water availability. In addition, there may not be a market for the plant (if it is exported).
Another proposal could be to change crops, and in particular not to cultivate those with too high a nitrogen requirement. Nevertheless, one of the challenges will remain that we will often fall back on crops that store less carbon. In order to be completely autonomous in nitrogen (we have already talked about self-fertility), we could very well imagine exchanging the concept of green manure for that of a green crop in the sense that the cash crop would be left on the plot – let’s say one year out of four or five – to return nitrogen to the soil (for example, a crushed alfalfa crop). This proposal could respond to the increase in the price of nitrogen fertilizers, but could be confronted with issues of production areas and, not surprisingly, economic questions.
The nitrous oxide issue
It should no longer be a surprise to you, but with regard to nitrogen fertilization, it is indeed nitrous oxide (N2O) that is the source of the greenhouse gas emissions in the nitrogen cycle that we saw at the beginning of the dossier (we will talk again in the next section about the decarbonization of the nitrogen fertilization process as such). N2O emissions would have visibly exceeded the most pessimistic emission scenarios so far. In contrast to natural N2O emissions mainly due to biological N fixation (by legumes or soil bacteria), we learn from Harris et al. (2022) that anthropogenic soil N2O emissions are mainly derived from atmospheric N deposition – not from N fertilization, even though this source is becoming increasingly important. It may be reassuring that COMIFER (French Committee for the Study and Development of Reasoned Fertilization) has revised its volatilization grid to no longer endorse higher rates.
The measurement of nitrous oxide fluxes is also subject to uncertainty because the monitoring methodologies are not completely clear. And nitrogen emission factors could also be largely underestimated because of the dynamic nature of N2O emissions that we are unable to capture correctly with our sparse sampling, but also because emission factors would only be calculated during the plant growth period (whereas we have seen that N2O emissions do not cancel out when plants are not growing). Emission factors are also widely variable between regions of the world (depending on the climate of these regions of course) which would again suggest that aggregated emission factors at too large spatial scales could also be underestimated.
The warming climate could lead to an increase in soil microbial activity with consequent nitrogen release due to the activity of nitrifying bacteria for example. But it is actually very difficult to conclude with certainty about the real implications of an increase in nitrification or denitrification in the soil. Climate disruption will impact the interconnected nitrogen, carbon, and water cycles anyway – and it is perhaps the uncertainty of these impacts that is most concerning. One thing is certain, however, and that is that these implications of climate deregulation on the dynamics of nitrogen stocks and flows will vary widely on a global scale.
Climate deregulation requires us to consider whether the nitrogen supply strategies of the past are still the best today and whether we could secure nitrogen nutrition by changing practices to intervene when nitrogen may be available. The spring drought periods that can be expected are indeed worrisome for a correct valorization of the nitrogen applied. The word “drought” is starting to scare people in the field (for example, many farmers did not apply the third nitrogen application on wheat for fear that it would not rain afterwards). With the current ecological emergency, we can perhaps not be picky about choosing between this or that practice of improving the efficiency of nitrogen use but rather use them together. The majority of digital nitrogen management tools seek to correct an instantaneous state of nitrogen to make up for something that might have been missed before the fertilizer was applied. Few people actually try to correct bad practices and to really study if the nitrogen inputs have been really valorized by the crops or not.
Can we decarbonize nitrogen fertilization?
Nitrogen fertilization is undoubtedly the practice that puts the greatest burden on the carbon footprint of crop production. So far, we have mainly discussed nitrous oxide during the application of nitrogen fertilizers (denitrification, volatilization and denitrification after deposition…). For example, urea – one of the most used nitrogen fertilizers – contains 46% of nitrogen, a large part of which volatilizes into the atmosphere before it is actually used by plants. At the beginning of this dossier, we also mentioned possible actions to improve the efficiency of nitrogen use in fertilizers, including coated fertilizers, bio-inhibitors of nitrification, and non-urea based fertilizers (ammonium nitrate, ammonium sulfate…).
But it is also upstream of the nitrogen fertilization chain, during fertilizer manufacturing, that carbon emissions are important. Ammonia is the basic component of mineral nitrogen fertilizers. It is produced by the Haber-Bosch reaction, which combines hydrogen with nitrogen from the air (this is why it is called hydrogenation of nitrogen from the air). The hydrogen atom not being available in its natural state, it is necessary to recover it in one way or another by breaking certain bonds (water H2O or methane CH4). This hydrogen is thus obtained with energy which can have various origins (coal, natural gas, renewable origin…). The Haber Bosch reaction itself also requires energy (the energy mix of the country of production must be taken into account). The vast majority of the ammonia produced (70%) is used for the production of nitrogen fertilizers (the rest is used for industrial applications: plastics, explosives, synthetic fibers…). Again, energy will be needed for these new chemical reactions and fertilizer assemblies – and here it all depends on the energy mix of the country in which this processing is carried out.
We are talking about low-carbon nitrogen in the sense that it is the production of mineral nitrogen fertilizers that we should decarbonize (I am not talking about organic fertilizers, of course, since the Haber-Bosch reaction does not come into play). Main interest: the origin of hydrogen. Keep in mind that about half of the mineral nitrogen fertilizers are produced from natural gas (which will have to be extracted, generating greenhouse gases – you should not be surprised anymore). The challenge here is to make ammonia as carbon-free as possible. Many players are starting to generate hydrogen from renewable energy sources (solar, wind, hydroelectric, etc.) to feed the Haber-Bosch reaction directly with so-called “green hydrogen”. You may be amused to learn that hydrogen has the chance to have a rather impressive palette of colors depending on its origin: brown hydrogen (produced by coal gasification), blue (produced by steam reformation of natural gas and the carbon emissions from the reaction are recovered by carbon capture and storage technologies), gray (same as blue but there are no capture and storage technologies for the generated carbon), green (produced from renewable energy that usually powers a water electrolysis reaction), turquoise (produced by pyrolysis of natural gas), the list goes on. These new productions – still in the minority – will obviously require considerable industrial investments and a lot of logistics. The literature on the subject is still rather weak but recent works seem to be quite critical on the capacity of green hydrogen to support nitrogen fertilization (at least in Germany as the authors have worked – Ahrens et al., 2022). Nevertheless, the emissions associated with the manufacture of a kilogram of nitrogen fertilizer are reported to be continuously decreasing (Bentrup et al., 2016; Ammonia Technology Roadmap).
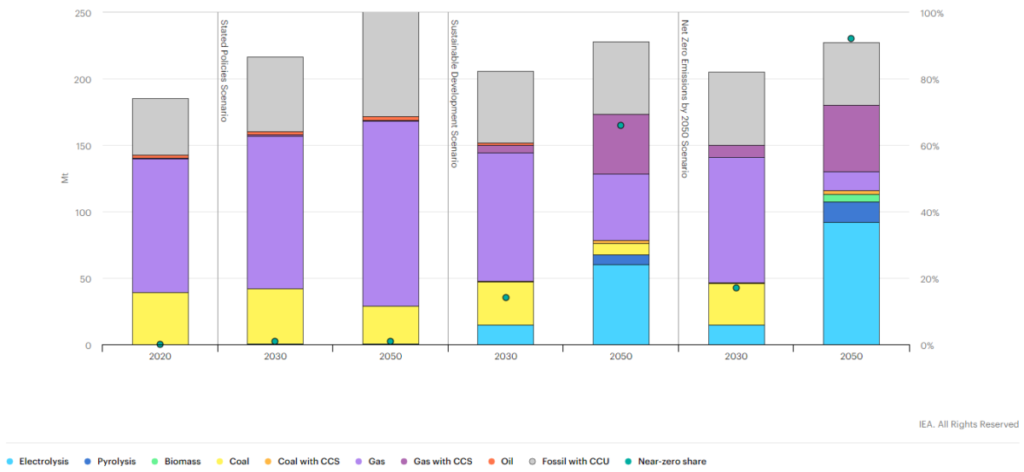
Figure 6. Global ammonia production by technology and scenario, 2020-2050. Source: IEA
Some would even talk about recovering the nitrogen in fossil fuels for use in nitrogen fertilizer – I’ll let you dig into that!
Work is also underway to consider ammonia as a hydrogen carrier because it can be more easily stored as a lightly pressurized liquid and has a relatively high volumetric energy density despite the energy required to synthesize ammonia from nitrogen and hydrogen. The transport of hydrogen alone suffers from operational and logistical constraints (dry loss due to gas leaks because the atom is very small; flammability of hydrogen…). Once it arrives at its destination, ammonia can be decomposed into hydrogen or used directly as a fuel (this is why the demand for hydrogen to replace marine fuel is expected to rise sharply: https://www.iea.org/reports/ammonia-technology-roadmap/executive-summary)
More expensive and less available nitrogen fertilizers
The price of nitrogen fertilizers has risen, it is a reality. Against the backdrop of the war in Ukraine and the lack of Russian gas supplies, the cost of mineral nitrogen fertilizer, made from gas (we have already talked about this), has increased significantly. And the geopolitical stakes are enormous. In the summer of 2022, the European Commission has asked European countries to reduce their gas consumption by 15% (mainly by focusing on industry). France is seeking to diversify its gas supply sources, in particular by bringing in American shale gas. France has just launched a major project to build a port in Le Havre to accommodate liquefied natural gas tankers – particularly American ones – that transport liquefied natural gas (we agree that this is shale gas obtained by hydraulic fracturing).
But the crops have also been valued upwards. We could of course expect a scissor effect in the future (the fact that the cost of nitrogen goes up but not the cost of crops) but that is not what we are seeing today. In fact, it is quite surprising that the industry seems to be focused on driving down the price of nitrogen inputs. Inputs are seen as an expense and a direct loss, while the crop is seen as a profit – but the two seem to belong on a different balance sheet. The psychological bar of fertilizer price (like fuel price at 2€) is sometimes just too high. With nitrogen delivered to the yard at less than 1€ per kilo so far, this is somewhat understandable. In any case, it is certain that the economic optimum of agricultural production is likely to change with the evolution of fertilizer and crop costs. Perhaps current events will push the industry to consolidate the manure forecasting plans and to see them as real working tools for the management of nitrogen fertilization.
Perhaps the problem is not so much the price as the supply. Some farmers no longer even look at the purchase price of these nitrogenous fertilizers to be sure of having enough for the coming seasons. Fertilizers follow terrible speculation curves and the ships carrying these fertilizers are bought back several times between the moments when they leave their port of departure and arrive at their port of arrival – the intermediary of course applying a small margin and his surplus value. Distribution to the last mile also involves huge logistics costs. One could also worry that with the drought, water levels on ship passages are lowering, requiring ships to be less loaded and thereby increasing the transport price per kilo of fertilizer transported. In view of the supply difficulties at the end of the winter, there is a risk that farmers will be told that they simply cannot be delivered.
The greenhouse gas reduction targets to which France is committed would have most certainly had an impact on the cost of nitrogen fertilizers anyway. The war in Ukraine may have simply accelerated a phenomenon that would have happened anyway.
Human health and food waste
The analysis of exported wheat shows that it is rich in nitrogen. In terms of public health, the question to ask is whether this form of nitrogen is interesting for animals and humans. I am of course referring to the phenomenon of gluten intolerance. The pressure of industrial processes, with standardized wheat (with so many percent of proteins) imposes to cultivate wheat very rich in gluten so that the dough of the baker is very elastic and that he can make the same bread all year long. Since wheat gluten is not digestible, is there really any point in producing food that is not digestible and not very valuable?
To conclude, let’s mention the stakes of food waste which – to make the link with our theme – can be simply reduced to a dry loss of nitrogen since the nitrogen used to produce these foods is not valorized. Diets with a low carbon footprint are also often diets with a lower nitrogen footprint; simply because if we eat a plant directly rather than an animal that has eaten that plant; there will be less nitrogen loss in the transformation process. However, I will stop here on this food topic as the debates on food are numerous (replacement of animal proteins by insect proteins, societal acceptance of new diets, colonialism of low meat diets in developing countries…)
In conclusion
The nitrogen cycle remains extraordinarily complex and the dynamics of nitrogen flow and stock are still poorly understood (the nitrogen provided by mineral nitrogen fertilizers is not used in its entirety by the plant in the year of their application), as the processes underway vary at fine spatial and temporal scales.
Nevertheless, it must be emphasized that digital technologies have significantly advanced the reasoning behind nitrogen fertilization. Measurement tools and approaches that were very simplified at the beginning have given way to more mature and operational technologies and to more and more accomplished agronomic considerations (we are thinking, for example, of integral control). Digital tools have directly or indirectly helped to improve the apparent nitrogen use efficiency (NUE), even though it will always be difficult to determine the exact contribution of digital tools, given the diversity of methods and practices for reasoning nitrogen fertilization. Substantial efforts have been made and, even if this dossier sometimes points out the limits of digital technologies (I invite you to read the entire dossier), it cannot be denied that France has made great progress in the area of nitrogen fertilization reasoning. The nitrogen use coefficient is certainly of the order of about fifty percent, but everyone will be able to see the glass as half empty or half full.
Digital tools for reasoning nitrogen fertilization are extremely numerous and their diversity actually underlies quite different approaches and functioning. The main infographic in the dossier, which separates the tools for calculating the forecasted dose at the beginning of the season, the tools for adjusting the dose at the end of the winter, the tools for managing nitrogen during the season, and the tools related to managing nitrogen fertilization, should help to clarify matters. Nevertheless, it is important to continue to use this classification in order to further discriminate between the approaches of these digital technologies. To make the topic accessible to the greatest number of people, the digital ecosystem in agriculture must be simplified and presented with an agronomic reading.
The vast majority of digital tools have been designed to improve fertilizer efficiency, i.e., to enable farmers to achieve the highest possible productivity with the least amount of nitrogen. They are therefore calibrated to achieve a yield that maximizes the relationship between profitability and the efficiency of the nitrogen applied (or a maximum commercial yield that takes into account yield and crop quality), but they have not been designed to achieve a minimal environmental impact (any unit of nitrogen necessarily pollutes, even if the first doses of nitrogen are often much more efficient than the following ones). Consideration of environmental impacts is not yet part of the equation as these impacts have not been quantified economically. In view of the ecological emergency we are facing and the fact that new planetary limits are regularly exceeded (the planetary limit linked to the deregulation of the nitrogen cycle has been largely exceeded), it is time to be concerned.
Could we imagine that the definition of fertility is not simply the moment when the nitrogen dynamics within the soil-plant system are so well understood that fertilization can be dispensed with? Digital tools, as instruments for measuring, describing and modelling the phenomena involved, could play a part in this….
Soutenez Agriculture et numérique – Blog Aspexit sur TipeeeBibliography complementary to the interviews
Ahrens, F. et al. (2022). Decarbonization of Nitrogen Fertilizer: A Transition Engineering Desk Study for Agriculture in Germany. Sustainability, 14
Bramley, R.G.V., & Ouzman, J. (2018). Farmer attitudes to the use of sensors and automation in fertilizer decision‑making: nitrogen fertilization in the Australian grains sector. Precision Agriculture
Brentrup, F. & Hoxha, A. (2016). Carbon footprint analysis of mineral fertilizer production in Europe and other world regions. Conference: The 10th International Conference on Life Cycle Assessment of Food
Cassman, K.G. & Dobermann, A. (2022). Nitrogen and the future of agriculture: 20 years on. Ambio, 51, 17-24
Colaço, A.F. et al. (2021). How will the next-generation of sensor-based decision systems look in the context of intelligent agriculture? A case-study. Field Crops Research, 270
Colaco, A.F. & Bramley, R.G.V. (2018). Do crop sensors promote improved nitrogen management in grain crops? Field Crops Research, 126-140
Comer, B.M. et al. (2019). Prospects and Challenges for Solar Fertilizers. Joule, 3, 1578-1605
Davies, C.A, et al. (2021). The importance of nitrogen for net carbon sequestration when considering natural climate solutions. Global Change Biology, 27: 218-219
Diacono, M., et al. (2012). Precision nitrogen management of wheat. A review. Agronomy for Sustainable Development
Fixen, P.E., & west, F.B. (2002). Nitrogen Fertilizers: Meeting Contemporary Challenges. Ambio, 31, 169-176
Gao, Y. et al. (2022). A critical review of biochar‑based nitrogen fertilizers and their effects on crop production and the environment. Biochar, 4:36
Guenet, B. et al. (2021). Can N2O emissions offset the benefits from soil organic carbon storage? Global Change Biology, 27: 237-256
Harris, E., et al. (2022). Warming and redistribution of nitrogen inputs drive an increase in terrestrial nitrous oxide emission factor. Nature communications, 13
Houlton, B.Z., et al. (2019). A World of Cobenefits: Solving the Global Nitrogen Challenge. Earth’s Future, 7, 865-872
Houser, M. (2021). Does adopting a nitrogen best management practice reduce nitrogen fertilizer rates? Agriculture and Human Values, 39, 79-84
Guerrero, A., et al. (2021). Current sensor technologies for in situ and on-line measurement of soil nitrogen for variable rate fertilization: A review. Advances in agronomy
Kayad, A. (2021). Ten years of corn yield dynamics at field scale under digital agriculture solutions: A case study from North Italy. Computers and Electronics in Agriculture, 185.
Ladha, J.K., et al. (2020). Achieving the sustainable development goals in agriculture: The crucial role of nitrogen in cereal-based systems. Advances in agronomy
Lassaletta, L. et al. (2014). 50 year trends in nitrogen use efficiency of world cropping systems: the relationship between yield and nitrogen input to cropland. Environmental Research Letters, 14
Li, T., et al. (2018). Enhanced-efficiency fertilizers are not a panacea for resolving the nitrogen problem. Global Change Biology
Mandrini, G. et al. (2021). Understanding differences between static and dynamic nitrogen fertilizer tools using simulation modelling. Agricultural Systems, 194
Mandrini, G., et al. (2021) Exploring Trade-Offs Between Profit, Yield, and the Environmental Footprint of Potential Nitrogen Fertilizer Regulations in the US Midwest. Frontiers in Plant Science, 13
Martinez-Dalmau, J. et al. (2022). Nitrogen Fertilization. A Review of the Risks Associated with the Inefficiency of Its Use and Policy Responses. Sustainability, 13
Ministère de l’agriculture, de l’agro-alimentaire et de la forêt (2016). Recensement et analyse des outils de raisonnement dynamique et de pilotage de la fertilisation azotée. Rapport, 81p
Munoz-Huerta, R.F. et al. (2013). A Review of Methods for Sensing the Nitrogen Status in Plants: Advantages, Disadvantages and Recent Advances. Sensors, 13.
Omara, P. (2019). World Cereal Nitrogen Use Efficiency Trends: Review and Current Knowledge. Agrosystems, Geosciences & Environment
Pasquel, D., et al. (2022). A review of methods to evaluate crop model performance at multiple and changing spatial scales. Precision Agriculture
Thomas, F. (2022). Circulation de l’azote apporté dans les systèmes agricoles l’azote intègre pour un tiers les matières organiques. Agronomie, écologie et innovation. TCS n°118. D’après la publication de Sebilo et al. (2013) Long-term fate of nitrate fertilizer in agricultural soils, publié dans Proceedings of the National Academy of Sciences
Samborski, S. et al. (2009). Strategies to Make Use of Plant Sensors-Based Diagnostic Information for Nitrogen Recommendations. Agronomy Journal, 101
Padilla, F.M., et al. (2018). Proximal Optical Sensors for Nitrogen Management of Vegetable Crops: A Review. Sensors, 18.
Verdenal, T. et al. (2021). Understanding and managing nitrogen nutrition in grapevine: a review. Oeno One, 1, 1-43
Wu, S. et al. (2022). Energy Decarbonization via Green H2 or NH3? ACS Energy Letters, 7, 1021-1033
Yan, M. (2019). Rethinking sources of nitrogen to cereal crops. Global Change Biology, 26:191-199
Interviewees
Name | Structure |
Pierre ALOUIS | Vantage |
Timothée BARBIER | Wanaka |
Cédric BOUDES et Rémi Delcaillau | Yara |
Marie Aure BOURGEON | CNH |
Benoit CALMES | UAPL |
Edith CAUMES | Arterris |
Jérôme CIPEL | Fritzmeier |
Jérôme CLAIR | BASF |
Anthony CLENET | ICV |
Chloé COCHAIS | Earth Daily Agro |
Thibaut DEPLANCHE | Celestalab |
Jérôme DIAZ | Konica Minolta |
Rémi DUMERY | Agriculteur |
Grégoire DUPRE | Abelio |
Romain FAROUX | Consultant indépendant |
Clément FRAIGNEAU | Permagro |
Sylvain HYPOLITE | Agrod’Oc |
Hélène LAGILLE et Thomas LOMBERTY | Vivescia |
Clément LESTAGE | Maïsadour |
Aurélie METAY | INRAE – Institut Agro Montpellier |
Vincent MIGAULT | ITK |
Daniel PASQUEL | Institut Agro Montpellier |
Thomas SALLEMBIAN | Trimble Agriculture |
François TAMBOISE | Agriculteur |
Alexandre WEIL | Precifield |
1 thought on “The reasoning of nitrogen fertilization by digital tools: a rather fragile love affair”